Links to external sources may no longer work as intended. The content may not represent the latest thinking in this area or the Society’s current position on the topic.
Computation by natural systems
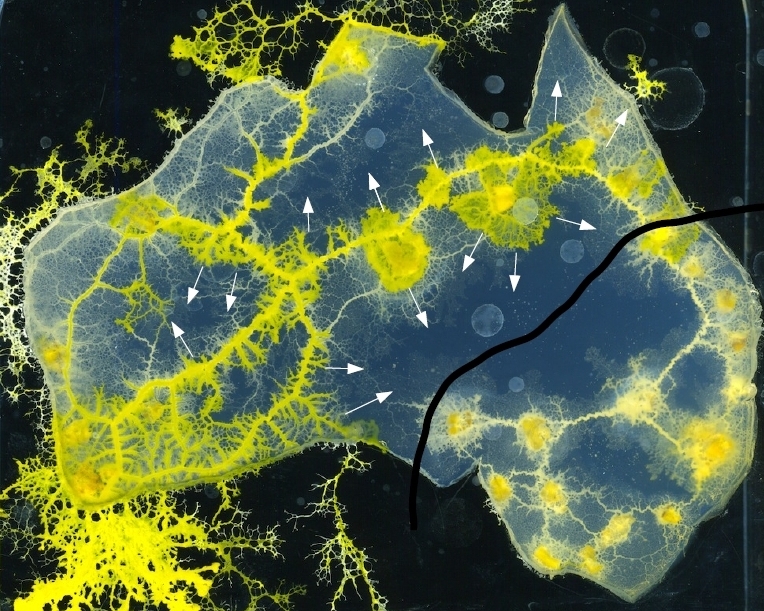
Theo Murphy scientific meeting organised by Dr Dominique Chu, Professor Christian Ray and Professor Mikhail Prokopenko
Over recent years it has become clear in various sciences that many natural systems perform computations. Research into the properties of these natural computers remains fragmented along disciplinary boundaries between computer science, physics, engineering and biology. The objective of this meeting was to overcome this fragmentation by bringing together researchers from different fields to discuss their latest finding on natural computation.
Enquiries: please contact the Scientific Programmes team
Schedule
09:20 - 09:45 |
Fundamental limits on the thermodynamics of circuits
The thermodynamics of computation specifies the minimum amount that entropy must increase in the environment of any physical system that implements a given computation, when there are no constraints on how the system operates (the so-called “Landauer limit”). However common engineered computers use digital circuits that physically connect separate gates in a specific topology. Each gate in the circuit performs its own “local” computation, with no a priori constraints on how it operates. In contrast, the circuit’s topology introduces constraints on how the aggregate physical system implementing the overall “global” computation can operate. These constraints cause additional minimal entropy increase in the environment of the overall circuit, beyond that caused by the individual gates. ![]() Professor David Wolpert, Santa Fe Institute, USA
![]() Professor David Wolpert, Santa Fe Institute, USADavid Wolpert is a professor at the Santa Fe Institute, visiting professor at MIT, and adjunct professor at ASU. He is the author of three books, over 200 papers, has three patents, is an associate editor at over half a dozen journals, has received numerous awards, and is a fellow of the IEEE. |
|
---|---|---|
10:00 - 10:30 |
Entropy and information in natural complex systems
The metaphor of a potential epigenetic differentiation landscape broadly suggests that during differentiation a stem cell follows the steepest descending gradient toward a stable equilibrium state which represents the final cell type. It has been conjectured that there is an analogy to the concept of entropy in statistical mechanics. In order to assess these predictions, Wiesner et al. computed the Shannon entropy for time-resolved single-cell gene expression data in two different experimental setups of haematopoietic differentiation. They found that the behaviour of this entropy measure is in contrast to these predictions. This is one of very few examples where information theory gives direct insights into a measurable biochemical process. This talk will discuss these findings and their interpretation. And an overview will be given over information theoretic measures of complexity which might quantify the computation of such processes. ![]() Associate Professor Karoline Wiesner, Bristol University, UK
![]() Associate Professor Karoline Wiesner, Bristol University, UKKaroline Wiesner received a PhD in physics from Uppsala University, and has held postdoctoral positions at the Santa Fe Institute and at the University of California, Davis. Since 2007 she is faculty member of the School of Mathematics at the University of Bristol, where she also co-directs the Bristol Centre for Complexity Sciences. Her work focuses on information theoretic methods for applications in the sciences (e.g. protein dynamics, glass formers, stem cells), and on one foundations of complexity. |
|
11:00 - 11:30 |
Thermodynamics of computation with chemical reaction networks
Computing can be seen as transformations between nonequilibrium states. In stochastic thermodynamics, the second law can be used to provide a direct connection between the thermodynamic cost needed to induce such transformations and the distance from equilibrium (measured as a relative entropy) of these nonequilibrium states. This nonequilibrium Landauer principle not only holds for detailed balanced dynamics but also for driven non-detailed balanced ones. Remarkably, a closely related result also holds for open chemical reaction networks described by deterministic mass action law kinetics, even in presence of diffusion. These results lay the foundations for a systematic study of the thermodynamic cost needed to produce chemical spatio-temporal signals which play a key role in biology and chemical computing. ![]() Professor Massimiliano Esposito, Université du Luxembourg, Luxembourg
![]() Professor Massimiliano Esposito, Université du Luxembourg, LuxembourgProfessor Massimiliano Esposito is a theoretical physicist specializing in statistical physics and the study of complex systems. His current research focuses on energy and information processing in small quantum systems and biological systems, in particular chemical reaction networks. He obtained his PhD in 2004 at Université Libre de Bruxelles (ULB) in Belgium. After two postdocs in California at UC Irvine and UC San Diego, he came back as a contract researcher for two years at ULB. In 2012 he was awarded a five year Attract Fellowship by the National Research Fund of Luxembourg to start his own research group "Complex Systems and Statistical Mechanics" in the Physics and Materials Science Research Unit at the University of Luxembourg. In 2016 he became Professor of Theoretical Physics and was awarded a five years Consolidator Grant by the European Research Council. |
|
11:45 - 12:30 |
Computation in the ground state: from spin models to memristors
We will describe two aspects of ongoing current research on (classical and analogic) computation using spin models and memristors (resistors with memory). We will first discuss how a simple Ising spin model can be turned into a bottom-up, programmable logic gate computational model. As I will show, it is possible to encode logic gates in the ground state of a spin system using only local external fields, and this model can serve as a proof of concept for more complicated computations using nanomagnets. I will briefly describe at the end why a completely different physical device, the memristor, performs a similar yet different type of computation. Memristors are simply resistors which change resistance as currents flows through them, and are thought of as low-energy 2-ports computational devices which can be used for neuromorphic (brain-inspired) computing. We conclude discussing future experiments to provide a more accurate mapping of memristive annealer to Ising models at an effective (hopefully low?) temperature. ![]() Dr Francesco Caravelli, Los Alamos National Laboratory, USA
![]() Dr Francesco Caravelli, Los Alamos National Laboratory, USAI began studying Quantum Gravity for my PhD at UWaterloo/Perimeter Institute, but right after I got interested in statistical mechanics and in systems out of equilibrium. Before working at the Los Alamos National Laboratory, I have been a joint postdoc between the SFI (USA) and OCIAM Oxford (UK) with Doyne Farmer, a researcher at UCL (UK) with Francesca Medda and a Senior Researcher at Invenia Labs in Cambridge (UK). Specifically, I have focused on dynamical graphs, neuromorphic circuits, and in particular memristors and their collective behavior. Memristors are 2-port passive devices, which have characteristics similar to a resistance but exhibit a very nonlinear behavior. Neuromorphic circuits are, in general, interesting for many reasons. These provide in fact an alternative to the von Neumann architecture at the classical level using analog computation. I have recently started to explore the kinetic behavior of artificial spin ice (magnetic nanoisland), and how to harness their interaction complexity for computation purposes. |
13:30 - 14:00 |
Robust accessible states allow efficient training of neural networks with very low precision stochastic synapses
The problem of training neural networks is in general non-convex and in principle computationally hard. This is true even for single neurons, when synapses are only allowed a few bits of precision. In practice, however, many fairly greedy heuristics exhibit surprisingly good results. A large-deviations analysis performed with statistical physics tools has shown that efficient learning is made possible in these models by the existence of rare but very dense regions of optimal configurations, which have appealing robustness and generalization properties. The analysis also shows that the capacity of the networks saturates fast with the number of synaptic values and thus indicates that very few bits are indeed sufficient for effective learning. Further analyses also show that these regions can be directly targeted by learning algorithms, in various ways: a particularly simple one consists in exploiting synaptic fluctuations, offering an appealing avenue of investigation to interpret the high levels of stochasticity observed in biological neurons. ![]() Professor Carlo Baldassi, Bocconi University, Milan, Italy
![]() Professor Carlo Baldassi, Bocconi University, Milan, ItalyCarlo Baldassi is assistant professor at Bocconi University. His has a background in theoretical physics, and did his Ph.D. in complex systems in biology, with a thesis in computational neuroscience. His research interests focus on the application of analytical and algorithmic tools of statistical mechanics of disordered systems to computational neuroscience, machine learning, computational biology, and more generally to large-scale inference and optimization problems. |
|
---|---|---|
14:15 - 14:45 |
Computations and control in brain systems
The human brain is a complex organ characterized by a heterogeneous pattern of structural connections that supports long-range functional interactions. Non-invasive imaging techniques now allow for these patterns to be carefully and comprehensively mapped in individual humans, paving the way for a better understanding of how the complex network architecture of structural wiring supports computation, cognition, and behavior. While a large body of work now focuses on descriptive statistics to characterize these wiring patterns, a critical open question lies in how the organization of these networks constrains the potential repertoire of brain dynamics. In this talk, I will describe an approach for understanding how perturbations to brain dynamics propagate through complex wiring patterns, driving the brain into new states of activity. Drawing on a range of disciplinary tools – from graph theory to network control theory and optimization – I will identify control points in brain networks, characterize trajectories of brain activity states following perturbation to those points, and propose a mechanism for how network control evolves in our brains as we grow from children into adults. I will close with a more general discussion of the importance of understanding the role of structural network architecture in computation and cognition. ![]() Professor Danielle S. Bassett, University of Pennsylvania, USA
![]() Professor Danielle S. Bassett, University of Pennsylvania, USA
Danielle S. Bassett is the Eduardo D. Glandt Faculty Fellow and Associate Professor in the Department of Bioengineering at the University of Pennsylvania. She is most well-known for her work blending neural and systems engineering to identify fundamental mechanisms of cognition and disease in human brain networks. She received a B.S. in physics from the Pennsylvania State University and a Ph.D. in physics from the University of Cambridge, UK. Following a postdoctoral position at UC Santa Barbara, she was a Junior Research Fellow at the Sage Center for the Study of the Mind. In 2012, she was named American Psychological Association's `Rising Star' and given an Alumni Achievement Award from the Schreyer Honors College at Pennsylvania State University for extraordinary achievement under the age of 35. In 2014, she was named an Alfred P Sloan Research Fellow and received the MacArthur Fellow Genius Grant. In 2015, she received the IEEE EMBS Early Academic Achievement Award, and was named an ONR Young Investigator. In 2016, she received an NSF CAREER award and was named one of Popular Science’s Brilliant 10. In 2017 she was awarded the Lagrange Prize in Complexity Science. She is the founding director of the Penn Network Visualization Program, a combined undergraduate art internship and K-12 outreach program bridging network science and the visual arts. Her work -- which has resulted in 143 published articles -- has been supported by the National Science Foundation, the National Institutes of Health, the Army Research Office, the Army Research Laboratory, the Alfred P Sloan Foundation, the John D and Catherine T MacArthur Foundation, and the Office of Naval Research
|
|
15:30 - 16:00 |
Energy-efficient information transfer in neural networks
Abstract not yet available. ![]() Professor Renaud Jolivet, University of Geneva and CERN, Switzerland
![]() Professor Renaud Jolivet, University of Geneva and CERN, SwitzerlandRenaud Jolivet studied theoretical biophysics at the University of Lausanne (1996-2001) before doing a PhD in computational neuroscience at École polytechnique fédérale de Lausanne in Lausanne, Switzerland (2001-2005). After his doctoral studies, he was a postdoc in Lausanne (2006-2007) and a fellow in Tokyo, Zürich and London (2007-2015). Since 2016, he is Joint Titular Professor in Medical Physics at CERN and at the University of Geneva, Switzerland. His main interest lies in the organization of brain energy metabolism and in the relation between energy consumption and information processing in neural networks, which his team studies in both experiments and computational models. He also holds a particular interest in the non-neuronal cells of the brain. |
|
16:15 - 16:45 |
The evolution of cellular individuality
Abstract not yet available.![]() Professor Eric Deeds, University of Kansas, USA
![]() Professor Eric Deeds, University of Kansas, USAProf. Deeds received a B.S. in Biochemistry and a B.A. in English from Case Western Reserve University in 2001. He got his Ph.D. from Harvard University in 2005, and his thesis research in the lab of Prof. Eugene Shakhnovich focused on the evolution of protein structure and the biophysics of protein interaction networks. He carried out postdoctoral studies in the lab of Prof. Walter Fontana in the Department of Systems Biology at Harvard Medical school, where his work focused on the dynamics of protein interactions.. In 2010, he became an Assistant Professor at the University of Kansas, and he was promoted to Associate Professor in 2016. Research in his lab focuses on understanding signal transduction and information processing within cells. |
09:00 - 09:30 |
Novel computing substrates
Natural systems do not compute. Humans interpret their space-time development as a computation. Anything around us can be used to design computing circuits. We demonstrate this on examples of collision-based logical circuits implemented in experimental laboratory prototypes and computer models of reaction-diffusion chemical systems, living swarms, slime mould, plants, liquid marbles and protein polymers. In collision-based computers logical values are represented by a space-time position of mobile objects: excitation wavefronts, protoplasmic tubes, solitons, plat roots. Logical gates are realized in interactions between the objects. The experimental and theoretical designs uncovered might not be better than existing silicon architectures but offer us worlds of alternative computation. Principle of information processing in spatially extended chemical systems, slime mould, plants and polymers might inspire us to develop efficient algorithms, design optimal architectures and manufacture working prototypes of future and emergent computing devices. ![]() Professor Andrew Adamatzky, University of the West of England, UK
![]() Professor Andrew Adamatzky, University of the West of England, UKAndrew Adamatzky is Professor of Unconventional Computing and Director of the Unconventional Computing Laboratory, Department of Computer Science, University of the West of England, Bristol, UK. He does research in molecular computing, reaction-diffusion computing, collision-based computing, cellular automata, slime mould computing, massive parallel computation, applied mathematics, complexity, nature-inspired optimisation, collective intelligence and robotics, bionics, computational psychology, non-linear science, novel hardware, and future and emergent computation. He authored seven books, mostly notable are `Reaction-Diffusion Computing’, `Dynamics of Crow Minds’, `Physarum Machines’, and edited twenty-two books in computing, including `Collision Based Computing’, `Game of Life Cellular Automata’, `Memristor Networks’; he also produced a series of influential artworks published in the atlas `Silence of Slime Mould’. He is founding editor-in-chief of ‘J of Cellular Automata’ and “ J of Unconventional Computing’ and editor-in-chief of “J Parallel, Emergent, Distributed Systems’ and ‘Parallel Processing Letters’. |
|
---|---|---|
09:45 - 10:15 |
Computing during development
Abstract not yet available.![]() Professor Nick Monk, University of Sheffield, UK
![]() Professor Nick Monk, University of Sheffield, UKNick Monk is Head of the School of Mathematics and Statistics at the University of Sheffield. Following an undergraduate degree in Mathematics and a PhD in Theoretical Physics, his research interests have been on the development and analysis of mathematical models of biological systems. His research has focused mainly on the mechanisms by which cells use signalling to coordinate their activities, particularly in the context of embryonic development. Working closely with experimental biologists, he has studied spatio-temporal patterning in a range of animal and plant systems. Complementary to his work in mathematical biology, he has an interest in exploring how a philosophical approach in which process and flow are primary can be used as a framework for understanding living systems. |
|
11:00 - 11:30 |
Exploiting a structured environment: biochemical approaches for constructing information-harvesting devices
Organisms exploit correlations in their environment to survive and grow. This fact holds across scales, from bacterial chemotaxis, which leverages the spatial clustering of food molecules, to the loss of leaves by deciduous trees, which is worthwhile because sunlight exposure is highly correlated from day to day. At the most basic level, correlations within the environment that are not sustained by physical interactions indicate a non-equilibrium state that can be exploited via information processing. Here, it is demonstrated that relatively simple molecular systems can extract useful work from correlations ("feed on the information"). The use of concrete biochemical designs helps to demystify the thermodynamic role of information, and highlights the constraints under which information-exploiting devices must operate. Both free-running devices and those in which external manipulation (but, importantly, not feedback-control) is required are shown. Furthermore, competing strategies for exploiting a correlated environment are contrasted. These simple constructions illustrate a fundamental dilemma between exploring and exploiting a resource. ![]() Dr Thomas Ouldridge, Imperial College London, UK
![]() Dr Thomas Ouldridge, Imperial College London, UKIn October 2016 Dr Ouldridge took up a Royal Society University Research Fellowship in the Department of Bioengineering at Imperial College London, leading the Principles of Biomolecular Systems group. Dr Ouldridge has previously been affiliated with Nick Jones' systems and signals group at Imperial, the biochemical networks group of Pieter Rein ten Wolde in Amsterdam, and the Doye / Louis biophysics groups in Oxford (where they did their PhD). |
|
11:45 - 12:15 |
Programming DNA circuits
Biological organisms use complex molecular networks to navigate their environment and regulate their internal state. The development of synthetic systems with similar capabilities could lead to applications such as smart therapeutics or fabrication methods based on self-organization. To achieve this, molecular control circuits need to be engineered to perform integrated sensing, computation and actuation. In this talk, it will be demonstrated how DNA hybridization and strand displacement can be used to implement the computational core of such control circuits. To assist the design process, domain-specific programming languages have been developed to specify a sequence-level circuit design, and compile to chemical reaction networks, a well-established formalism for describing and simulating chemistry. Furthermore, parameter inference techniques have been embedded in this design platform, which facilitates design-build-test cycles via model-based characterization and circuit prediction. A first example will introduce the design and construction of a DNA implementation of the approximate majority algorithm, which seeks to establish consensus in a population of agents (molecules). A second example will illustrate how DNA circuits can be considerably accelerated by tethering DNA hairpin molecules to a fixed template, overcoming molecular diffusion as a rate-limiting step. ![]() Dr Neil Dalchau, Microsoft Research, UK
![]() Dr Neil Dalchau, Microsoft Research, UKNeil Dalchau is a Scientist at Microsoft Research Cambridge. He is interested in how biological systems process information, perform computations, and make life-preserving decisions. To directly test how biochemistry can achieve these tasks, he uses tools from dynamical systems theory and computer science to guide the construction of synthetic gene circuits in bacteria and DNA hybridization circuits in vitro. He is also seeking to understand how the adaptive immune system recognizes pathogens and disease states via the antigen presentation pathway. His undergraduate master’s degree from University of Oxford was in Mathematics, and he has a PhD in Plant Sciences from the University of Cambridge. He was the winner of the Tansley medal for plant sciences research in 2011. |
13:30 - 14:00 |
What time is it?
Even without looking at a watch, we have an inner feeling for time. How do we measure time? Our body, in particular each of our cells has an inner clock enabling all our rhythmic biological activities like sleeping. Astonishingly, prokaryotic cyanobacteria, which can divide faster than ones a day, also use an inner timing system to foresee the accompanying daily changes of light and temperature and regulate their physiology and behavior in 24-hour cycles. Their underlying biochemical oscillator fulfills all criteria of a true circadian clock though it is made of solely three proteins. It ticks with a robust 24-hour period even under fluctuating or continuous conditions. Nevertheless, it can be entrained by light, temperature or nutrients. Reconstituted from the purified protein components, KaiC, KaiB, and KaiA, the cyanobacterial protein clock can tick autonomously in a test tube for weeks. This apparent simplicity has proven to be an ideal system for answering questions about information transfer and robustness of circadian clocks. ![]() Professor Dr Ilka Maria Axmann, Heinrich-Heine-Universität Düsseldorf, Germany
![]() Professor Dr Ilka Maria Axmann, Heinrich-Heine-Universität Düsseldorf, GermanyIlka Maria Axmann is Junior-Professor and Head of the Institute for Synthetic Microbiology at Heinrich-Heine-University Düsseldorf, Coordinator of the EU project RiboNets and a mother of two children. Her background is in Molecular Biology where she earned her doctoral degree at Humboldt-University Berlin, and Biotechnology, which she studied during her Diploma. Her research focuses on molecular regulatory processes in microorganisms influenced by internal factors like small RNA molecules and the circadian clock. Particular focus is placed on the engineering of cyanobacteria as a future host for sustainable biotechnology. |
|
---|---|---|
14:15 - 14:45 |
Dynamics, Feedback, and Noise in Natural and Synthetic Gene Regulatory Networks
Cells live in uncertain, dynamic environments and have many feedback mechanisms for sensing and responding to changes in their surroundings. This talk will discuss examples of both natural and engineered feedback circuits and how they can be used to alter dynamics of gene expression. Using a combination of time-lapse microscopy experiments and stochastic modeling, the talk will show how E. coli bacteria use feedback to generate dynamics and noise in expression of a key regulatory protein, providing transient antibiotic resistance at the single-cell level. In addition, it will highlight diverse examples of how feedback can be used to allow cells to responds to changing environments. ![]() Professor Mary Dunlop, Boston University, USA
![]() Professor Mary Dunlop, Boston University, USAMary Dunlop is an Assistant Professor in the Biomedical Engineering Department at Boston University. She graduated from Princeton University with a B.S.E. in Mechanical and Aerospace Engineering and a minor in Computer Science. She then received her Ph.D. from the California Institute of Technology, where she studied synthetic biology with a focus on dynamics and feedback in gene regulation. As a postdoctoral scholar, she conducted research on biofuel production at the Department of Energy's Joint BioEnergy Institute. Her lab engineers novel synthetic feedback control systems and also studies naturally occurring examples of feedback in gene regulation. In recognition of her outstanding research and service contributions, she has received many honors including a Department of Energy Early Career Award, a National Science Foundation CAREER Award, and the ACS Synthetic Biology Young Investigator Award. |
|
15:30 - 16:00 |
Computing with motile biological agents exploring networks
Many mathematical problems, e.g., cryptography, network routing, require the exploration a large number of candidate solutions. Because the time required for solving these problems grows exponentially with their size, electronic computers, which operate sequentially, cannot solve them in a reasonable time. In contrast, biological organisms routinely process information in parallel for essential tasks, e.g., foraging, searching for space opens three possible biocomputing avenues. Biomimetic algorithms translate biological procedures, e.g., space searching, chemotaxis, etc., into mathematical algorithms. This approach was used to derive fungi-inspired algorithms for searching space and bacterial chemotaxis-inspired algorithms for finding the edges of geometrical patterns. Biosimulation uses the procedures of large numbers of motile biological agents, directly, without any translation to formal mathematical algorithms, thus by-passing computation-proper. The agents explore complex networks that mimic real situations, e.g., traffic. This approach focused almost entirely on traffic optimization, using an amoeboid organisms placed in confined geometries, with chemotactic ‘cues’, e.g., nutrients in node coordinates. Computing with biological agents in networks uses very large number of agents exploring microfluidics networks, purposefully designed to encode hard mathematical problems. The foundations of a parallel-computation system in which a combinatorial problem (SUBSET SUM) is encoded into a graphical, modular network embedded in a nanofabricated planar device was reported. Exploring the network in a parallel fashion using a large number of independent agents, e.g., molecular motor-propelled cytoskeleton filaments, bacteria, algae, solves the mathematical problem. This device uses orders of magnitude less energy than conventional computers, additionally addressing issues related to parallel computing implementation. ![]() Professor Dan V. Nicolau, McGill University, Canada
![]() Professor Dan V. Nicolau, McGill University, CanadaDan V. Nicolau is Maria Zeleka Roy Chair of Bioengineering; and the first Chair of the Department of Bioengineering at McGill University. Prof. Nicolau has a PhD in Chemical Engineering, a MS in Cybernetics, Informatics & Statistics and a MEng in Polymer Science & Engineering. He has published more than 100 papers in peer-reviewed scientific journals, a similar number of full papers in conference proceedings and 6 book chapters. He has edited one book (with U. Muller; on microarray technology and applications), and edited or co-edited the proceedings of more than 30 international conferences. Prof. Nicolau is a Fellow of the International Society of Optical Engineering (SPIE). His present research aggregates around three themes: (i) intelligent-like behaviour of microorganisms in confined spaces, which manifests in the process of survival and growth; (ii) dynamic micro/nanodevices, such as microfluidics/lab-on-a-chip, and devices based on protein molecular motors, with applications in diagnosis, drug discovery and biocomputation; and (iii) micro/nano-structured surfaces for biomedical microdevices. |
|
16:15 - 17:00 | Panel discussion |