Machine learning has been the subject of much attention in recent years, as progress in the field has opened up opportunities across a range of industry sectors.
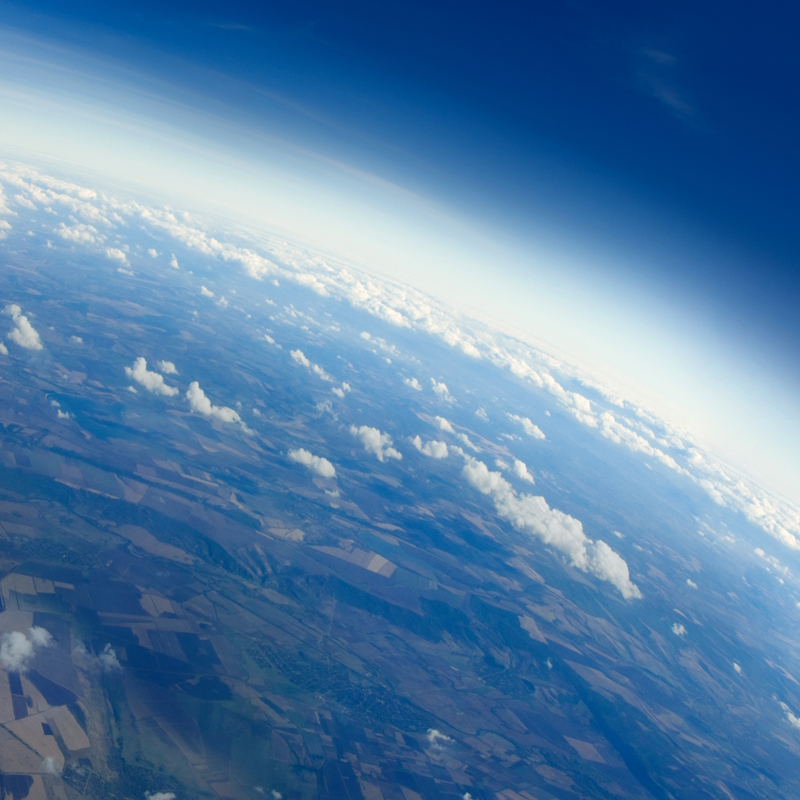
Machine learning has been the subject of much attention in recent years, as progress in the field has opened up opportunities across a range of industry sectors, and supported a new wave of artificial intelligence-based applications.
On 31 January and 1 February 2017, the Raymond and Beverly Sackler Forum brought together experts in machine learning at the US National Academy of Sciences to consider the frontiers of machine learning, its place in society, and activity in the research and commercial communities.
This post gives a flavour of some of the topics that were discussed at the meeting, before a full report of proceedings is published later this year. If you want to find out more about what was discussed at the Forum, videos have now been posted online, and you can view them via the Forum’s website.
The frontiers of machine learning: “the road to building intelligence is paved with dumb stones”
Machine learning systems already underpin a range of applications we use every day, from making Google searches more accurate, to helping virtual personal assistants on our smartphones understand our queries, and allowing ATMs to recognise handwriting on deposited cheques. Zoubin Ghahramani FRS outlined a number of key areas – or canonical problems – that machine learning can now be used to address, including classifying data into different categories, clustering similar data points together, and regression analysis . Using these techniques, machine learning systems are able to perform certain tasks – such as image recognition, voice recognition, or machine translation – to a high standard.
Yet, as Vint Cerf ForMemRS explained, while these capabilities may be deep, they are also narrow. And the ability to learn to perform narrow tasks to a high standard, and to a better standard than humans in some cases, is not the same as achieving general intelligence or human-style reasoning. Rather than seeking to mimic human intelligence, these are systems which augment human abilities, allowing us to accomplish tasks to a standard that we could not otherwise achieve.
And in many cases, as Greg Corrado, Google, noted, the applications of machine learning that we see today are not things we would naturally think of as being ‘intelligent’. Artificial intelligence (AI) is a broader field than machine learning; while AI seeks to make machines intelligent, machine learning focuses on the learning component. This is necessary to achieve intelligence – a stepping stone – but is not all that is required.
Machine learning in society: “as citizens, where do we want to deploy algorithms?”
From scoring credit to advising on criminal sentencing, machine learning is increasingly being used in systems which may have significant personal or societal effects.
Pam Dixon, World Privacy Forum, explained that there are already various regulatory mechanisms that – in different ways – contribute to governing the use of data and machine learning:
- The US Fair Credit Reporting Act requires companies to provide an explanation about which factors have negatively affected your credit score;
- The US Equal Credit Opportunity Act prohibits the use of certain personal characteristics in determining whether or not an applicant is granted credit.
- The EU General Data Protection Regulation restricts the use of certain categories of sensitive data.
Beyond these existing regulatory measures, ensuring continued public confidence in machine learning systems requires deliberation about what Karen Yeung, King’s College London, described as the “constitutional values” – such as transparency, accountability, privacy, and equality – which could be influenced by increased use of this technology.
Machine learning in the research and commercial communities: “what is your business model?”
There is huge further potential in the field, both in terms of technical advances, and new applications, often in tandem.
For example, in the field of healthcare, machine learning could help both understand the genetic basis of disease and design new treatments. Large-scale studies into genetic variation in humans have already provided insights into the genetic basis of some complex diseases. Gaining deeper insights into the genetic basis of disease, and what this means for how our cells and bodies function, requires combining this genetic data with further information about the impact of genetic changes on our physiology. Analysing this data in an integrated way could yield new insights into the causes of disease, and create new opportunities to design treatments, and Gil McVean FRS explained how Genomics plc is working to address this.
In transport, as Raquel Urtasun, University of Toronto, and Jeff Schneider, CMU and Uber, explained, autonomous vehicles could help reduce the number of accidents on the roads, ease congestion, and reduce the environmental impact of driving by making more efficient use of our transport systems. Self-driving cars could also make transport accessible for people who can’t currently access it. These vehicles draw from machine learning to guide their interactions with the environment. For example, image recognition systems using machine learning can help vehicles navigate the roads.
And there are further opportunities across healthcare, finance, and commerce, in addition to a range of exciting research challenges for the field.
This blog gives a taste of some of the issues discussed at the Sackler Forum on the frontiers of machine learning. A full report of proceedings at the Forum will be published later this year. In the meantime, you can see videos from each of the talks at the Sackler Forum website.