Links to external sources may no longer work as intended. The content may not represent the latest thinking in this area or the Society’s current position on the topic.
Numerical algorithms for high-performance computational science
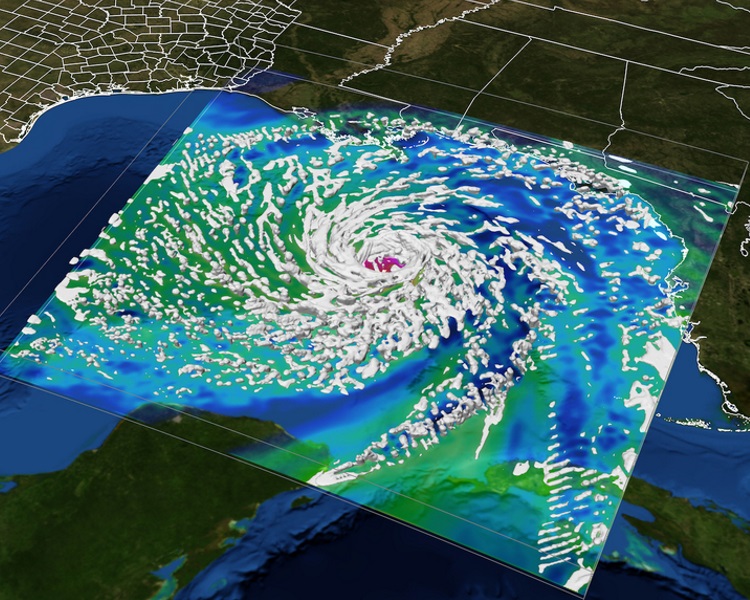
Scientific discussion meeting organised by Professor Nicholas Higham FRS, Laura Grigori and Professor Jack Dongarra.
As computer architectures evolve, numerical algorithms for high-performance computing struggle to cope with the high resolution and data intensive methods that are now key to many research fields. This meeting brought together computer and computational scientists who are developing innovative scalable algorithms and software with application scientists who need to explore and adopt the new algorithms in order to achieve peta/exascale performance.
Speaker abstracts and biographies can be found below. Recorded audio of the presentations is also available below. An accompanying journal issue for this meeting was published in Philosophical Transactions of the Royal Society A.
Attending this event
This meeting has taken place.
Enquiries: contact the Scientific Programmes team
Organisers
Schedule
Chair
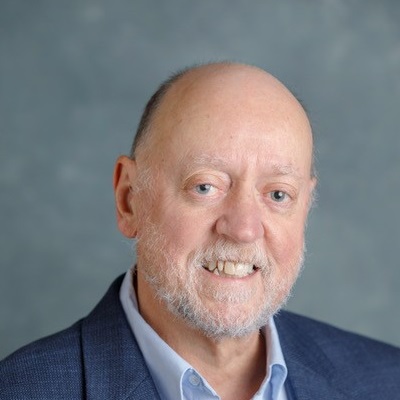
Professor Jack Dongarra, University of Tennessee, USA and University of Manchester, UK
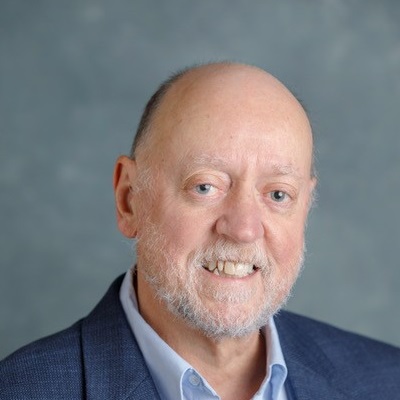
Professor Jack Dongarra, University of Tennessee, USA and University of Manchester, UK
Jack Dongarra holds an appointment at the University of Tennessee, Oak Ridge National Laboratory, and the University of Manchester. He specialises in numerical algorithms in linear algebra, parallel computing, use of advanced-computer architectures, programming methodology, and tools for parallel computers. He was awarded the IEEE Sid Fernbach Award in 2004; in 2008 he was the recipient of the first IEEE Medal of Excellence in Scalable Computing; in 2010 he was the first recipient of the SIAM Special Interest Group on Supercomputing's award for Career Achievement; in 2011 he was the recipient of the IEEE Charles Babbage Award; and in 2013 he received the ACM/IEEE Ken Kennedy Award. He is a Fellow of the AAAS, ACM, IEEE, and SIAM and a foreign member of the Russian Academy of Science and a member of the US National Academy of Engineering.
08:15 - 08:45 |
Hierarchical algorithms on hierarchical architectures
![]() Professor David E Keyes, King Abdullah University of Science and Technology, Saudi Arabia
![]() Professor David E Keyes, King Abdullah University of Science and Technology, Saudi ArabiaDavid Keyes directs the Extreme Computing Research Center at KAUST. He works at the interface between parallel computing and the numerical analysis of PDEs, with a focus on scalable implicit solvers. Newton-Krylov-Schwarz (NKS) and Additive Schwarz Preconditioned Inexact Newton (ASPIN) are methods he helped name and is helping to popularise. Before joining KAUST as a founding dean in 2009, he led multi-institutional scalable solver software projects in the SciDAC and ASCI programs of the US DOE, ran university collaboration programs at LLNL’s ISCR and NASA’s ICASE, and taught at Columbia, Old Dominion, and Yale Universities. He is a Fellow of SIAM, AMS, and AAAS, and has been awarded the ACM Gordon Bell Prize, the IEEE Sidney Fernbach Award, and the SIAM Prize for Distinguished Service to the Profession. He earned a BSE in Aerospace and Mechanical Sciences from Princeton in 1978 and a PhD in Applied Mathematics from Harvard in 1984. |
|
---|---|---|
08:55 - 09:25 |
Antisocial parallelism: avoiding, hiding and managing communication
![]() Professor Katherine Yelick, UC Berkeley and Lawrence Berkeley National Laboratory, USA
![]() Professor Katherine Yelick, UC Berkeley and Lawrence Berkeley National Laboratory, USAKatherine Yelick is a Professor of Electrical Engineering and Computer Sciences at the University of California at Berkeley and the Associate Laboratory Director for Computing Sciences at Lawrence Berkeley National Laboratory. She is known for her research in parallel languages, compilers, algorithms, libraries, and scientific applications. She is the former director of the National Energy Research Scientific Computing Center (NERSC), and currently oversees NERSC, ESnet, and the and the Computational Research Division. Yelick has served on the Computer Science Telecommunications Board (CSTB) and the Computing Community Consortium (CCC). She is a Fellow of ACM and AAAS, and recipient of the ACM-W Athena award and ACM/IEEE Ken Kennedy Award. She is also a member of the National Academy of Engineering and the American Academy of Arts and Sciences. |
|
09:00 - 09:15 | Introduction | |
09:45 - 09:55 | Discussion | |
10:05 - 10:35 |
Big telescope, big data: towards exa-scale with the SKA
The Square Kilometre Array (SKA) will be the world’s largest radio telescope. The pre-construction design effort for the SKA started in 2012 and involves approximately 100 organisations in 20 countries. The scale of the project makes it a huge endeavour, not only in terms of the science that it will ultimately undertake, but also in terms of the engineering and development that is required to design and build such a unique instrument. This design effort is now drawing to a close and early science operations are anticipated to begin in ~2025. With raw data rates capable of producing zetta-byte volumes per year, the online compute and data compression for the telescope is a key component of observatory operations. Expected to run in soft real-time, this processing will require a significant fraction of an exa-flop when at full capacity and will reduce the data rate from the observatory to the outside world to a few hundred peta-bytes of data products per year. Astronomers will receive their data through a network of worldwide SKA Regional Centres (SRCs). Unlike the observatory science data processing, it is not possible to define a finite set of compute models for an SRC. An SKA Regional Centre requires the flexibility to enable a broad range of single user development and processing, as well as providing an infrastructure that can support efficient large-scale compute for the standardised data processing of reserved access key science projects. Here Professor Scaife will discuss the processing model for the SKA telescope, from the antennas to the regional centres, and highlight the components of this processing that dominate the compute load. She will discuss the details of specific algorithms being used and approaches that have been adopted to improve efficiency, both in terms of optimisation and re-factoring. ![]() Professor Anna Scaife, University of Manchester, UK
![]() Professor Anna Scaife, University of Manchester, UKAnna Scaife (MPhys Bristol, PhD Cambridge) is Professor of Radio Astronomy at the University of Manchester, where she is head of the Jodrell Bank Interferometry Centre of Excellence, and holds the 2017 Blaauw Chair in Astrophysics at the University of Groningen. She is the recipient of a European Research Council Fellowship for her research group's work investigating the origin and evolution of large-scale cosmic magnetic fields, and leads a number of projects in technical radio astronomy development and scientific computing as part of the Square Kilometre Array project. As well as scientific research, Anna runs two Newton Fund training programs that provide bursaries for students from Southern Africa and Latin America to pursue graduate degrees in the UK focusing on Big Data and data intensive science. In 2014, Anna was honoured by the World Economic Forum as one of thirty scientists under the age of 40 selected for their contributions to advancing the frontiers of science, engineering or technology in areas of high societal impact. |
|
10:25 - 10:35 | Discussion | |
10:35 - 11:05 | Coffee | |
10:45 - 11:15 |
Algorithms for in situ data analytics in next generation molecular dynamics workflows
Molecular dynamics (MD) simulations studying the classical time evolution of a molecular system at atomic resolution are widely recognized in the fields of chemistry, material sciences, molecular biology, and drug design; these simulations are one of the most common simulations on supercomputers. Next-generation supercomputers will have dramatically higher performance than do current systems, generating more data that needs to be analyzed (ie in terms of number and length of MD trajectories). The coordination of data generation and analysis cannot rely on manual, centralized approaches as it is predominately done today. In this talk Dr Taufer will discuss how the combination of machine learning and data analytics algorithms, workflow management methods, and high performance computing systems can transition the runtime analysis of larger and larger MD trajectories towards the exascale era. Dr Taufer will demonstrate her group's approach on three case studies: protein-ligand docking simulations, protein folding simulations, and analytics of protein functions depending on proteins’ three-dimensional structures. She will show how, by mapping individual substructures to metadata, frame by frame at runtime, it is possible to study the conformational dynamics of proteins in situ. The ensemble of metadata can be used for automatic, strategic analysis and steering of MD simulations within a trajectory or across trajectories, without manually identify those portions of trajectories in which rare events take place or critical conformational features are embedded. ![]() Dr Michela Taufer, The University of Tennessee Knoxville, USA
![]() Dr Michela Taufer, The University of Tennessee Knoxville, USAMichela Taufer holds the Jack Dongarra Professorship in High Performance Computing in the Department of Electrical Engineering and Computer Science at the University of Tennessee Knoxville. She earned her undergraduate degrees in Computer Engineering from the University of Padova, Italy and her doctoral degree in Computer Science from the Swiss Federal Institute of Technology or ETH, Switzerland. From 2003 to 2004 she was a La Jolla Interfaces in Science Training Program (LJIS) Postdoctoral Fellow at the University of California San Diego (UCSD) and The Scripps Research Institute (TSRI), where she worked on interdisciplinary projects in computer systems and computational chemistry. Taufer’s research interests in high performance computing include scientific applications, scheduling and reproducibility challenges, and big data analytics. She is currently serving on the NSF Advisory Committee for Cyberinfrastructures (ACCI). She is a professional member of the IEEE and a Distinguished Scientist of the ACM. |
|
11:35 - 11:45 | Discussion | |
12:15 - 12:25 | Discussion |
Chair
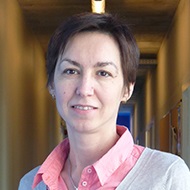
Laura Grigori, INRIA Paris, France
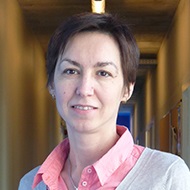
Laura Grigori, INRIA Paris, France
Laura Grigori is a senior research scientist at INRIA in France, where she leads the Alpines group, a joint group between INRIA and the J.L. Lions Laboratory, Sorbonne University, in Paris. Her field of expertise is in numerical linear algebra and high performance scientific computing. She co-authored 2008 papers introducing communication avoiding algorithms that provably minimise communication, for which she was awarded, with her co-authors, the SIAM Siag on Supercomputing Best Paper Prize 2016 for the most outstanding paper published in 2012–2015 in a journal in the field of high performance computing. She leads several projects on preconditioning, communication avoiding algorithms and associated numerical libraries for large scale parallel/multicore machines. She was the co-chair of the Algorithms Area, Supercomputing 2013 conference and the Program Director of the SIAM special interest group on supercomputing from January 2014 to December 2015, then the chair from January 2016 to December 2017. She has been a member of the PRACE Scientific Steering Committee since 2016 and a member of SIAM Council since January 2018.
12:25 - 12:55 |
Numerical computing challenges for large-scale deep learning
In this talk Professor Stevens will discuss the convergence of traditional high-performance computing, data analytics and deep learning and some of the architectural, algorithmic and software challenges this convergence creates as we push the envelope on the scale and volume of training and inference runs on todays' largest machines. Deep learning is beginning to have significant impact in science, engineering and medicine. The use of HPC platforms in deep learning ranges from training single models at high speed, training large number of models in sweeps for model development and model discovery, hyper-parameter optimisation, and uncertainty quantification as well as large-scale ensembles for data preparation, inferencing on large-scale data and for data post-processing. Already it has been demonstrated that some reinforcement learning problems need multiple exaops/s days of computing to reach state-of-the-art performance. This need for more performance is driving the development of architectures aimed at accelerating deep learning training and inference beyond the already high-performance of GPUs. These new 'AI' architectures are often optimised for common cases in deep learning, typically deep convolutional networks and variations of half-precision floating point. Professor Stevens will review some of these new accelerator design points, the approaches to acceleration and scalability, and discuss some of the driver science problems in deep learning. ![]() Professor Rick Stevens, Argonne National Laboratory and University of Chicago, USA
![]() Professor Rick Stevens, Argonne National Laboratory and University of Chicago, USAProfessor Rick Stevens is internationally known for work in high-performance computing, collaboration and visualisation technology, and for building computational tools and web infrastructures to support large-scale genome and metagenome analysis for basic science and infectious disease research. He is the principle investigator for the NIH-NIAID funded PATRIC Bioinformatics Resource Center which is developing comparative analysis tools for infectious disease research, and for the Exascale Computing Project (ECP) Exascale Deep Learning and Simulation Enabled Precision Medicine for Cancer project which focuses on building a scalable deep neural network code called the CANcer Distributed Learning Environment (CANDLE) to address three top challenges of the National Cancer Institute. Stevens has been a professor at the University of Chicago since 1999, and Associate Laboratory Director at Argonne National Laboratory since 2004. Over the past twenty years, he and his colleagues have developed the SEED, RAST, MG-RAST and ModelSEED genome analysis and bacterial modelling servers that have been used by tens of thousands of users to annotate and analyse more than 250,000 microbial genomes and metagenomic samples. He teaches and supervises students in the areas of computer systems and computational biology, and he co-leads the DOE national laboratory group that has been developing the national initiative for exascale computing. |
|
---|---|---|
13:05 - 13:35 |
High-performance sampling of determinantal point processes
Despite having been introduced for sampling eigenvalue distributions of ensembles of random matrices, Determinantal Point Processes (DPPs) have recently been popularised due to their usage in encouraging diversity in recommender systems. Traditional sampling schemes have used dense Hermitian eigensolvers to reduce sampling to an equivalent of a low-rank diagonally-pivoted Cholesky factorization, but researchers are starting to understand deeper connections to Cholesky that avoid the need for spectral decompositions. This talk begins with a proof that one can sample a DPP via a trivial tweak of an LDL factorization that flips a Bernoulli coin weighted by each nominal pivot: simply keep an item if the coin lands on heads, or decrement the diagonal entry by one otherwise. The fundamental mechanism is that Schur complement elimination of variables in a DPP kernel matrix generates the kernel matrix of the conditional distribution if said variables are known to be in the sample. While researchers have begun connecting DPP sampling and Cholesky factorization to avoid expensive dense spectral decompositions, high-performance implementations have yet to be explored, even in the dense regime. The primary contributions of this talk (other than the aforementioned theorem) are side-by-side implementations and performance results of high-performance dense and sparse-direct DAG-scheduled DPP sampling and LDL factorizations. The software is permissively open sourced as part of the catamari project at gitlab.com/hodge_star/catamari. ![]() Dr Jack Poulson
![]() Dr Jack PoulsonJack Poulson is a computational scientist with interests spanning numerical linear algebra, mathematical optimisation, lattice reduction, statistical inference, and differential geometry. As a graduate student at The University of Texas at Austin, he created a hierarchy of open source libraries for distributed-memory linear algebra that culminated in his doctorate on fast solvers for frequency-domain anisotropic wave equations. His subsequent academic career involved serving as a Postdoctoral Scholar at Stanford University, an Assistant Professor of Computational Science and Engineering at The Georgia Institute of Technology, and an Assistant Professor of Mathematics at Stanford University. He then spent several years developing production machine learning systems as a Senior Research Scientist at Google and is now the owner of a small scientific computing company. |
|
13:55 - 14:05 | Discussion | |
14:10 - 14:40 |
Computing beyond the end of Moore’s Law
Moore’s Law is a techno-economic model that has enabled the Information Technology (IT) industry to nearly double the performance and functionality of digital electronics roughly every two years within a fixed cost, power and area. Within a decade, the technological underpinnings for the process Gordon Moore described will come to an end as lithography gets down to atomic scale. This talk provides an updated view of what a 2021-2023 system might look like and the challenges ahead, based on our most recent understanding of technology roadmaps. It also will discuss the tapering of historical improvements in lithography, and how it affects options available to continue scaling of successors to the first exascale machine. ![]() Mr John Shalf, Lawrence Berkeley National Laboratory, USA
![]() Mr John Shalf, Lawrence Berkeley National Laboratory, USAJohn Shalf is Department Head for Computer Science at Lawrence Berkeley National Laboratory, and recently was deputy director of Hardware Technology for the DOE Exascale Computing Project. Shalf is a co-author of over 60 publications in the field of parallel computing software and HPC technology, including three best papers and the widely cited report The Landscape of Parallel Computing Research: A View from Berkeley (with David Patterson and others), as well as ExaScale Software Study: Software Challenges in Extreme Scale Systems, which sets the Defense Advanced Research Project Agency's (DARPA's) information technology research investment strategy for the next decade. |
|
14:35 - 14:45 | Discussion | |
14:45 - 15:10 | Coffee | |
14:50 - 15:20 |
Exascale applications: skin in the game
As noted in Wikipedia, skin in the game refers to having “incurred risk by being involved in achieving a goal”, where “skin is a synecdoche for the person involved, and game is the metaphor for actions on the field of play under discussion”. For exascale applications under development in the U.S Department of Energy (DOE) Exascale Computing Project (ECP), nothing could be more apt, with the skin being exascale applications and the game being delivering comprehensive science-based computational applications that effectively exploit exascale HPC technologies to provide breakthrough modelling and simulation and data science solutions. These solutions must yield high-confidence insights and answers to our nation’s most critical problems and challenges in scientific discovery, energy assurance, economic competitiveness, health enhancement, and national security. Exascale applications (and their companion co-designed computational motifs) are a foundational element of the ECP and are the vehicle for delivery of consequential solutions and insight from exascale systems. The breadth of these applications runs the gamut: chemistry and materials; energy production and transmission; earth and space science; data analytics and optimisation; and national security. Each ECP application is focused on targeted development to address a unique mission challenge problem, ie, one that possesses solution amenable to simulation insight, represents a strategic problem important to a DOE mission program, and is currently intractable without the computational power of exascale. Any tangible progress requires close coordination with exascale application, algorithm, and software development to adequately address six key application development challenges: porting to accelerator-based architectures; exposing additional parallelism; coupling codes to create new multi-physics capability; adopting new mathematical approaches; algorithmic or model improvements; and leveraging optimised libraries. Each ECP application possesses a unique development plan base on its requirements-based combination of physical model enhancements and additions, algorithm innovations and improvements, and software architecture design and implementation. Illustrative examples of these development activities will be given along with results achieved to date on existing DOE supercomputers such as the Summit system at Oak Ridge National Laboratory. ![]() Dr Douglas B Kothe, Oak Ridge National Laboratory, USA
![]() Dr Douglas B Kothe, Oak Ridge National Laboratory, USADouglas B Kothe (Doug) has over three decades of experience in conducting and leading applied R&D in computational science applications designed to simulate complex physical phenomena in the energy, defence, and manufacturing sectors. Doug is currently the Director of the U.S. Department of Energy Exascale Computing Project. Prior to that, he was Deputy Associate Laboratory Director of the Computing and Computational Sciences Directorate at Oak Ridge National Laboratory (ORNL). Other prior positions for Doug at ORNL, where he has been since 2006, include Director of the Consortium for Advanced Simulation of Light Water Reactors, DOE’s first Energy Innovation Hub (2010–2015), and Director of Science at the National Center for Computational Sciences (2006–2010). Before coming to ORNL, Doug spent 20 years at Los Alamos National Laboratory, where he held a number of technical and line and program management positions, with a common theme being the development and application of modelling and simulation technologies targeting multi-physics phenomena characterised in part by the presence of compressible or incompressible interfacial fluid flow. Doug also spent one year at Lawrence Livermore National Laboratory in the late 1980s as a physicist in defence sciences. Doug holds a Bachelor in Science in Chemical Engineering from the University of Missouri – Columbia (1983) and a Masters in Science (1986) and Doctor of Philosophy (1987) in Nuclear Engineering from Purdue University. |
|
15:30 - 16:00 | Poster flash talks | |
15:40 - 15:50 | Discussion | |
16:20 - 16:30 | Discussion | |
17:00 - 18:00 | Poster session |
Chair
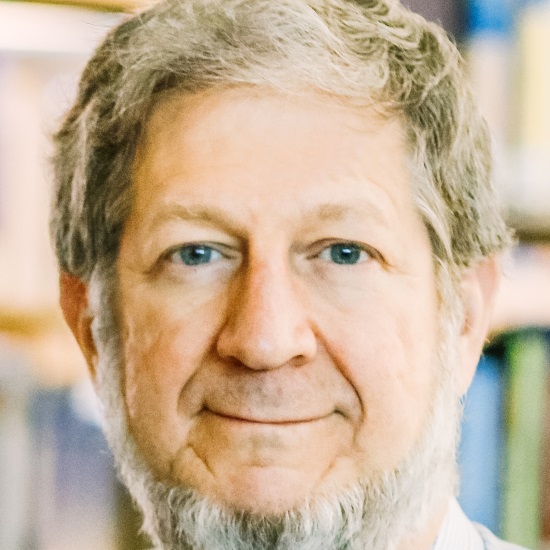
Professor David E Keyes, King Abdullah University of Science and Technology, Saudi Arabia
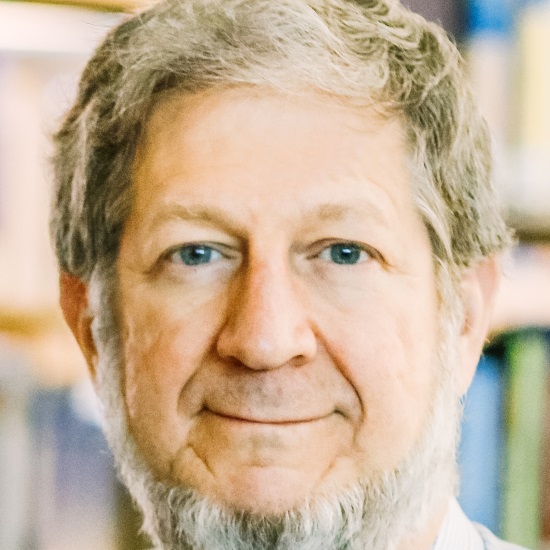
Professor David E Keyes, King Abdullah University of Science and Technology, Saudi Arabia
David Keyes directs the Extreme Computing Research Center at KAUST. He works at the interface between parallel computing and the numerical analysis of PDEs, with a focus on scalable implicit solvers. Newton-Krylov-Schwarz (NKS) and Additive Schwarz Preconditioned Inexact Newton (ASPIN) are methods he helped name and is helping to popularise. Before joining KAUST as a founding dean in 2009, he led multi-institutional scalable solver software projects in the SciDAC and ASCI programs of the US DOE, ran university collaboration programs at LLNL’s ISCR and NASA’s ICASE, and taught at Columbia, Old Dominion, and Yale Universities. He is a Fellow of SIAM, AMS, and AAAS, and has been awarded the ACM Gordon Bell Prize, the IEEE Sidney Fernbach Award, and the SIAM Prize for Distinguished Service to the Profession. He earned a BSE in Aerospace and Mechanical Sciences from Princeton in 1978 and a PhD in Applied Mathematics from Harvard in 1984.
08:00 - 08:30 |
Machine learning and big scientific data
There is now broad recognition within the scientific community that the ongoing deluge of scientific data is fundamentally transforming academic research. Turing Award winner Jim Gray referred to this revolution as 'The Fourth Paradigm: Data Intensive Scientific Discovery'. Researchers now need new tools and technologies to manipulate, analyse, visualise, and manage the vast amounts of research data being generated at the national large-scale experimental facilities. In particular, machine learning technologies are fast becoming a pivotal and indispensable component in modern science, from powering discovery of modern materials to helping us handling large-scale imagery data from microscopes and satellites. Despite these advances, the science community lacks a methodical way of assessing and quantifying different machine learning ecosystems applied to data-intensive scientific applications. There has so far been little effort to construct a coherent, inclusive and easy to use benchmark suite targeted at the ‘scientific machine learning’ (SciML) community. Such a suite would enable an assessment of the performance of different machine learning models, applied to a range of scientific applications running on different hardware architectures – such as GPUs and TPUs – and using different machine learning frameworks such as PyTorch and TensorFlow. In this paper, Professor Hey will outline his approach for constructing such a 'SciML benchmark suite' that covers multiple scientific domains and different machine learning challenges. The output of the benchmarks will cover a number of metrics, not only the runtime performance, but also metrics such as energy usage, and training and inference performance. Professor Hey will present some initial results for some of these SciML benchmarks. ![]() Professor Tony Hey CBE FREng, Science and Technology Facilities Council, UKRI, UK
![]() Professor Tony Hey CBE FREng, Science and Technology Facilities Council, UKRI, UKTony Hey began his career as a theoretical physicist with a doctorate in particle physics from the University of Oxford in the UK. After a career in physics that included research positions at Caltech and CERN, and a professorship at the University of Southampton in England, he became interested in parallel computing and moved into computer science. In the 1980s he was one of the pioneers of distributed memory message-passing computing and co-wrote the first draft of the successful MPI message-passing standard. After being both Head of Department and Dean of Engineering at Southampton, Tony Hey was appointed to lead the UK’s ground-breaking ‘eScience’ initiative in 2001. He recognised the importance of Big Data for science and wrote one of the first papers on the ‘Data Deluge’ in 2003. He joined Microsoft in 2005 as a Vice President and was responsible for Microsoft’s global university research engagements. He worked with Jim Gray and his multidisciplinary eScience research group and edited a tribute to Jim called The Fourth Paradigm: Data-Intensive Scientific Discovery. Hey left Microsoft in 2014 and spent a year as a Senior Data Science Fellow at the eScience Institute at the University of Washington. He returned to the UK in November 2015 and is now Chief Data Scientist at the Science and Technology Facilities Council. In 1987 Tony Hey was asked by Caltech Nobel physicist Richard Feynman to write up his ‘Lectures on Computation’. This covered such unconventional topics as the thermodynamics of computing as well as an outline for a quantum computer. Feynman’s introduction to the workings of a computer in terms of the actions of a ‘dumb file clerk’ was the inspiration for Tony Hey’s attempt to write The Computing Universe, a popular book about computer science. Tony Hey is a fellow of the AAAS and of the UK's Royal Academy of Engineering. In 2005, he was awarded a CBE by Prince Charles for his ‘services to science.’ |
|
---|---|---|
08:40 - 09:10 |
Iterative linear algebra in the exascale era
Iterative methods for solving linear algebra problems are ubiquitous throughout scientific and data analysis applications and are often the most expensive computations in large-scale codes. Approaches to improving performance often involve algorithm modification to reduce data movement or the selective use of lower precision in computationally expensive parts. Such modifications can, however, result in drastically different numerical behavior in terms of convergence rate and accuracy due to finite precision errors. A clear, thorough understanding of how inexact computations affect numerical behaviour is thus imperative in balancing tradeoffs in practical settings. In this talk, Dr Carson focuses on two general classes of iterative methods: Krylov subspace methods and iterative refinement. She presents bounds on attainable accuracy and convergence rate in finite precision variants of Krylov subspace methods designed for high performance and show how these bounds lead to adaptive approaches that are both efficient and accurate. Then, motivated by recent trends in multiprecision hardware, she presents new forward and backward error bounds for general iterative refinement using three precisions. The analysis suggests that if half precision is implemented efficiently, it is possible to solve certain linear systems up to twice as fast and to greater accuracy. As we push toward exascale level computing and beyond, designing efficient, accurate algorithms for emerging architectures and applications is of utmost importance. Dr Carson finishes by discussing extensions in new applications areas and the broader challenge of understanding what increasingly large problem sizes will mean for finite precision computation both in theory and practice. ![]() Dr Erin Carson, Charles University, Czech Republic
![]() Dr Erin Carson, Charles University, Czech RepublicErin Carson is a research fellow in the Department of Numerical Mathematics at Charles University in Prague. She received her PhD at the University of California, Berkeley in 2015, supported by a National Defense Science and Engineering Graduate Fellowship, and spent the following three years as a Courant Instructor/Assistant Professor at the Courant Institute of Mathematical Sciences at New York University. Her research interests lie at the intersection of high-performance computing, numerical linear algebra, and parallel algorithms, with a focus on analysing tradeoffs between accuracy and performance in algorithms for large-scale sparse linear algebra. |
|
09:30 - 09:40 | Discussion | |
09:50 - 10:20 |
Stochastic rounding and reduced-precision fixed-point arithmetic for solving neural ODEs
There is increasing interest from users of large-scale computation in smaller and/or simpler arithmetic types. The main reasons for this are energy efficiency and memory footprint/bandwidth. However, the outcome of a simple replacement with lower precision types is increased numerical errors in the results. The practical effects of this range from unimportant to intolerable. Professor Furber will describe some approaches for reducing the errors of lower-precision fixed-point types and arithmetic relative to IEEE double-precision floating-point. He will give detailed examples in an important domain for numerical computation in neuroscience simulations: the solution of Ordinary Differential Equations (ODEs). He will look at two common model types and demonstrate that rounding has an important role in producing improved precision of spike timing from explicit ODE solution algorithms. In particular the group finds that stochastic rounding consistently provides a smaller error magnitude - in some cases by a large margin - compared to single-precision floating-point and fixed-point with rounding-to-nearest across a range of Izhikevich neuron types and ODE solver algorithms. We also consider simpler and computationally much cheaper alternatives to full stochastic rounding of all operations, inspired by the concept of 'dither' that is a widely understood mechanism for providing resolution below the LSB in digital audio, image and video processing. Professor Furber will discuss where these alternatives are are likely to work well and suggest a hypothesis for the cases where they will not be as effective. These results will have implications for the solution of ODEs in all subject areas, and should also be directly relevant to the huge range of practical problems that are modelled as Partial Differential Equations (PDEs). ![]() Professor Steve Furber CBE FREng FRS, The University of Manchester, UK
![]() Professor Steve Furber CBE FREng FRS, The University of Manchester, UKSteve Furber CBE FREng FRS is ICL Professor of Computer Engineering in the School of Computer Science at the University of Manchester, UK. After completing a BA in mathematics and a PhD in aerodynamics at the University of Cambridge, UK, he spent the 1980s at Acorn Computers, where he was a principal designer of the BBC Microcomputer and the ARM 32-bit RISC microprocessor. Over 120 billion variants of the ARM processor have since been manufactured, powering much of the world's mobile and embedded computing. He moved to the ICL Chair at Manchester in 1990 where he leads research into asynchronous and low-power systems and, more recently, neural systems engineering, where the SpiNNaker project has delivered a computer incorporating a million ARM processors optimised for brain modelling applications. |
|
10:10 - 10:20 | Discussion | |
10:20 - 10:50 | Coffee | |
10:30 - 11:00 |
Rethinking deep learning: architectures and algorithms
Professor Constantinides will consider the problem of efficient inference using deep neural networks. Deep neural networks are currently a key driver for innovation in both numerical algorithms and architecture, and are likely to form an important workload for computational science in the future. While algorithms and architectures for these computations are often developed independently, this talk will argue for a holistic approach. In particular, the notion of application efficiency needs careful analysis in the context of new architectures. Given the importance of specialised architectures in the future, Professor Constantinides will focus on custom neural network accelerator design. He will define a notion of computation general enough to encompass both the typical design specification of a computation performed by a deep neural network and its hardware implementation. This will allow us to explore - and make precise - some of the links between neural network design methods and hardware design methods. Bridging the gap between specification and implementation requires us to grapple with questions of approximation, which he will formalise and explore opportunities to exploit. This will raise questions about the appropriate network topologies, finite precision data-types, and design/compilation processes for such architectures and algorithms, and how these concepts combine to produce efficient inference engines. Some new results will be presented on this topic, providing partial answers to these questions, and we will explore fruitful avenues for future research. ![]() Professor George Anthony Constantinides, Imperial College London, UK
![]() Professor George Anthony Constantinides, Imperial College London, UKProfessor George Constantinides holds the Royal Academy of Engineering / Imagination Technologies Research Chair in Digital Computation at Imperial College London, where he leads the Circuits and Systems Group. His research is focused on efficient computational architectures and their link to software through High Level Synthesis, in particular automated customisation of memory systems and computer arithmetic. He is a programme and steering committee member of the ACM International Symposium on FPGAs, a programme committee member of the IEEE International Symposium on Computer Arithmetic, and Associate Editor of IEEE Transactions on Computers. |
|
11:10 - 11:40 |
Reduced numerical precision and imprecise computing for ultra-accurate next-generation weather and climate models
Developing reliable weather and climate models must surely rank amongst the most important tasks to help society become resilient to the changing nature of weather and climate extremes. With such models we can determine the type of infrastructure investment needed to protect against the worst weather extremes, and can prepare for specific extremes well in advance of their occurrence. Weather and climate models are based on numerical representations of the underlying nonlinear partial differential equations of fluid flow. However, they do not conform to the usual paradigm in numerical analysis: you give me a problem you want solved to a certain accuracy and I will give you an algorithm to achieve this. In particular, with current supercomputers, we are unable to solve the underpinning equations to the accuracy we would like. As a result, models exhibit pervasive biases against observations. These biases can be as large as the signals we are trying to simulate or predict. For climate modelling the numerical paradigm instead becomes this: for a given computational resource, what algorithms can produce the most accurate weather and climate simulations? Professor Palmer argues for an oxymoron: that to maximise accuracy we need to abandon the use of 64-bit precision where it is not warranted. Examples are given of the use of 16-bit numerics for large parts of the model code. The computational savings so made can be reinvested to increase model resolution and thereby increase model accuracy. In assessing the relative degradation of model performance at fixed resolution with reduced-precision numerics, the group utilises the notion of stochastic parametrisation as a representation of model uncertainty. Such stochasticity can itself reduce model systematic error. The benefit of stochasticity suggests a role for low-energy non-deterministic chips in future HPC. ![]() Professor Tim Palmer CBE FRS, University of Oxford, UK
![]() Professor Tim Palmer CBE FRS, University of Oxford, UKTim Palmer is a Royal Society Research Professor in the Department of Physics at the University of Oxford. Prior to that he was Head of Division at the European Centre for Medium-Range Weather Forecasts where he pioneered the development of ensemble prediction techniques, allowing weather and climate predictions to be expressed in flow-dependent probabilistic terms. Such developments have included the reformulation of sub-grid parametrisations as stochastic rather than deterministic schemes. Over the last 10 years, Tim has been vocal in advocating for much greater dedicated computing capability for climate prediction than is currently available. He has won the top awards of the European and Meteorological Societies, the Dirac Gold Medal of the Institute of Physics, and is a Foreign or Honorary Member of a number of learned societies around the world. |
|
11:20 - 11:30 | Discussion | |
12:00 - 12:10 | Discussion | |
12:40 - 12:50 | Discussion |
Chair
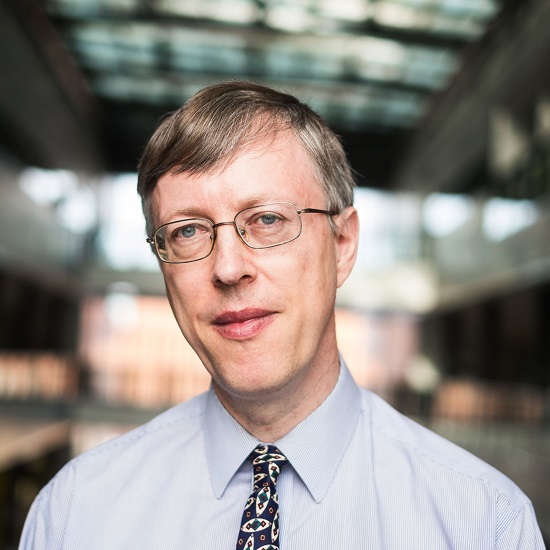
Professor Nicholas Higham FRS, University of Manchester, UK
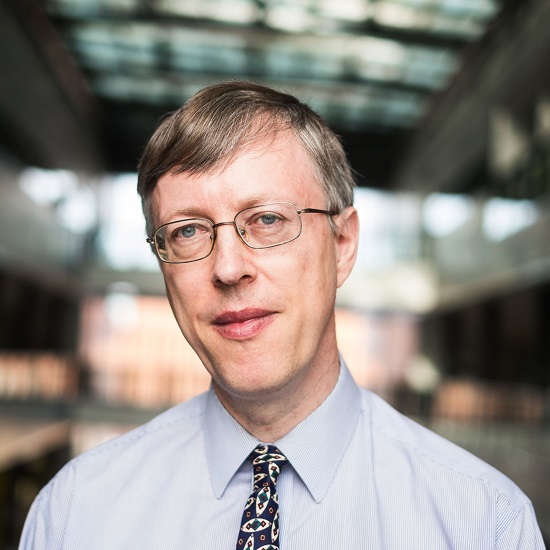
Professor Nicholas Higham FRS, University of Manchester, UK
Nicholas Higham is Royal Society Research Professor and Richardson Professor of Applied Mathematics in the School of Mathematics at the University of Manchester. He is a Fellow of the Royal Society, a SIAM Fellow, a Member of Academia Europaea, and served as President of the Society for Industrial and Applied Mathematics (SIAM), 2017–2018.
Much of his research is concerned with the accuracy and stability of numerical algorithms, and the second edition of his monograph on this topic was published by SIAM in 2002. His most recent books are Functions of Matrices: Theory and Computation (SIAM, 2008), the first ever research monograph on matrix functions, and the 1000-page The Princeton Companion to Applied Mathematics (2015), of which he was editor.
His current research interests include multiprecision and mixed precision numerical linear algebra algorithms.
He blogs about applied mathematics at https://nickhigham.wordpress.com/.
13:00 - 13:30 |
Memory-aware algorithms for automatic differentiation and backpropagation
In this talk Dr Pallez will discuss the impact of memory in the computation of automatic differentiation or for the back-propagation step of machine learning algorithms. He will show different strategies based on the amount of memory available. In particular he will discuss optimal strategies when one can reuse memory slots, and when considering a hierarchical memory platform. ![]() Dr Guillaume Pallez (Aupy), Inria, France
![]() Dr Guillaume Pallez (Aupy), Inria, FranceGuillaume Pallez is a researcher at Inria Bordeaux Sud-Ouest. His research interests include algorithm design and scheduling techniques for parallel and distributed platforms, and also the performance evaluation of parallel systems. Specifically his current focus revolves around data-aware scheduling at the different levels of the memory hierarchy (cache, memory, buffers, disks). He completed his PhD at ENS Lyon in 2014 on reliable and energy efficient scheduling strategies in High-Performance Computing. He served as the Technical Program vice-chair for SC'17, workshop chair for SC'18 and algorithm track vice-chair for ICPP'18. |
|
---|---|---|
13:40 - 14:10 |
Post-K: the first ‘exascale’ supercomputer for convergence of HPC and big data/AI
With the rapid rise and increase of Big Data and AI as a new breed of high-performance workloads on supercomputers, we need to accommodate them at scale, and thus the need for R&D for HW and SW Infrastructures where traditional simulation-based HPC and Big Data/AI would converge. Post-K is the flagship next generation national supercomputer being developed by Riken R-CCS and Fujitsu in collaboration. Post-K will have hyperscale datacenter class resource in a single exascale machine, with well more than 150,000 nodes of sever-class A64fx many-core Arm CPUs with the new SVE (Scalable Vector Extension) for the first time in the world, augmented with HBM2 memory paired with CPUs for the first time, exhibiting nearly a Terabyte/s memory bandwidth for both HPC and Big Data rapid data movements, along with AI/deep learning. Post-K’s target performance is 100 times speedup on some key applications cf its predecessor, the K-Computer, realised through extensive co-design process involving the entire Japanese HPC community. It also will likely to be the premier big data and AI/ML infrastructure for Japan; currently, the group is conducting research to scale deep learning to more than 100,000 nodes on Post-K, where they expect to obtain near top GPU-class performance on each node. ![]() Director Satoshi Matsuoka, RIKEN Center for Computational Science, Japan
![]() Director Satoshi Matsuoka, RIKEN Center for Computational Science, JapanSatoshi Matsuoka from April 2018 has been the director of Riken R-CCS, the tier-1 HPC center that represents HPC in Japan, currently hosting the K Computer and developing the next generation Post-K machine, along with multitudes of ongoing cutting edge HPC research being conducted. He was the leader of the TSUBAME series of supercomputers, at Tokyo Institute of Technology, where he still holds a Professor position, to continue his research activities in HPC as well as scalable Big Data and AI. He has won many accolades including the JSPS Prize from the Japan Society for Promotion of Science in 2006, presented by his Highness Prince Akishino; the ACM Gordon Bell Prize in 2011; the Commendation for Science and Technology by the Minister of Education, Culture, Sports, Science and Technology in 2012; and the 2014 IEEE-CS Sidney Fernbach Memorial Award, the highest prestige in the field of HPC. |
|
14:30 - 14:40 | Discussion | |
14:50 - 15:20 |
Accelerated sparse linear algebra: emerging challenges and capabilities for numerical algorithms and software
For the foreseeable future, the performance potential for most high-performance scientific software will require effective use of hosted accelerator processors, characterised by massive concurrency, high bandwidth memory and stagnant latency. These accelerators will also typically have a backbone of traditional networked multicore processors that serve as the starting point for porting applications and the default execution environment for non-accelerated computations. In this presentation Dr Heroux will briefly characterise experiences with these platforms for sparse linear algebra computations. He will discuss requirements for sparse computations that are distinct from dense computations and how these requirements impact algorithm and software design. He will also discuss programming environment trends that can impact design and implementation choices. In particular, Dr Heroux will discuss strategies and practical challenges of increasing performance portability across the diverse node architectures that continue to emerge, as computer system architects innovate to keep improving performance in the presence of stagnant latencies, and simultaneously add capabilities that address the needs of data science. Finally, Dr Heroux will discuss the role and potential for resilient computations that may eventually be required as we continue to push the limits of system reliability. While system designers have managed to preserve the illusion of a 'reliable digital machine' for scientific software developers, the cost of doing so continues to increase, as does the risk that a future leadership platform may never reach acceptable reliability levels. If we can develop resilient algorithms and software that survives failure events, we can reduce the cost, schedule delays and complexity challenges of future systems. ![]() Dr Michael A Heroux, Sandia National Laboratories, USA
![]() Dr Michael A Heroux, Sandia National Laboratories, USAMichael Heroux is a Senior Scientist at Sandia National Laboratories, Director of SW Technologies for the US DOE Exascale Computing Project (ECP) and Scientist in Residence at St. John’s University, MN. His research interests include all aspects of scalable scientific and engineering software for new and emerging parallel computing architectures. He leads several projects in this field: ECP SW Technologies is an integrated effort to provide the software stack for ECP. The Trilinos Project (2004 R&D 100 winner) is an effort to provide reusable, scalable scientific software components. The Mantevo Project (2013 R&D 100 winner) is focused on the development of open source, portable mini-applications and mini-drivers for the co-design of future supercomputers and applications. HPCG is an official TOP 500 benchmark for ranking computer systems, complementing LINPACK. |
|
15:10 - 15:20 | Discussion | |
15:20 - 15:50 | Coffee | |
15:30 - 16:00 | Closing remarks | |
16:20 - 16:30 | Discussion |