As regular readers will know, the Royal Society is currently carrying out a major policy project on machine learning.
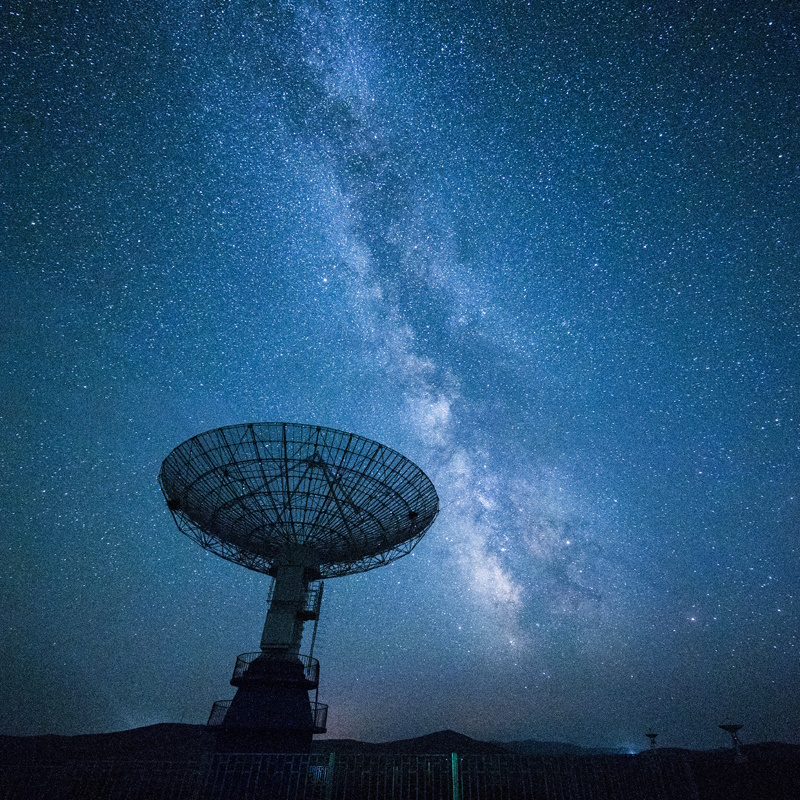
As regular readers will know, the Royal Society is currently carrying out a major policy project on machine learning.
Our previous blog posts on the subject have explored some of the questions the public have about machine learning and artificial intelligence, how these technologies can be used in different sectors, and some of the technical challenges associated with its use.
From 5 to 10 December, the Neural Information Processing Systems (NIPS) Conference 2016 brought together members of the machine learning community from across the globe to discuss current areas of interest and potential future directions. The conference is one of the main events in the machine learning calendar, so what can discussions at the conference tell us about the state of machine learning today?
Signs of growing interest
The first NIPS conference took place in 1987, and was attended by 600 people working in machine learning.
Since then, machine learning systems have beaten humans at chess, the US quiz show Jeopardy, and, most recently the ancient Chinese game of Go; they have helped create applications many of us use every day, such as virtual personal assistants; and trials of driverless vehicles, which use machine learning to analyse the world around them, have started across the globe.
So it’s not surprising that the conference has grown in size over its 30 year history, but the magnitude and pattern of this growth is something else. This year’s NIPS conference brought together almost 6000 attendees, making it ten times bigger than at its inception and twice the size it was only two years ago.
Deep learning is a hot topic, but it’s not the whole story
Deep learning – a particularly advanced area of machine learning – has underpinned many of the recent high-profile advances in the field, catalysing advances in language processing, robotics, and computer vision. The broader attention given to this area of work is reflected in the submissions to NIPS, with deep learning being the most popular subject area for papers and talks.
Yet, while deep learning remains a hot topic, it’s not the only game in town. Other fields also attracting significant interest at the conference, and being frequently referenced in conference activity, included computer vision, large scale learning, and learning theory, amongst others. Also notable was the growing interest amongst attendees and those running workshops in areas of research which relate to social or governance challenges in machine learning, such as interpretability, privacy and fairness.
Machine learning is feeding from and into other areas
As you would expect, the conference showcased many papers and presentations describing technical achievements in machine learning. While impressive in and of themselves, equally impressive was the range of applications to which these advances were being applied. These applications included, for example:
- Supporting businesses by giving more accurate forecasts of how much demand there will be for products or services provided by online retailers, and using these insights to inform pricing strategies
- Analysing the energy consumption of different home appliances, and using these insights to improve energy efficiency
- Underpinning scientific advances, by helping researchers make sense of the complex data generated by experiments such as those at CERN
- Improving the accuracy of medical diagnoses, for example by analysing images from brain scans, or by the link between the composition of microbial communities that live on or in the human body and the risk of pre-term birth
In addition to feeding into a broad range of subject areas, researchers are also thinking about what machine learning could learn from other subjects. For example, if advanced AI systems will be increasingly operating in complex and interactive environments, are there lessons we can draw from ecology – and the understanding it gives us about the natural environment and how organisms interact with this – to improve their design? Being able to simulate the natural environment could provide a more effective testing environment for AI.
The demand for machine learners
We’ve blogged previously about the demand for highly-trained data scientists. This “bloody war for talent” means that people with machine learning skills are in high demand in a range of industry sectors and in academia. With such a large number of machine learning specialists in one place, it is perhaps not surprising that companies looking to expand their machine learning expertise followed, and a large number of companies, both within and outside the tech sector, were looking for talent.
The Royal Society at NIPS2016
Finally, what did the Royal Society bring to this event? Our workshop on 9 December explored some of the preliminary results of our survey into public attitudes to machine learning, and considered how a new wave of research in machine learning could both push forward the field while also addressing key ethical or governance challenges associated with its use. We’ll write more about the workshop on this blog soon, and we’ll be publishing the results of our public dialogues in 2017.