Lyme disease is the most prevalent vector-borne disease in the temperate Northern Hemisphere. Spread by deer and sheep ticks, it affects over 1000 people each year in the UK and over 300,000 in the USA.
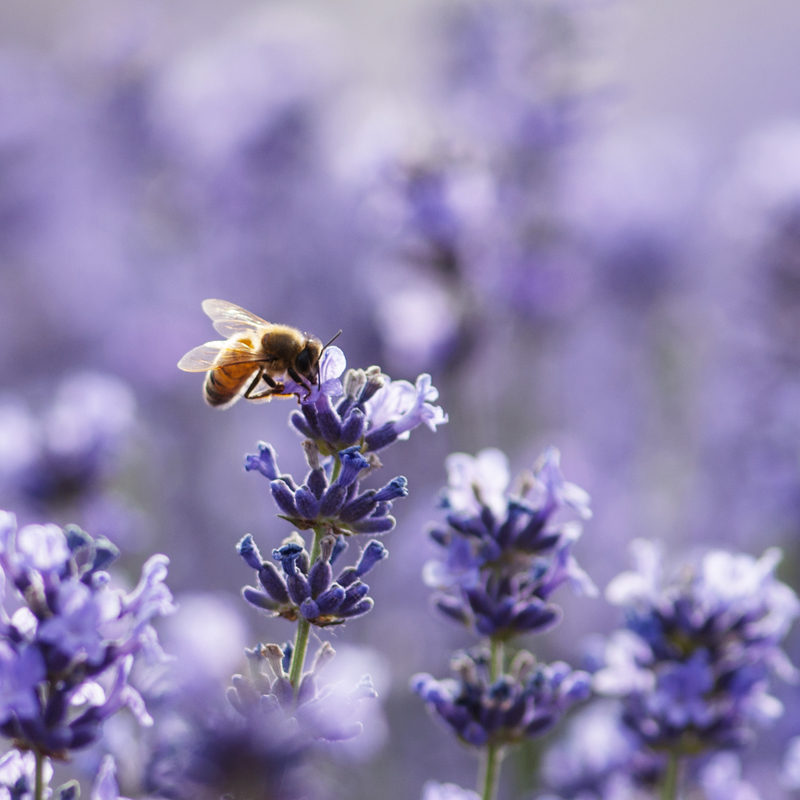
Where did Lyme disease come from?
Although the disease is named after the towns of Lyme and Old Lyme in Connecticut, where the first human case was reported in 1975, it is not a new disease; the bacteria responsible originated in Europe before the Ice Age and symptoms were officially described in 1883 in Germany.
Why is it important to track and model Lyme disease in this way? And, why specifically in Scotland?
A mechanistic model is a useful tool to explain the complex ecosystem of Lyme disease. It can be applied to detect unobserved disease risk patterns, test scenarios for potential future changes, and assess potential controlling strategies. There’s no vaccine for Lyme disease so its prevention relies on improving our understanding of how it is transmitted and using all the knowledge and data available to predict the patterns of disease risk in changing environments.
Scotland is where the disease exposure is potentially high, owing to a substantial public participation in nature-based activities. Scotland actually has much lower prevalence/incidence than most of Europe, but environmental and tick abundance data are available for Scotland. It’s also towards the northern range of Lyme disease with a previously low incidence, and that incidence has increased rapidly over the last 20 years, making Scotland a particularly interesting place to predict risk due to environmental changes.
What does your model tell us about Lyme disease?
The risk of Lyme disease is strongly related to the number of infected nymphal ticks, which can be influenced by a wide range of biophysical factors related to host communities, climate and landscape. Temperature has been shown to influence the transmission dynamics of Lyme disease by changing the behaviour, development, fertility and survival of ticks and their host suitability and population.
Our model integrates spatial heterogeneity and a number of temperature-dependent functions to assess tick population ecology, host movements and pathogen transmission over time. We predicted that, over a 12 year period, the risk of Lyme disease peaks in autumn, approximately 6 weeks after a temperature peak, and that this risk decreases with increasing altitude. Temperature increases were predicted to prolong the duration of the tick questing season – when they seek their hosts – and expand the risk area further north and to higher altitudes.
So does climate change mean a greater risk of Lyme disease?
The model predicts that a warmer climate could prolong and expand the tick-infested area. These predicated impacts on tick population ecology may lead to greater tick-host contacts under climate warming, increasing the risk of pathogen transmission and the chance of people becoming infected with the disease. In this study, however, disease risk is based solely on the density of infected nymphal ticks, but a high biophysical risk does not always indicate a high human infection rate. Socio-economic factors shaping the pattern of human-risk outdoor activities, such as walking, orienteering and gardening, are likely to highly influence the disease pattern, and many of these activities are also likely to be influenced by climate change too.
Are there things we still do not understand about Lyme disease?
Yes, many. The transmission system involves multiple vector life stages, pathogen genospecies, host types and movement patterns. The potentially important mechanisms underpinning the interactions of these system components are numerous and complex. Unfortunately, there is insufficient knowledge and field evidence for their full quantification within our modelling framework; we still need more detailed research on the role of deer densities relative to the densities of other host types to aid with environmental management of risk.
How will you develop the model for future studies, or how might it be applied managing the disease?
Firstly, we want to move to the next-level of disease risk prediction – the pathogen spill-over risk at the human-tick interface. This will be integrated with a socio-demographical model of outdoor human activity frequency to estimate the contact possibility between humans and ticks. And secondly, we want to apply this model with more plausible scenarios of future climate and socio-economic change. Scenario development exercises with stakeholder involvement are being undertaken in our EU-funded IMPRESSIONS project.
‘Modelling the seasonality of Lyme disease risk and the potential impacts of a warming climate within the heterogeneous landscapes of Scotland’ is available to read online now.