The theme for this year's Peer Review Week is "Peer Review and the Future of Publishing". We speak with Denisse Albornoz, Science Policy adviser in the Royal Society’s Data team, about the policies that will likely evolve as AI technology continues to advance and its impact on scholarly publishing becomes more pronounced.
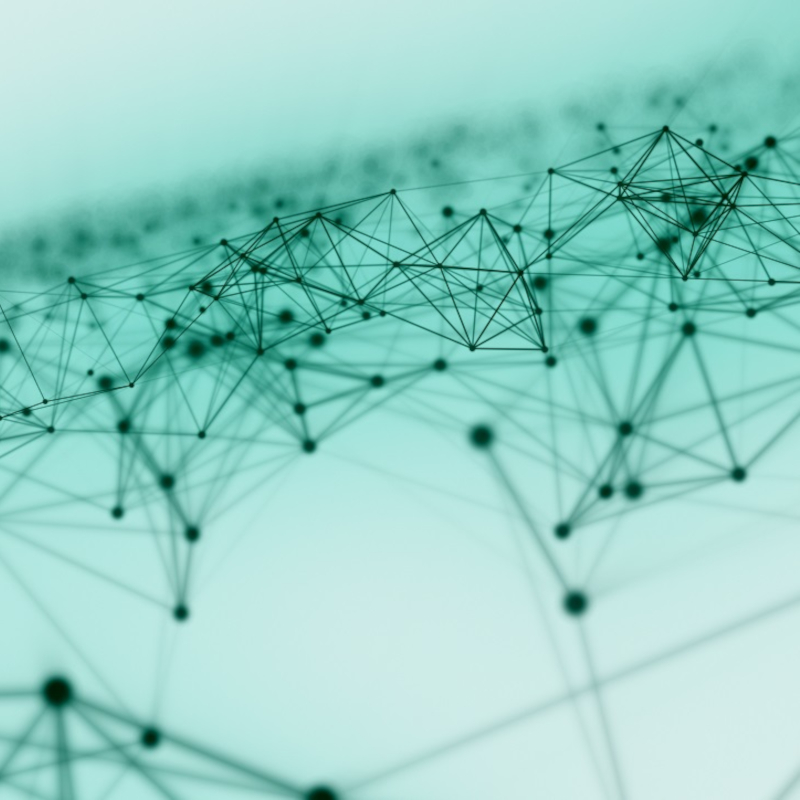
This year’s theme is “Peer Review and the Future of Publishing”. As noted in the theme announcement, “scholarly publishing is in a period of rapid, transformational change, fuelled by new policies, new business models, new technologies, and a drive toward increased transparency and reproducibility.”[1]
The Royal Society has been creating spaces for public discussion about AI technologies, and their implications for individuals, communities, and societies. Work such as the You and AI project, and the Society’s AI Narratives project bring cutting-edge research to a public audience and have been examining how society talks about AI, and why this matters. These conversations have enabled a deeper understanding of AI while addressing critical issues to ensure its responsible and effective implementation in the scientific community. AI policies in scholarly publishing aim to balance the potential benefits of AI with ethical, legal, and quality considerations, while also ensuring fair and equitable access to research outputs.
We speak with Denisse Albornoz, Science Policy adviser in the Royal Society’s Data team, about the policies that will likely evolve as AI technology continues to advance and its impact on scholarly publishing becomes more pronounced.
Could you tell us a bit about AI governance and why we need it?
AI governance focuses on establishing guidelines, regulations, or frameworks that can help us govern how we use, design, and deploy AI technologies. The end goal is to ensure that we maximise the benefits of AI and minimise or eliminate potential harms.
For example, in the Data team, we are exploring how AI is transforming scientific research. AI is enhancing the abilities of scientists, enabling them to tackle more difficult questions and solve pressing societal and environmental challenges that were previously unsolvable. However, there are risks associated with incorporating AI into scientific workflows.
Data and AI governance frameworks can help scientists and regulators address these risks and allocate resources to ensure that scientific progress aided by AI is ethical, safe, and trustworthy.
How do think AI might change scholarly communication (for better or for worse)?
Positive developments
AI can be used to address time-consuming stylistic or language issues for scientists who struggle with writing, freeing up their time to focus on the substance rather than the presentation of their articles. There are some equity implications of this development. AI-assisted writing could support scientists who need to write articles in their second or third language and help them meet the stylistic standards of publication. Using AI to address these barriers can also lower bias from readers and human reviewers who could make erroneous assessments on the quality of the content based on formatting or stylistic errors.
There is also potential for using AI technologies to widen access to scientific outputs for diverse publics. It can, for example, widen the geographic scope of the science we consume. It could support researchers who do not speak English as a first language or researchers who only speak English, reach, and understand research produced in other countries and languages. AI can also help us tackle inaccessibility in science, supporting the production and dissemination of scientific knowledge in multiple modes (e.g., audio, visual) or creative formats. However, to achieve this potential, the inputs (e.g., training data) of AI models need to be localised and representative of diverse knowledge systems.
Negative developments
The most pressing risk is the generation of scientific misinformation and disinformation. LLMs can be used to generate convincing but false text, data, and graphics that will become increasingly difficult to detect. The publication of scientific disinformation can have deep societal harms (e.g., health or climate disinformation) but also contaminate information available online. This creates an additional risk as the next generation of models trained on web-scraped data can continue to deteriorate in performance and absorb biases and inaccuracies found in fabricated data.
AI is also being used to assist in peer review and plagiarism detection in scholarly publications, even though they are still unreliable and inaccurate. There have been cases of false positives in which text generated by humans has been flagged as generated by AI. Being wrongly accused of not authoring their own work can have an impact on future funding and career progression opportunities for researchers. Using AI to estimate the quality of articles and predict citation counts also risks amplifying biases, putting authors from under-represented disciplines, institutions, or regions at a disadvantage.
What gets you most excited about your work?
Speaking to scientists who speak candidly about their hopes and concerns in the age of AI. There is a lot of excitement and hope around how this technology can benefit humanity – as long as we address potential inequities and harms. We are working to ensure their perspectives are represented in science policy and in the decisions that will shape the future of science.
References:
[1] https://peerreviewweek.wordpress.com/
https://royalsociety.org/topics-policy/data-and-ai/artificial-intelligence/
You can read part 2 of this blog post here.