Machine learning – the form of narrow artificial intelligence which allows machines to learn from data – has enormous potential to transform urban life.
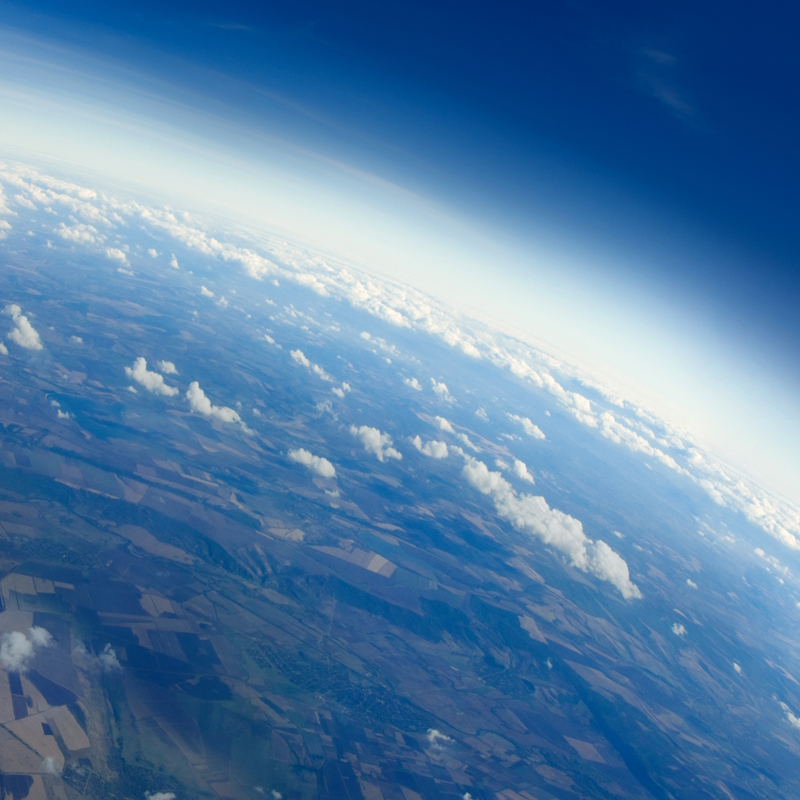
Therefore, as part of our wider project on machine learning, the Royal Society led a workshop on machine learning for smart cities, transport and utilities on 29 September. We explored the ‘art of the possible’ and discussed barriers to the safe and rapid implementation of machine learning in everyday life.
This blog post provides a brief overview of the discussion.
What would transformation look like?
Transport: Intelligent transport systems could help ease congestion, reduce pollution, and improve customer experiences on public transport. In one vision of the future, transport options could be intelligent; smart phones could review a range of travel options and make personalised suggestions, using machine learning to account for personal preferences such as lifestyle choices. Machine learning also supports driverless cars, as we’ve discussed before, which could have a range of benefits. For example, Elon Musk has suggested that humans could be banned from driving due to the enhanced safety benefits of driverless cars.
Utilities: Machine learning is already being used in the energy sector to optimise power grids and could support decarbonisation by providing utilities more intelligently. For example, the Nest Learning Thermostat uses machine learning to learn a homeowner’s preferences and schedules, optimising home heating and cooling. This ‘home intelligence’ may also spur changes in utility business models, meaning that users could pay for services such as mobility, comfort and cleanliness rather than products like electricity, gas and water.
Smart cities: Cities are melting pots of many complex and inter-dependent problems. Machine learning could solve these connected issues by optimising city planning and integrating city services for personalised outcomes, even if individuals have not used particular services before. For example, machine learning could track waste levels in recycling bins and tailor collection services based on waste predictions. This could also provide insights into variable recycling rates.
Hurdles to overcome
The availability, quality and format of data are central to enabling the use of using machine learning. Yet our workshop identified data accessibility issues as a key barrier for using machine learning in the context of smart cities, utilities and transport. There are different ways of addressing this issue, and Manchester’s support of public data sharing as part of its recent urban devolution shows one potential way forward.
That said, data alone is not enough. ‘Big data doesn’t mean big knowledge’: public bodies and private companies need to formulate useful questions to interrogate data with, in order to generate the desired outcome, such as more bus journeys at lower cost and less carbon, for example. Generating these questions requires domain experts and data scientists to work together, but these roles are often disconnected. A form of ‘data science for non-data scientists’ training could help increase understanding of when machine learning can be valuable, and create intelligent customers for machine learning systems.
Some machine learning systems are able to produce highly accurate results, but suffer from a lack of transparency in how these results were created, as we’ve noted on this blog before. The low interpretability of such systems can create issues with verification or certification, and increasing the time taken to secure regulatory approval could discourage innovative services or products. Yet this also has to be balanced against protecting customers from risky innovations.
What next?
There is a strong need to ‘join up the dots’ between technologies, such as machine learning and the Internet of Things, to create truly smart environments. This could be achieved in part through greater awareness of the potential of machine learning – through case studies, for example – and by breaking down siloes between sectors. For example Greenwich’s driverless cars project is drawing from new thinking in energy, transport and smart cities.
Ultimately, any vision for the future of utilities, transport and smart cities needs to be consumer-led, as public buy-in will inform ethical and acceptability considerations as machine learning is applied in everyday life. The first step is to identify consumer needs and ‘proof of concept’ examples for machine learning to increase interest and safe uptake by private and public services alike. Machine learning is currently most widespread in marketing such as Amazon’s recommender systems and Netflix, but used properly, machine learning could be harnessed to create transformational change for the public good.
The Royal Society is currently carrying out a project looking at the potential of machine learning over the next 5-10 years, and barriers to realising that potential. As part of this project, overseen by an expert Working Group, we’ve been holding public events and industry roundtables, including the pharmaceutical sector, manufacturing and the professions. For further information, take a look at our website.