Links to external sources may no longer work as intended. The content may not represent the latest thinking in this area or the Society’s current position on the topic.
Understanding images in biological and computer vision
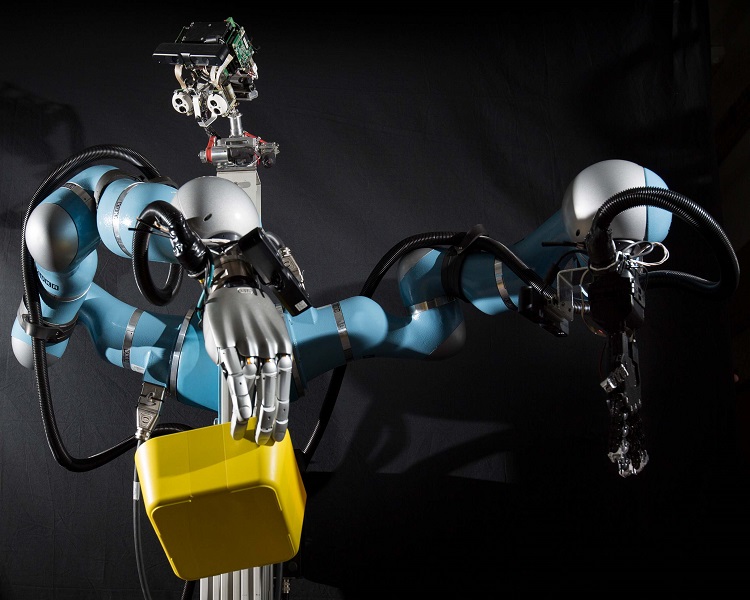
Scientific discussion meeting organised by Dr Andrew Schofield, Professor Aleš Leonardis, Professor Marina Bloj, Professor Iain D Gilchrist and Dr Nicola Bellotto.
Vision appears easy for biological systems but replicating such performance in artificial systems is challenging. Nonetheless we are now seeing artificial vision deployed in robots, cars, mobile and wearable technologies. Such systems need to interpret the world and act upon it much as humans do. This multi-disciplinary meeting discussed recent advances at the junction of biological and computer vision.
Attending the event
This meeting has taken place.
Recorded audio of the presentations are now available on this page. Meeting papers will be published in a future issue of Interface Focus.
Enquires: Contact the Scientific Programmes team.
Schedule
Chair
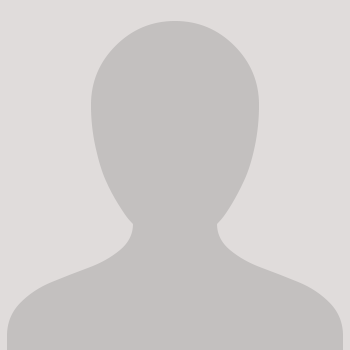
Professor Iain D Gilchrist, University of Bristol, UK
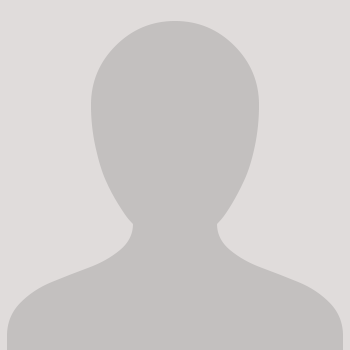
Professor Iain D Gilchrist, University of Bristol, UK
Iain Gilchrist is Professor of Neuropsychology at the University of Bristol. He studied in Durham, Birmingham and Strasbourg before taking up an academic post in Bristol in 1998. His work focuses on Active Vision, which acknowledges that seeing is not a passive process but rather one where the eyes move to explore the visual environment to gather information that is relevant to drive behaviour. Each movement is a decision, and this leads to a distinct set of research questions about those decisions: what determines where we look? And what determined when we move our eye to look at a new location? He has used a wide range of methods in his work including human psychophysics, computational and mathematical modelling, neuropsychology, brain imaging and robotics.
09:45 - 10:00 | Welcome by the Royal Society and Dr Andrew Schofield | |
---|---|---|
10:00 - 10:30 |
Insect vision for robot navigation
Many insects have excellent navigational skills, covering distances, conditions and terrains that are still a challenge for robotics. The primary sense they use is vision, both to obtain self-motion information for path integration, and to establish visual memories of their surroundings to guide homing and route following. Insect vision is relatively low resolution, but exploits a combination of sensory tuning and behavioural strategies to solve complex problems. For example, by filtering for ultraviolet light in an omnidirectional view, segmentation of the shape of the horizon between sky and ground becomes both simple and highly consistent. These shapes can then be used for recognition of location, even under different weather conditions or variations in pitch and tilt. Insect brains are also relatively small and low powered, yet able to produce efficient and effective solutions to complex problems. Through computational modelling, it is becoming possible to link the insights from field experiments to neural data, and thus test hypotheses regarding these brain processing algorithms. For example, we have shown the circuitry of the insect mushroom body neuropil has been shown to be sufficient to support memory of hundreds of images, allowing rapid assessment of familiarity which can be used to guide the animal along previously experienced routes. ![]() Professor Barbara Webb, University of Edinburgh, UK
![]() Professor Barbara Webb, University of Edinburgh, UKBarbara Webb grew up in Sydney, Australia, and studied Psychology at the University of Sydney, obtaining a 1st class BSc Honours degree and university medal in 1988. She moved to Edinburgh to study Artificial Intelligence for her PhD, graduating in 1993; her thesis work to build a robotic cricket was featured in Scientific American. She is a leading researcher in the field of Biorobotics, using robots to model biological systems. She held lectureships in Psychology at the University of Edinburgh (1993-1995), the University of Nottingham (1995-1998) and the University of Stirling (1999-2003), before joining the School of Informatics at the University of Edinburgh as a Reader in 2003. She became a full Professor of Biorobotics in 2010. |
|
10:30 - 11:00 |
Vision for action selection
In the production of visually guided, goal-directed behaviour two types of action may be distinguished. Sampling actions involve shifting gaze in order to acquire information from the visual environment. Manipulative actions involve using effectors in order to alter the state of the environment. Both types of actions are coordinated in a cycle in which noisy information from the environment is used to infer the state of that environment. Given this estimated state, an appropriate course of action has to be selected. Decision theoretic models have been used to account for the selection between possible competing actions within each class. A common feature of these models is that noisy sensory evidence in favour of different action choices is accumulated over time until a decision threshold is reached. The utility of these models will be evaluated for both types of actions. Specifically, the following open questions will be addressed. Is saccade target selection controlled by a "race to threshold" between competing motor programmes in the presence of a foveal load? To what extent is the temporal trigger signal controlled by the foveal processing demands and the selection of the next target? Do the dynamics of real world manipulative actions (reaching and grasping) reflect the underlying decision process? What is the linking function between the temporally evolving decision variable and the different components of manipulative actions? ![]() Dr Casimir Ludwig, University of Bristol, UK
![]() Dr Casimir Ludwig, University of Bristol, UKCasimir was trained as a neuropsychologist at the Radboud University Nijmegen (The Netherlands). He moved to the UK in 1998 and spent almost a year working at the MRC – Cognition and Brain Sciences Unit, before moving on to a PhD at the University of Bristol. After a succession of post-doctoral positions and a 5-year EPSRC Advanced Research Fellowship, he was appointed Reader in Experimental Psychology in August 2012. Casimir's research is focused on the way gaze is used to extract information from the environment, and how the sampled information is then used to infer the state of the environment and guide action. He uses a variety of approaches, including eye tracking, visual psychophysics, motion capture, and computational modelling. |
|
11:00 - 11:15 | Discussion | |
11:15 - 11:45 | Coffee | |
11:45 - 12:15 |
Vision in the context of natural behaviour
Investigation of vision in the context of ongoing behaviour has contributed a number of insights by highlighting the importance of behavioural goals, and focusing attention on how vision and action play out in time. In this context, humans make continuous sequences of sensory-motor decisions to satisfy current goals, and the role of vision is to provide the relevant information for making good decisions in order to achieve those goals. Professor Hayhoe will review the factors that control gaze in natural behaviour, including evidence for the role of the task, which defines the immediate goals, the rewards and costs associated with those goals, uncertainty about the state of the world, and prior knowledge. Visual computations are often highly task-specific, and evaluation of task relevant state is a central factor necessary for optimal action choices. This governs a very large proportion of gaze changes, which reveal the information sampling strategies of the human visual system. When reliable information is present in memory, the need for sensory updates is reduced, and humans can rely instead on memory estimates, depending on their precision, and combine sensory and memory data according to Bayesian principles. It is suggested that visual memory representations are critically important not only for choosing, initiating and guiding actions, but also for predicting their consequences, and separating the visual effects of self-generated movements from external changes. ![]() Professor Mary Hayhoe, University of Texas, USA
![]() Professor Mary Hayhoe, University of Texas, USAMary Hayhoe received her PhD from UC San Diego and has served on the faculty at the University of Rochester and University of Texas at Austin, where she is currently a member of the Center for Perceptual Systems. She served on the Board of Directors of the Vision Sciences Society and was President in 2014-2015. She is on the editorial board of the Journal of Vision and was awarded the Davida Teller Award in 2017. She has pioneered the study of behaviour in in both real and virtual environments and her work addresses how visual perception and action work together to generate behaviour. She has made contributions to understanding human eye movements in natural environments, especially how gaze behavior relates to attention, working memory, and cognitive goals. |
|
12:15 - 12:45 |
Visual perception in legged and dynamic robots
Robotics captures the attention like few other fields of research, however to move beyond controlled laboratory settings dynamic robots need flexible, redundant and trustworthy sensing which pairs with their control systems. In this talk Dr Fallon will discuss the state of research in perception for dynamic robots: specifically robots which move quickly and rely on visual understanding to make their way in the world namely walking and flying robots. Dr Fallon will outline the development of state estimation, mapping and navigation algorithms for humanoid and quadruped robots and describe how they have been demonstrated in real fielded systems. The talk will also overview current limitations, both computational and sensory, and describe some prototype sensing systems which are biologically inspired. The first topic will overview the adaption of accurate registration methods to the Boston Dynamics Atlas and NASA Valkyrie robots where explored the challenge of localization over long baselines and with low sensory overlap. Secondly Dr Fallon will explore how these methods can be fuse with Vision for a dynamic quadruped trotting and crawling in challenging lighting conditions. Lastly, he will present ongoing research in probabilistically fusing proprioceptive state estimation with dense visual mapping to allow a humanoid robot to build a rich dense map while overcoming dynamics, moving objects and challenging lighting conditions. ![]() Dr Maurice Fallon, University of Oxford, UK
![]() Dr Maurice Fallon, University of Oxford, UKMaurice Fallon is a Royal Society University Research Fellow at the Oxford Robotics Institute (University of Oxford). His research is focused on probabilistic methods for localization and mapping. He has also make made research contributions to state estimation for legged robots and is also interested in high dimensional motion planning and manipulation for dynamic robots. He received his PhD from the University of Cambridge and went on to a post doc position in MIT where he work on the problem of Simultaneous Localization and Mapping or SLAM. From 2013-2015, he was the perception lead of MIT's DARPA Robotics Challenge team - a multi-year competition developing technologies for semi-autonomous humanoid exploration and manipulation in disaster situations. After a period in the School of Informatics in the University of Edinburgh, he joined Oxford in 2017. |
|
12:45 - 13:00 | Discussion | |
13:00 - 14:00 | Lunch |
Chair
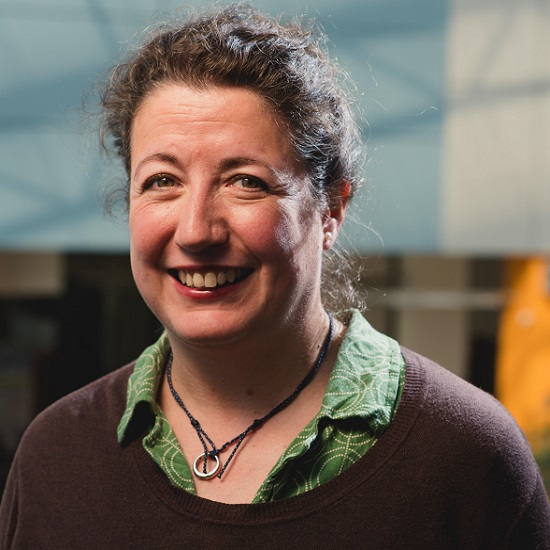
Professor Marina Bloj, University of Bradford, UK
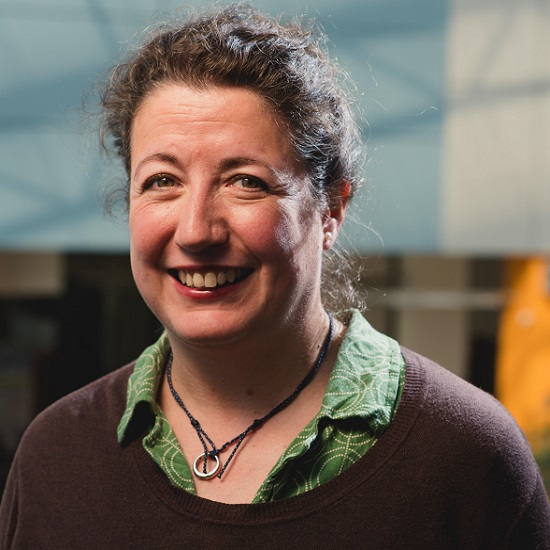
Professor Marina Bloj, University of Bradford, UK
Marina Bloj is Professor of Visual Perception and Associate Director of the cross-faculty Centre for Visual Computing at the University of Bradford. She trained as a physicist (BSc, Universidad de Tucumán, Argentina) and completed post-graduate studies in lighting design, human and computer vision, neuroscience and experimental psychology (MPhil and PhD from the University of Newcastle). Her main research interests lie in exploring how humans perceive objects and material appearance with particular emphasis on colour perception. She conducts research with both real and computer simulated stimuli and has become more interested in trying to establish what constitutes a perceptually realistic virtual experience and what are the technical aspects that limit the realism of simulations.
Professor Bloj has a strong interest in the ethical and societal impact of research and technology. Since 2010 she serves as a Partner Governor on the Bradford Teaching Hospitals NHS Foundation Trust with a particular interest in the adoption of innovation and technology in healthcare. Currently she sits on the executive committees of the Applied Vison Association, British Machine Vision Association and the Colour Group with an agenda to promote interdisciplinary work and support the development and recognition of women in science and technology.
14:00 - 14:30 |
Understanding interactions between object colour, form, and light in human vision
Without light, there would be no colour. But, in human vision, colour is not simply determined by light. Rather, colour is the result of complex interactions between light, surfaces, eyes and brains. Embedded in these are the neural mechanisms of colour constancy, which stabilise object colours under changes in the illumination spectrum. Yet, colour constancy is not perfect, and predicting the colour appearance of a particular object for a particular individual, when viewed under a particular illumination, is not simple. Yellow bananas may remain ever yellow even under fluorescent lamps, but blue dresses may turn white under ambiguous lights. Various factors affect colour appearance: the shape of the object, whether it is 2D or 3D, of a recognisable form or not; its other surface properties, whether it is glossy or matte, textured or uniform; and individual variations in visual processing. The shape of the illumination spectrum also affects colour appearance: metamerism in contemporary lighting provides a new challenge to colour constancy. How to measure colour – a subjective experience – most reliably is another challenge for vision scientists. This talk will describe psychophysical experiments and theory addressing these considerations in our understanding of object colour perception by humans. ![]() Professor Anya Hurlbert, Newcastle University, UK
![]() Professor Anya Hurlbert, Newcastle University, UKAnya Hurlbert is Professor of Visual Neuroscience, Director of the Centre for Translational Systems Neuroscience and Dean of Advancement at Newcastle University. She trained as a physicist (BA, Princeton University), physiologist (MA, Cambridge University), neuroscientist (PhD, Brain and Cognitive Sciences, MIT), and physician (MD, Harvard Medical School). Her main research interest is in understanding the human brain, through understanding the human visual system, and in particular, colour perception; she is also interested in applied areas such as digital imaging and novel lighting technologies. She co-founded the Institute of Neuroscience at Newcastle, and was Institute Director for 10 years. Professor Hurlbert is active in the public understanding of science, and has developed several science-based art exhibitions, most recently an interactive installation at the National Gallery, London, for its 2014 summer exhibition "Making Colour". She is past Chairman of the Colour Group (GB), and currently Scientist Trustee of the National Gallery. |
|
---|---|---|
14:30 - 15:00 |
Critical contours link generic image flows to salient surface organisation
Shape inferences from images, or line drawings, are classical ill-posed inverse problems. Computational researchers mainly seek 'priors' for regularisation, e.g. regarding the light source, or scene restrictions for training neural networks, such as indoor rooms. While of technical interest, such solutions differ in two fundamental ways from human perception: (i) our inferences are largely robust across lighting and scene variations; and (ii) individuals only perceive qualitatively, not quantitatively, the same shape from a given image. Importantly, we know from psychophysics that similarities across individuals concentrate near certain configurations, such as ridges and boundaries, and it is these configurations that are often represented in line drawings. Professor Zucker will introduce a method for inferring qualitative 3D shape from shading that is consistent with these observations. For a given shape, certain shading patches become equivalent to “line drawings” in a well-defined shading-to-contour limit. Under this limit, and invariantly, the contours partition the surface into meaningful parts using the Morse-Smale complex. Critical contours are the (perceptually) stable parts of this complex and are invariant over a wide class of rendering models. The result provides a topological organisation of the surface into 'bumps' and 'dents' from the underlying shading geometry, and provides an invariant linking image gradient flows to surface organisation. ![]() Professor Steven Zucker, Yale University, USA
![]() Professor Steven Zucker, Yale University, USASteven W Zucker works in computational vision, computational neuroscience, and computational biology. He is the David and Lucile Packard Professor of Computer Science at Yale University, and also Professor of Biomedical Engineering. He is a member of the Program in Applied Mathematics, which he directed from 2003 to 2009, and a member of the Interdepartmental Neuroscience Program. Currently he co-directs the Swartz Center, which fosters collaborative research and interdisciplinary training in computational and systems neuroscience. Zucker was elected a Fellow of the Royal Society of Canada, the Canadian Institute for Advanced Research, a Fellow of the IEEE, and (by)Fellow of Churchill College, Cambridge. He won the Siemens Award and was recently named a Distinguished Investigator by the Paul G Allen Family Foundation. |
|
15:00 - 15:15 | Discussion | |
15:15 - 15:45 | Tea | |
15:45 - 16:15 |
Colour and illumination in computer vision
In computer vision, illumination is considered to be a problem that needs to be ‘solved’. The colour bias due to illumination is removed to support colour-based image recognition, stable tracking (in and out of shadows) amongst other tasks. In this talk Professor Finlayson will review historical and current algorithms for illumination estimation. In the classical approach, the illuminant colour is estimated by an - ever more sophisticated - analysis of simple image summary statistics. More recently, the full power - and much higher complexity - of deep learning has been deployed (where, effectively, the definition of the image statistics of interest are found as part of the overall optimisation). Professor Finlayson will challenge the orthodoxy of deep learning i.e. that it is the obvious solution to illuminant estimation. Instead he will propose that the estimates made by simple algorithms are biased and this bias can be corrected to deliver leading performance. The key new observation in our method - bias correction has been tried before with limited success - is that the bias must be corrected in an exposure invariant way. ![]() Professor Graham Finlayson, University of East Anglia, UK
![]() Professor Graham Finlayson, University of East Anglia, UKGraham Finlayson is a professor of computer science at the University of East Anglia where he leads the Colour and Imaging Lab. Graham's research spans colour image processing, physics-based computer vision and visual perception. He is interested in taking the creative spark of an idea, developing the underlying theory and algorithms and then implementing and commercialising the technology. Graham's IP ships in 10s of millions of products. |
|
16:15 - 16:45 |
Resolution of visual ambiguity: interactions in the perception of colour, material and illumination
A key goal of biological image understanding is to extract useful perceptual representations of the external world from the images that are formed on the retina. This is challenging for a variety of reasons, one of which is that multiple configurations of the external world can produce the same retinal image, making the image-understanding problem fundamentally under constrained. None-the-less, biological systems are able to combine constraints provided by the retinal image with those provided by statistical regularities in the natural environment to produce representations that are well correlated with physical properties of the external world. One example of this is provided by our ability to perceive object colour and material properties. These percepts are correlated with object spectral surface and geometric surface reflectance, respectively. But the retinal image formed of an object depends not only on object surface reflectance, but also on the spatial and geometric properties of the illumination. This dependence in turn leading to ambiguity that perceptual processing must resolve. To understand how such processing works, it is necessary to measure how perception is used to identify object colour and material, and how such identification depends on object surface reflectance (both spatial and spectral) as well as on object-extrinsic factors such as the illumination. Classically, such measurements have been made using indirect techniques, such as matching by adjustment or naming. This talk will introduce a novel measurement method that uses object selection directly, together with a model of underlying perceptual representation, to study the stability of object colour across changes in illumination, as well as how object colour and material trade off in identification. ![]() Professor David H Brainard, University of Pennsylvania, USA
![]() Professor David H Brainard, University of Pennsylvania, USADavid H Brainard is the RRL Professor of Psychology at the University of Pennsylvania. He received an AB in Physics (Magna Cum Laude) from Harvard University (1982) and an MS (Electrical Engineering) and PhD (Psychology) from Stanford University in 1989. He is currently the RRL Professor of Psychology at the University of Pennsylvania and his research focuses on colour vision and colour image processing. He is a fellow of the Optical Society, ARVO and the Association for Psychological Science. At present, he directs Penn's Vision Research Center, co-directs Penn's Computational Neuroscience Initiative, is on the Board of the Vision Sciences Society, and is a member of the editorial board of the Journal of Vision. |
|
16:45 - 17:00 | Discussion |
Chair
Dr Nicola Bellotto, University of Lincoln, UK
Dr Nicola Bellotto, University of Lincoln, UK
Dr Nicola Bellotto is a Reader in Computer Science at the University of Lincoln and a member of the Lincoln Centre for Autonomous Systems. His research interests are mostly in machine perception for robotics and autonomous systems, including sensor fusion, active vision and qualitative spatial reasoning for human monitoring and activity recognition. He holds a Laurea in Electronic Engineering from the University of Padua and a PhD in Computer Science from the University of Essex. Before joining Lincoln, he was a postdoctoral researcher in the Active Vision Laboratory at the University of Oxford. Dr Bellotto is also a Principal Investigator in two large EU Horizon 2020 projects, ENRICHME and FLOBOT; he was the recipient of a Google Faculty Research Award in 2015, and chair of the 2017 BMVA Computer Vision Summer School.
Chair
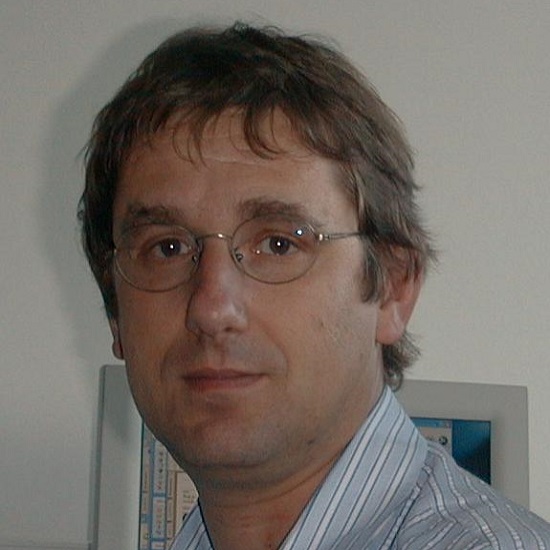
Professor Aleš Leonardis, University of Birmingham, UK
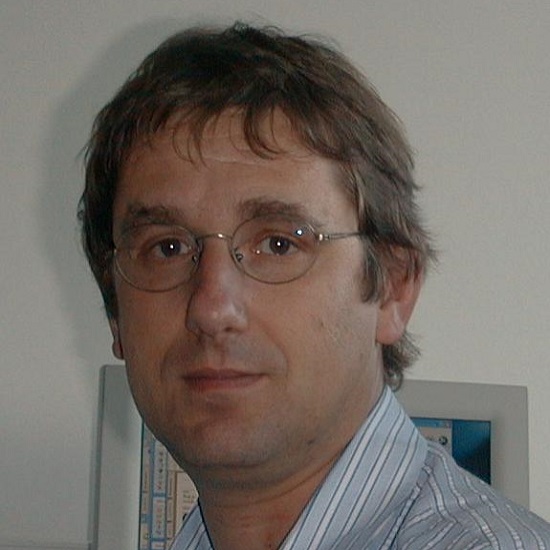
Professor Aleš Leonardis, University of Birmingham, UK
Aleš Leonardis is Chair of Robotics and Co-Director of the Computational Neuroscience and Cognitive Robotics Centre at the University of Birmingham, United Kingdom. He is also Professor of Computer and Information Science at the University of Ljubljana, Slovenia and an Adjunct Professor at the Faculty of Computer Science, Graz University of Technology, Austria. His research interests include robust and adaptive methods for computer/cognitive vision, object and scene recognition and categorization, statistical visual learning, object tracking, and biologically motivated vision - all in a broader framework of artificial cognitive systems and robotics. He was Program Co-chair of the European Conference on Computer Vision 2006, and he has been an Associate Editor of the IEEE Transactions on Pattern Analysis and Machine Intelligence, an editorial board member of Pattern Recognition and Image and Vision Computing, and an editor of the Springer book series Computational Imaging and Vision. Aleš Leonardis is a Fellow of the International Association of Pattern recognition and a recipient of the 29th Annual Pattern Recognition Society award.
09:30 - 10:00 |
Neural Mechanisms Underlying the Development of Face Recognition
How do brain mechanisms develop from childhood to adulthood leading to better face recognition? There is extensive debate if brain development is due to pruning of excess neurons, synapses, and connections, leading to reduction of responses to irrelevant stimuli, or if development is associated with growth of dendritic arbors, synapses, and myelination leading to increased responses and selectivity to relevant stimuli. Dr Grill-Spector’s research addresses this central debate using cutting edge multimodal imaging in children (ages 5-12) and adults. In her talk, Dr Grill-Spector, will present compelling empirical evidence supporting the growth hypothesis. Anatomically, her recent research has discovered developmental increases in macromolecular tissue volume which is correlated with specific increases in both functional selectivity to faces, as well as improvements in face recognition. Functionally, her results reveal that across childhood development, face-selective regions not only increase in size and selectivity to faces, but also show increases in their neural sensitivity to face identity, in turn improving perceptual discriminability among faces. Finally, Dr Grill-Spector will show how visual experience during development, such as looking behavior, may play a role in sculpting population receptive fields in face-selective regions. Together, these data suggest that both anatomical and functional development play a role in the development of face recognition ability. These results are important as they propose a new model by which emergent brain function and behavior during childhood result from cortical tissue growth rather than from pruning. ![]() Dr Kalanit Grill-Spector, Stanford University, USA
![]() Dr Kalanit Grill-Spector, Stanford University, USADr Grill-Spector studied Electrical Engineering and Computer Science at Ben Gurion University in Israel. Then she obtained a PhD in Computer Science and Neurobiology, at the Weizmann Institute of Science in Israel supervised by Dr Rafael Malach and Dr Shimon Edelman. She did a postdoctoral fellowship with Dr Nancy Kanwisher at the department of Brain and Cognitive Sciences at MIT. She is a professor of Psychology and a member of the Stanford Neurosciences Institutes at Stanford University since September 2001. Her research uses multimodal imaging, computational methods, and behavioural measurements to elucidate the neural mechanisms of visual recognition and perception in humans and how these neural processes develop from childhood to adulthood. |
|
---|---|---|
10:00 - 10:30 |
Recognition in computer vision
Recent advances in visual recognition in computer vision owe significantly to the adoption of deep neural networks trained on large image datasets that have been annotated by human observers. Professor Malik will review state of the art techniques on problems such as object detection, instance segmentation, action recognition and human pose and shape estimation. These techniques have also advanced methods for 3D shape recovery from single images. Professor Malik will also point to areas for future work and ways in which computer vision still falls short of biological vision. ![]() Professor Jitendra Malik, University of California, USA
![]() Professor Jitendra Malik, University of California, USAJitendra Malik is Arthur J Chick Professor of EECS at UC Berkeley, and a Director of Research at Facebook AI Research in Menlo Park. He has published widely in computer vision, computational modeling of human vision, and machine learning. Several well-known concepts and algorithms arose in this research, such as anisotropic diffusion, normalized cuts, high dynamic range imaging, shape contexts and R-CNN. Jitendra received the Distinguished Researcher in Computer Vision Award from IEEE, the K.S. Fu Prize from IAPR, and the Allen Newell award from ACM and AAAI. He has been elected to the National Academy of Sciences, the National Academy of Engineering and the American Academy of Arts and Sciences. |
|
10:30 - 10:45 | Discussion | |
10:45 - 11:15 | Coffee | |
11:15 - 11:45 |
Frequency-resolved correlates of visual object recognition revealed by deep convolutional neural networks
Previous work demonstrated a direct correspondence between the hierarchy of the human visual areas and layers of deep convolutional neural networks (DCNN) trained on visual object recognition. In this talk it will be presented how DCNNs are used to investigate which frequency bands carry feature transformations of increasing complexity along the ventral visual pathway. By capitalizing on intracranial depth recordings from 100 patients and 11293 electrodes it was assessed the alignment between the DCNN and signals at different frequency bands in different time windows. It was found that activity in low and high gamma bands was aligned with the increasing complexity of visual feature representations in the DCNN. These findings show that activity in the gamma band is not only a correlate of object recognition, but carries increasingly complex features along the ventral visual pathway. These results demonstrate the potential that modern artificial intelligence algorithms have in advancing our understanding of the brain. Finally, it will be discussed how far can we compare animal and current artificial systems of perception and cognition. ![]() Dr Raul Vicente, University of Tartu, Estonia
![]() Dr Raul Vicente, University of Tartu, EstoniaRaul Vicente is professor of Data Science at the Institute of Computer Science at UTartu. His research is focused on neuroscience, machine learning, and artificial intelligence. He received the PhD Extraordinary Award in Physics of the University of the Balearic Islands in 2006, and the 2007 QEOD Prize of the European Physical Society for the best PhD Thesis in Applied Optics. He was a visiting scholar at the Department of Electrical Engineering at UCLA (USA) during 2003 and 2004, and junior fellow at the Max-Planck Institute for Brain Research in Frankfurt (Germany) from 2006 to 2013. He has authored more than 40 articles in peer-reviewed journals and co-edited of the book “Directed information measures in Neuroscience”. |
|
11:45 - 12:15 |
Image understanding beyond object recognition
Computational models of vision have advanced in recent years at a rapid rate, rivaling in some areas human-level performance. Much of the progress to date has focused on analyzing the visual scene at the object level – the recognition and localisation of objects in the scene. Human understanding of images reaches a richer and deeper image understanding both ‘below’ the object level, such as identifying and localizing objects’ part and sub-parts, as well as ‘above’ the object levels, such as identifying object relations, and agents with their actions and interactions. In both cases, understanding depends on recovering meaningful structures in the image, their components, properties, and inter-relations. The talk will describe new directions, based on human and computer vision studies, towards human-like image interpretation, beyond the reach of current schemes, both below the object level, (based on the perception of so-called ‘minimal images’), as well as the level of meaningful configurations of objects, agents and their interactions. In both cases the interpretation process depends on combining ‘bottom-up’ processing, proceeding from the images to high-level cognitive levels, together with ‘top-down’ processing, proceeding from cognitive levels to lower-levels image analysis. ![]() Professor Shimon Ullman Weizmann Institute of Science, Israel
![]() Professor Shimon Ullman Weizmann Institute of Science, IsraelShimon Ullman is the Samy and Ruth Cohn Professor of Computer Science at the Weizmann Institute of Science. Prior to this position, he was a Professor at the Brain and Cognitive Science and the AI Laboratory at MIT. His areas of research combine computer and human vision, human cognition, and brain modeling. He obtained his B.Sc. in Mathematics, Physics and Biology, at the Hebrew University of Jerusalem, and Ph.D. in Electrical Engineering and Computer Sciences, at the Artificial Intelligence Laboratory in the Massachusetts Institute of Technology. He is a recipient of the 2008 David E Rumelhart Prize in human cognition, the 2014 Emet Prize for Art, Science and Culture, and the 2015 Israel Prize in Computer Science. He is a member of the Israeli Academy of Sciences and Humanities, and the American Academy of Arts and Sciences. |
|
12:15 - 12:30 | Discussion | |
12:30 - 13:30 | Lunch |
Chair
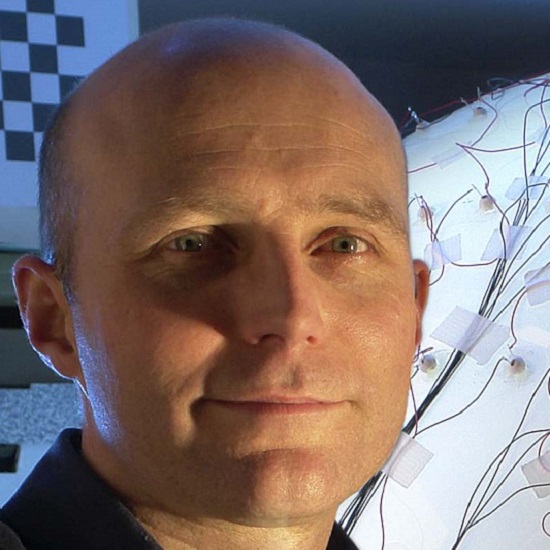
Dr Andrew Schofield, University of Birmingham, UK
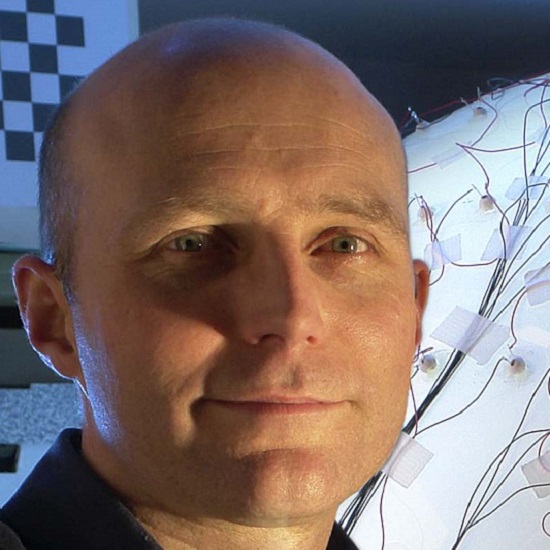
Dr Andrew Schofield, University of Birmingham, UK
With a Bachelor’s degree in Electronics and a PhD in Communication and Neuroscience Andrew Schofield is a multidisciplinary thinker whose work on visual perception crosses the boundaries between Psychology, Neuroscience and Computer Science. After receiving his PhD Andrew completed a Research Fellowship in image processing at Brunel University and then worked for a year in the Civil Service before returning to academia in 1996 as a Research Fellow in Psychology at Birmingham. He was appointed Lecturer in 1999 and Senior Lecturer in 2006. He is a past-chair of the Applied Vision Association and lead the EPSRC Network: Visual Image Interpretation in Humans and Machines. His research interests include the perception of visual texture, shape-from-shading and the discrimination of illumination and reflectance changes.
13:30 - 14:00 |
What are the computations underlying primate versus machine vision?
Primates excel at object recognition: For decades, the speed and accuracy of their visual system have remained unmatched by computer algorithms. But recent advances in Deep Convolutional Networks (DCNs) have led to vision systems that are starting to rival human decisions. A growing body of work also suggests that this recent surge in accuracy is accompanied by a concomitant improvement in our ability to account for neural data in higher areas of the primate visual cortex. Overall, DCNs have become de facto computational models of visual recognition. This talk will review recent work by the Serre lab bringing into relief limitations of DCNs as computational models of primate vision. Results will be presented showing that visual features learned by DCNs from large-scale object recognition databases differ markedly from those used by human observers during visual recognition. Evidence will be presented suggesting that the depth of visual processing achieved by modern DCN architectures is greater than that achieved by human observers. Finally, it will be shown that DCNs are limited in their ability to solve seemingly simple visual reasoning problems involving similarity and spatial relation judgments suggesting the need for additional neural computations beyond those implemented in modern visual architectures. ![]() Dr Thomas Serre, Brown University, USA
![]() Dr Thomas Serre, Brown University, USADr Serre is Associate Professor in Cognitive Linguistic & Psychological Sciences at Brown University. He received a PhD in computational neuroscience from MIT (Cambridge, MA) in 2006 and an MSc in EECS from Télécom Bretagne (Brest, France) in 2000. His research focuses on understanding the brain mechanisms underlying the recognition of objects and complex visual scenes using a combination of behavioural, imaging and physiological techniques. These experiments fuel the development of quantitative computational models that try not only to mimic the processing of visual information in the cortex but also to match human performance in complex visual tasks. He is the recipient of an NSF early career award and a DARPA young faculty award. His research has been featured in the BBC series "Visions from the Future" and appeared in several news articles (The Economist, New Scientist, Scientific American, IEEE Computing in Science and Technology, Technology Review and Slashdot). |
|
---|---|---|
14:00 - 14:30 |
Learning about shape
Vision is naturally concerned with shape. If we could recover a stable and compact representation of object shape from images, we would hope it might aid with numerous vision tasks. Just the silhouette of an object is a strong cue to its identity, and the silhouette is generated by its 3D shape. In computer vision, many representations have been explored: collections of points, “simple” shapes like ellipsoids or polyhedra, algebraic surfaces and other implicit surfaces, generalised cylinders and ribbons, and piecewise (rational) polynomial representations like NURBS and subdivision surfaces. Many of these can be embedded more or less straightforwardly into probabilistic shape spaces, and recovery (a.k.a. “learning”) of one such space is the goal of the experimental part of this talk. When recovering shape from measurements, there is at first sight a natural hierarchy of stability: straight linBies can represent very little but may be robustly recovered from data, then come conic sections, splines with fixed knots, and general piecewise representations. I will show, however, that one can pass almost immediately to piecewise representations without loss of robustness. In particular, I shall show how a popular representation in computer graphics—subdivision curves and surfaces—may readily be fit to a variety of image data using the technique for ellipse fitting introduced by Gander, Golub, and Strebel in 1994. I show how we can address the previously-difficult problem of recovering 3D shape from multiple silhouettes, and the considerably harder problem which arises when the silhouettes are not from the same object instance, but from members of an object class, for example 30 images of different dolphins each in different poses. ![]() Dr Andrew Fitzgibbon FREng, Microsoft, UK
![]() Dr Andrew Fitzgibbon FREng, Microsoft, UKDr Andrew Fitzgibbon has been closely involved in the delivery of three groundbreaking computer vision systems over two decades. In 2000, he was computer vision lead on the Emmy-award-winning 3D camera tracker "Boujou"; in 2009 he introduced large-scale synthetic training data to Kinect for Xbox 360, and in 2019 was science lead on the team that shipped fully articulated hand tracking on HoloLens 2. His passion is bringing the power of mathematics to the crucible of real-world engineering. He has numerous research awards, including twelve "best paper" or "test of time" prizes at leading conferences, and is a Fellow of the UK’s Royal Academy of Engineering. |
|
14:30 - 14:45 | Discussion | |
14:45 - 15:15 | Tea | |
15:15 - 15:45 |
The emergence of polychronization and feature binding in a spiking neural network model of the primate ventral visual system
Simulations of a biologically realistic ‘spiking’ neural network model of the primate ventral visual pathway are presented, in which the timings of action potentials are explicitly emulated. It is shown how the higher layers of the network develop regularly repeating spatiotemporal patterns of spiking activity that represent the visual objects on which the network is trained. This phenomenon is known as polychronization. In particular, embedded within the subpopulations of neurons with regularly repeating spike trains, called polychronous neuronal groups, are neurons that represent the hierarchical binding relations between lower level and higher level visual features. Such neurons are termed binding neurons. In the simulations, binding neurons learn to represent the binding relations between visual features across the entire visual field and at every spatial scale. In this way, the emergence of polychronization begins to provide a plausible way forwards to solving the classic binding problem in visual neuroscience, which concerns how the visual system represents the (hierarchical) relations between visual features within a scene. Such binding information is necessary for the visual brain to be able to make semantic sense of its visuospatial world, and may be needed for the future development of artificial general intelligence (AGI) and machine consciousness (MC). Evidence is also provided for the upward projection of visuospatial information at every spatial scale to the higher layers of the network, where it is available for readout by later behavioural systems. This upwards projection of spatial information into the higher layers is referred to as the "holographic principle". ![]() Dr Simon Stringer, University of Oxford, UK
![]() Dr Simon Stringer, University of Oxford, UKDr Simon Stringer has been a research mathematician at Oxford University for about 25 years. He has worked across a range of different areas of applied mathematics such as control systems, computational aerodynamics and epidemiology. For the last decade, he has led the Oxford Centre for Theoretical Neuroscience and Artificial Intelligence - www.oftnai.org. The centre houses a team of theoreticians who are modelling various aspects of brain function, including vision, navigation, behaviour, audition and speech recognition. The aim of the centre is to develop integrated models of the brain that display the rudiments of artificial general intelligence and machine consciousness within the next twenty years. |
|
15:45 - 16:15 |
SpiNNaker: spiking neural networks for computer vision
The SpiNNaker (Spiking Neural Network Architecture) machine is a many-core digital computer optimised for the modelling of large-scale systems of spiking neurons in biological real time. The current machine incorporates half a million ARM processor cores and is capable of supporting models with up to a hundred million neurons and a hundred billion synapses, or about the network scale of a mouse brain, though using greatly simplified neuron models compared with the formidable complexity of the biological neuron cell. One use of the system is to model the biological vision pathway at various levels of detail; another is to build spiking analogues of the Convolutional Neural Networks used for image classification in machine learning and AI applications. In SpiNNaker the equations describing the behaviours of neurons and synapses are defined in software, offering flexible support for novel dynamics and plasticity rules, including structural plasticity. This work is at an early stage, but results are already beginning to emerge that suggest possible mechanisms whereby biological vision systems may learn the statistics of their inputs without supervision and with resilience to noise, pointing the way to engineered vision systems with similar on-line learning capabilities. ![]() Professor Steve Furber CBE FREng FRS, University of Manchester
![]() Professor Steve Furber CBE FREng FRS, University of ManchesterSteve Furber CBE FREng FRS is ICL Professor of Computer Engineering in the School of Computer Science at the University of Manchester, UK. After completing a BA in mathematics and a PhD in aerodynamics at the University of Cambridge, UK, he spent the 1980s at Acorn Computers, where he was a principal designer of the BBC Microcomputer and the ARM 32-bit RISC microprocessor. Over 100 billion variants of the ARM processor have since been manufactured, powering much of the world's mobile and embedded computing. He moved to the ICL Chair at Manchester in 1990 where he leads research into asynchronous and low-power systems and, more recently, neural systems engineering, where the SpiNNaker project is delivering a computer incorporating a million ARM processors optimised for brain modelling applications. |
|
16:15 - 16:45 | Panel discussion |