Machine learning and AI in biological science, drug discovery and medicine
This Royal Society conference brought together stakeholders from industry and academia to explore advances in machine learning and artificial intelligence for biological research, drug discovery and medicine. Whilst these computational technologies are already transforming biological, clinical and pharmaceutical research, significant obstacles remain, in particular around skills, financing, and accessibility. Concerted industry/academia collaboration is required to address these challenges.
Three sessions around biology, chemistry and medicine included talks on machine learning for target discovery and 'omic technologies, AI-based chemoinformatics, and computational clinical trial design, as well as the classical prediction of protein folding, followed by a keynote address from Dr Demis Hassabis CBE FREng FRS (DeepMind).
The conference concluded with a panel discussion that addressed how machine learning and AI can be used to advance medicine and healthcare.
Conference report
Download the conference report.
About the conference series
Supported by AstraZeneca, the meeting formed part of the Royal Society’s Transforming our future series in the life sciences. These meetings are unique, high-level events that address the scientific and technical challenges of the next decade. Each conference features cutting-edge science from industry and academia and brings together leading experts from the scientific community, including regulatory, charity and funding bodies.
Watch the event recording
Organisers
Schedule
09:00-09:05 |
Opening remarks
Sir Adrian SmithPresident, the Royal Society Sir Adrian SmithPresident, the Royal Society Adrian Smith became President of the Royal Society on 30 November 2020. He is also Institute Director and Chief Executive of The Alan Turing Institute. He is a mathematician with expertise in Bayesian statistics. This branch of mathematics represents uncertainties in the form of probabilities, which are then modified through the mechanism of Bayes theorem as new information becomes available. Adrian's comprehensive publications on diverse areas of Bayesian statistics have had a major impact on statistical practice in a wide range of disciplines and application areas. Between 2008-2012, he was Director General, Knowledge and Innovation in BIS (now BEIS) and has previously worked with the UK Higher Education Funding and Research Councils. Adrian is Chair of the Board of the Diamond Light Source and is also a board member of the UK Atomic Energy Authority. In 2017, he carried out a review of the maths curriculum for 16-18 year olds for the Treasury and Department for Education. In the 2011 New Year Honours list, he was awarded the title of Knight Bachelor. |
---|
Chair
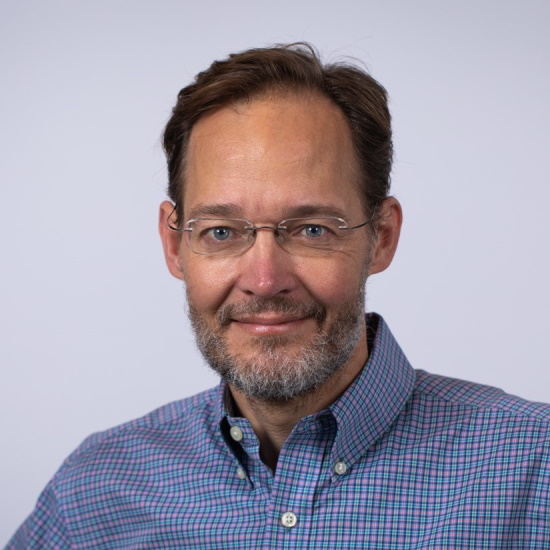
Dr Claus Bendtsen
AstraZeneca
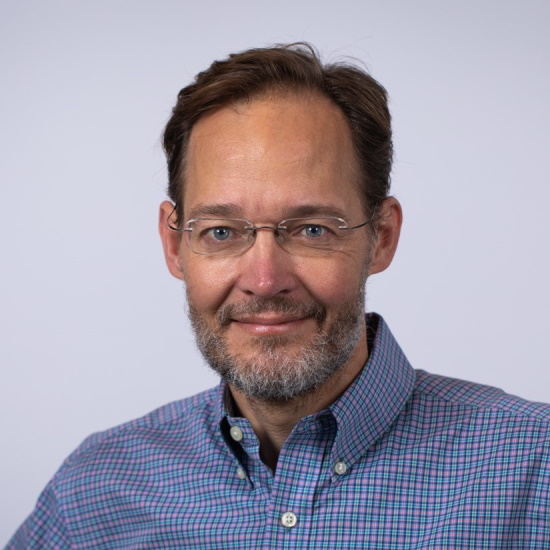
Dr Claus Bendtsen
AstraZeneca
Claus Bendtsen is an executive director at AstraZeneca where he heads Data Sciences & Quantitative Biology as part of Discovery Sciences. Prior to joining AstraZeneca, he held positions at Novartis and Merck & Co. Earlier in his career he co-founded three start-ups and worked in academia. He holds a PhD in applied mathematics and an MBA. He has more than 75 publications and 20 years of experience in the pharmaceutical industry.
09:10-09:30 |
AI-augmented target discovery
The complexity of human disease poses significant challenges in the translation of basic research into safe and effective therapies, with at least 50% of drugs failing in Phase II and Phase III trials for lack of efficacy. To tackle this complexity and enable data-driven discoveries, BenevolentAI has built a comprehensive knowledge graph incorporating and capitalising on many orthogonal data modalities, to build a detailed mechanistic representation of the dysregulated processes that underlie human disease. Powerful AI and ML tools are used to interrogate this corpus of knowledge, to hypothesise novel biological targets of potential therapeutic value for any disease of interest. These hypotheses are experimentally validated in physiologically relevant human patient-derived cell based systems, before entering the BenevolentAI drug discovery portfolio. In this talk, Dr Phelan will describe the current status of AI in drug discovery and her vision for future technological solutions that will help to overcome some of the key challenges required to affect change across the entire R&D value chain. ![]() Dr Anne PhelanBenevolentAI, UK ![]() Dr Anne PhelanBenevolentAI, UK Dr Anne Phelan is BenevolentAI’s Chief Scientific Officer and is responsible for all aspects of drug discovery from therapeutic area selection through to target identification, building BenevolentAI’s drug discovery portfolio and delivering candidate molecules to clinical development. She is also the Site Head for BenevolentAI’s Cambridge Laboratories. Anne’s key strengths focus on promoting organisational delivery and productivity. She is passionate about building high-functioning and cohesive teams with equal gender representation at every level that are committed to delivering life-changing medicines to the clinic. Anne has over 25 years of experience in pharma and biotech and has worked on all stages from early discovery to late-stage drug development. Before joining BenevolentAI, Anne worked for pharma (Pfizer) and biotech (Mission Therapeutics), across a wide range of therapeutic areas including fibrosis, pain, arthritis and rheumatology, and neurodegeneration. She was Head of Pharmacology and Chief Operating Officer for Pfizer in the UK where she was responsible for the generation of primary and secondary data to support the portfolio. Anne holds a BSc and PhD in Genetics from the University of Liverpool, UK. |
---|---|
09:30-09:50 |
Mapping and navigating biology and chemistry with genome-scale imaging
Image-based readouts of biology are information-rich and inexpensive. Yet historically, bespoke data collection methods and the intrinsically unstructured nature of image data have made these assays difficult to work with at scale. This presentation will discuss advances made at Recursion to industrialise the use of cellular imaging to drive drug discovery. In particular, the use of deep learning allows the transformation of unstructured images into biologically meaningful representations, and enables a 'map of biology' relating genetic and chemical perturbations to scale drug discovery. Dr Haque will further discuss how publicly-shared resources from Recursion, including the RxRx3 dataset and MolRecTM application, enable downstream research both on cellular images themselves and on deep learning-derived embeddings, making advanced image analysis more accessible to researchers worldwide. ![]() Dr Imran HaqueRecursion Pharmaceuticals ![]() Dr Imran HaqueRecursion Pharmaceuticals Dr Haque leads data science at Recursion, an interdisciplinary group spanning AI/ML, computational biology and computational chemistry that aims to build and apply Recursion’s maps of biology for drug discovery. Prior to Recursion, he was the first chief scientific officer at Freenome, leading R&D in developing early detection tests for colorectal cancer, and conducted guideline-changing research as VP Scientific Affairs at Counsyl (now Myriad Women's Health). He earned his BSc degree from UC Berkeley and a PhD in computer science from Stanford University, where his research on large-scale machine learning systems for drug discovery was co-advised by Vijay Pande and Daphne Koller. |
09:50-10:10 |
Digital Twins for personalised oncology
Cancer incidence is steadily increasing and is a major burden for patients, their families and society. Despite new treatments such as immunotherapies, currently only around 25% of cancer patients respond to treatments with drugs or biologicals leading to a cure or a decrease in disease progression (Spear et al 2001). New approaches are needed. In this talk, Walter will introduce Digital Twin strategies that aim to improve cancer diagnosis and treatment by constructing computer models of cancer patients. These models allow simulation of the disease in silico and optimisation and selection of the best possible therapy for each patient. Using childhood cancer as a paradigm, Walter will discuss the status of Digital Twin technology, what the current challenges are and what he hopes the field will achieve over the next five to ten years. ![]() Professor Walter KolchUniversity College Dublin ![]() Professor Walter KolchUniversity College Dublin Walter Kolch is the Director of Systems Biology Ireland and the Precision Oncology Ireland Consortium. Trained as an MD, he has worked in experimental clinical research, pharmaceutical industry, and basic biological research. Before moving to Dublin in 2009 he held a chair for molecular cell biology at the University of Glasgow and was a senior group leader at the Beatson Institute for Cancer Research in Glasgow, Scotland. Walter is best known for his work in oncogene signal transduction, proteomics, systems biology, and precision medicine. He is ranked No. 3 in world in precision oncology by citations. He has made salient contributions to elucidating the function of the Raf kinases and the ERK pathway, and more recently to understand network-wide effects of oncogenes. His current research interest focuses on understanding molecular mechanisms of malignant transformation, network-mediated drug resistance in cancer, and the construction of Digital Twins for personalized cancer diagnosis and therapy. Walter serves on several editorial boards and scientific advisory boards and is a Fellow of the Royal Society of Edinburgh and a Member of the Royal Irish Academy. |
10:10-10:35 |
Q&A and discussion
![]() Professor Walter KolchUniversity College Dublin ![]() Professor Walter KolchUniversity College Dublin Walter Kolch is the Director of Systems Biology Ireland and the Precision Oncology Ireland Consortium. Trained as an MD, he has worked in experimental clinical research, pharmaceutical industry, and basic biological research. Before moving to Dublin in 2009 he held a chair for molecular cell biology at the University of Glasgow and was a senior group leader at the Beatson Institute for Cancer Research in Glasgow, Scotland. Walter is best known for his work in oncogene signal transduction, proteomics, systems biology, and precision medicine. He is ranked No. 3 in world in precision oncology by citations. He has made salient contributions to elucidating the function of the Raf kinases and the ERK pathway, and more recently to understand network-wide effects of oncogenes. His current research interest focuses on understanding molecular mechanisms of malignant transformation, network-mediated drug resistance in cancer, and the construction of Digital Twins for personalized cancer diagnosis and therapy. Walter serves on several editorial boards and scientific advisory boards and is a Fellow of the Royal Society of Edinburgh and a Member of the Royal Irish Academy. ![]() Dr Imran HaqueRecursion Pharmaceuticals ![]() Dr Imran HaqueRecursion Pharmaceuticals Dr Haque leads data science at Recursion, an interdisciplinary group spanning AI/ML, computational biology and computational chemistry that aims to build and apply Recursion’s maps of biology for drug discovery. Prior to Recursion, he was the first chief scientific officer at Freenome, leading R&D in developing early detection tests for colorectal cancer, and conducted guideline-changing research as VP Scientific Affairs at Counsyl (now Myriad Women's Health). He earned his BSc degree from UC Berkeley and a PhD in computer science from Stanford University, where his research on large-scale machine learning systems for drug discovery was co-advised by Vijay Pande and Daphne Koller. ![]() Dr Anne PhelanBenevolentAI, UK ![]() Dr Anne PhelanBenevolentAI, UK Dr Anne Phelan is BenevolentAI’s Chief Scientific Officer and is responsible for all aspects of drug discovery from therapeutic area selection through to target identification, building BenevolentAI’s drug discovery portfolio and delivering candidate molecules to clinical development. She is also the Site Head for BenevolentAI’s Cambridge Laboratories. Anne’s key strengths focus on promoting organisational delivery and productivity. She is passionate about building high-functioning and cohesive teams with equal gender representation at every level that are committed to delivering life-changing medicines to the clinic. Anne has over 25 years of experience in pharma and biotech and has worked on all stages from early discovery to late-stage drug development. Before joining BenevolentAI, Anne worked for pharma (Pfizer) and biotech (Mission Therapeutics), across a wide range of therapeutic areas including fibrosis, pain, arthritis and rheumatology, and neurodegeneration. She was Head of Pharmacology and Chief Operating Officer for Pfizer in the UK where she was responsible for the generation of primary and secondary data to support the portfolio. Anne holds a BSc and PhD in Genetics from the University of Liverpool, UK. |
Chair
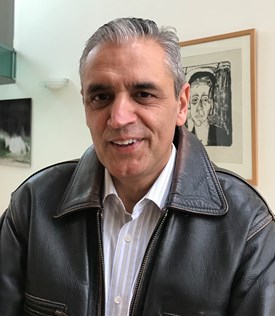
Dr Harren Jhoti OBE FMedSci FRS
Astex Pharmaceuticals
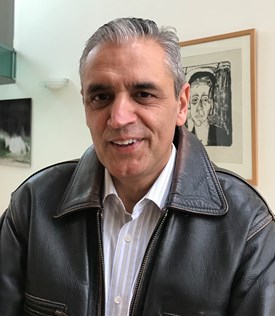
Dr Harren Jhoti OBE FMedSci FRS
Astex Pharmaceuticals
Dr Harren Jhoti FMedSci FRS is a structural biologist whose main interest has been rational drug design. He is President & CEO of Astex Pharmaceuticals in Cambridge, a biotech company he co-founded in 1999. He pioneered the development of ragment-based drug discovery, an approach now widely used in pharmaceutical and academic drug discovery centres to discover new medicines.
Astex’s first drug, called Kisqali, which originated from a Novartis collaboration, was approved in 2017 for patients with metastatic breast cancer in the US and EU. In 2013 Astex was acquired by Otsuka Pharmaceuticals for $886m, and operates as a wholly-owned subsidiary of the Japanese company. Prior to Astex, Harren was Head of Structural Biology at GlaxoWellcome (now GSK).
In 2018 Harren received the Lifetime Achievement Award from the BIA, the UK BioIndustry Association. He is a Fellow of the Academy of Medical Sciences, the Royal Society of Chemistry and the Royal Society of Biology. He was awarded the Prous Institute-Overton and Meyer Award by the European Federation for Medicinal Chemistry in 2012 and named by the Royal Society of Chemistry as World Entrepreneur of the Year for 2007.
11:10-11:30 |
Integrating chemical and biological data: a focus on relevance and translation will boost in vivo-relevant drug discovery
The amount of chemical and biological data has increased in both public and private domains, and algorithm and hardware design for machine learning has also progressed tremendously in the last ten years. This has enabled rapid development of machine learning for drug discovery. Several ‘AI-designed drugs’ have already entered clinical phases, and press releases now describe the design of functional proteins and antibodies from scratch. However, the attempt to marry algorithms with drug discovery often disregards the in vivo relevance of our current capabilities for processing chemical and biological data. In this talk, Dr Bender will pose that reductionist thinking remains pervasive in the field, and how, in combination with a lack of relevant data, our limited ability to handle it computationally with respect to in vivo-relevant decisions and the formation of many narrow specialist domains, this is undermining our ability to harness the full potential of available chemical and biological data. This talk will discuss how changing several areas, including data usage, algorithms and human mindset, might enable society to fully benefit from available computer power when it comes to in vivo-relevant decision making in drug discovery in the future. ![]() Professor Andreas BenderUniversity of Cambridge and Pangea Botanica ![]() Professor Andreas BenderUniversity of Cambridge and Pangea Botanica Dr Andreas Bender is a Professor for Molecular Informatics at Cambridge University as well as Chief Technology & Informatics Officer (CITO) at PangeAI, part of Pangea Botanica. Previously he was a Director for Digital Life Sciences at Nuvisan in Berlin, as well as as an Associate Director for Data Science and AI in the Clinical Pharmacology & Safety Sciences group at AstraZeneca. In his work, Andreas is involved in the integration and analysis of chemical and biological data from different sources, such as structural and bioactivity data, gene expression readouts, cellular imaging data, pathway information, etc. The computational analysis of this information then aims to understand phenotypic compound action such as cellular readouts and organism-level effects on a mechanistic level, predicting molecular properties related to compound efficacy and toxicity, as well as compound repurposing. On the entrepreneurial side, Andreas was involved in setting up Healx Ltd (for data-driven drug repurposing) and PharmEnable Ltd (for designing novel chemistry for targets that are difficult to drug conventionally), both based in Cambridge, UK. He received his PhD from the University of Cambridge and worked in the Lead Discovery Informatics group at Novartis in Cambridge, MA as well as at Leiden University in the Netherlands before his current post. |
---|---|
11:30-11:50 |
Machine learning to predict protein function from sequence - therapeutic applications
A central challenge in biochemistry is the prediction of the functional properties of a protein from its amino acid sequence, as it can lead to the discovery of new proteins with specific functionality and a better understanding of the functional effect of genomic mutations. Experimental and computational data enable the training and validation of powerful machine learning models that predict protein function directly from sequence. This talk will present deep learning models that accurately predict functional domains within protein sequences, and large language models that generate textual descriptions of protein sequences, collectively adding millions of annotations to public databases. Technical breakthroughs enable data on the sequence-to-function relationship to be rapidly acquired. However, the cost and latency of wet lab experiments means that we require new methods to find 'hits' (sequences that meet the function requirements of the campaign) in few experimental rounds, where each round contains a large batch of sequence designs. In this talk, Dr Colwell will discuss model-based optimisation approaches that take advantage of sample inefficient methods to find diverse sequence candidates for experimental evaluation. The potential of these approaches will be illustrated through three case studies demonstrating the design and experimental validation of proteins and peptides for therapeutic applications. |
11:50-12:10 |
Exploring the ability of machine learning-based virtual screening models to identify the functional groups responsible for binding
There is significant interest in developing machine learning models that can predict protein-ligand binding with high accuracy. Many recently proposed structure-based virtual screening models have done just this. However, there are still challenges in ensuring that these models are doing more than exploiting ligand-specific biases in the dataset making them potentially excellent predictors for proteins/ligands that are contained within the dataset but unable to generalise to unseen examples. One way to assess if a model can generalise is to test whether it understands the rules of intermolecular binding. Using synthetic data, the Deane lab has investigated whether different methods (from fingerprint-based random forests to deep learning virtual screening models) are learning more than the biases in datasets. The Deane lab found that their deep learning based virtual screening model, PointVS, identifies important functional groups with more efficiency than other methods tested. This suggests that it may generalise more effectively to new examples. Using attribution, the Deane lab demonstrated that PointVS can identify important interactions in real protein ligand complexes, and further, that it can be used to extract important binding pharmacophores from a given protein target when supplied with a number of bound structures. This information was then used to perform fragment elaboration, resulting in improvements in docking scores when compared to using structural information from a traditional data-based approach. This not only provides definitive proof that PointVS is learning to identify important binding interactions, but also constitutes the first deep learning-based method for extracting structural information from a target for molecule design. This presents an exciting opportunity for the future of lead discovery and drug development. ![]() Professor Charlotte Deane MBEUniversity of Oxford ![]() Professor Charlotte Deane MBEUniversity of Oxford Charlotte is Professor of Structural Bioinformatics in the Department of Statistics at the University of Oxford and Chief Scientist of Biologics AI at Exscientia. She is also a co-director of the Systems Approaches to Biomedical Research Centre for Doctoral Training which she founded in 2009. She served on SAGE, the UK Government’s Scientific Advisory Group for Emergencies, during the COVID-19 pandemic, and acted as UK Research and Innovation’s COVID-19 Response Director. She has held numerous senior roles at the University of Oxford and until recently was the Deputy Executive Chair of the UK’s Engineering and Physical Sciences Research Council. She was appointed Member of the Order of the British Empire (MBE) in the 2022 Birthday Honours for services to COVID-19 research. At Oxford, Charlotte leads the Oxford Protein Informatics Group (OPIG), who work on diverse problems across immunoinformatics, protein structure and small molecule drug discovery using statistics, AI and computation to generate biological and medical insight. Her work focuses on the development of novel algorithms, tools and databases that are openly available to the community. These tools are widely used web resources and are also part of several Pharma drug discovery pipelines. Charlotte is on several advisory boards and has consulted extensively with industry. She has set up a consulting arm within her own research group as a way of promoting industrial interaction and use of the group’s software tools. |
12:10-12:35 |
Q&A and discussion
![]() Professor Andreas BenderUniversity of Cambridge and Pangea Botanica ![]() Professor Andreas BenderUniversity of Cambridge and Pangea Botanica Dr Andreas Bender is a Professor for Molecular Informatics at Cambridge University as well as Chief Technology & Informatics Officer (CITO) at PangeAI, part of Pangea Botanica. Previously he was a Director for Digital Life Sciences at Nuvisan in Berlin, as well as as an Associate Director for Data Science and AI in the Clinical Pharmacology & Safety Sciences group at AstraZeneca. In his work, Andreas is involved in the integration and analysis of chemical and biological data from different sources, such as structural and bioactivity data, gene expression readouts, cellular imaging data, pathway information, etc. The computational analysis of this information then aims to understand phenotypic compound action such as cellular readouts and organism-level effects on a mechanistic level, predicting molecular properties related to compound efficacy and toxicity, as well as compound repurposing. On the entrepreneurial side, Andreas was involved in setting up Healx Ltd (for data-driven drug repurposing) and PharmEnable Ltd (for designing novel chemistry for targets that are difficult to drug conventionally), both based in Cambridge, UK. He received his PhD from the University of Cambridge and worked in the Lead Discovery Informatics group at Novartis in Cambridge, MA as well as at Leiden University in the Netherlands before his current post. ![]() Professor Charlotte Deane MBEUniversity of Oxford ![]() Professor Charlotte Deane MBEUniversity of Oxford Charlotte is Professor of Structural Bioinformatics in the Department of Statistics at the University of Oxford and Chief Scientist of Biologics AI at Exscientia. She is also a co-director of the Systems Approaches to Biomedical Research Centre for Doctoral Training which she founded in 2009. She served on SAGE, the UK Government’s Scientific Advisory Group for Emergencies, during the COVID-19 pandemic, and acted as UK Research and Innovation’s COVID-19 Response Director. She has held numerous senior roles at the University of Oxford and until recently was the Deputy Executive Chair of the UK’s Engineering and Physical Sciences Research Council. She was appointed Member of the Order of the British Empire (MBE) in the 2022 Birthday Honours for services to COVID-19 research. At Oxford, Charlotte leads the Oxford Protein Informatics Group (OPIG), who work on diverse problems across immunoinformatics, protein structure and small molecule drug discovery using statistics, AI and computation to generate biological and medical insight. Her work focuses on the development of novel algorithms, tools and databases that are openly available to the community. These tools are widely used web resources and are also part of several Pharma drug discovery pipelines. Charlotte is on several advisory boards and has consulted extensively with industry. She has set up a consulting arm within her own research group as a way of promoting industrial interaction and use of the group’s software tools. |
Chair
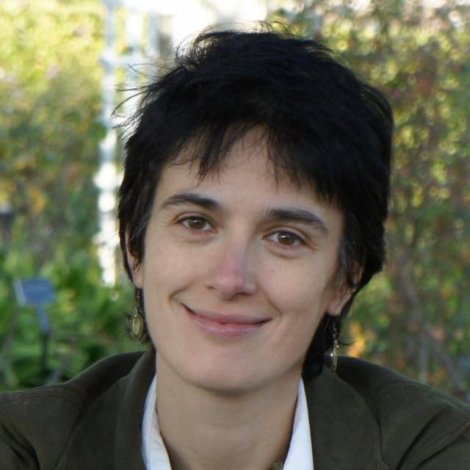
Professor Mihaela van der Schaar
University of Cambridge
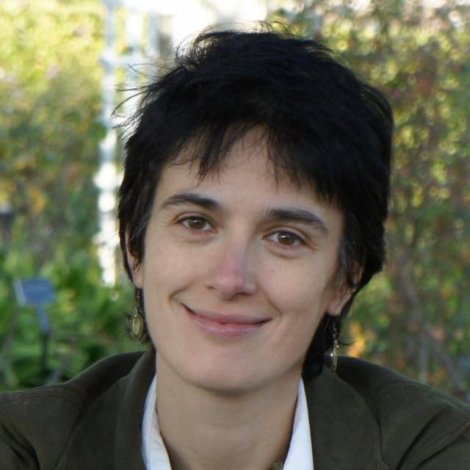
Professor Mihaela van der Schaar
University of Cambridge
Mihaela van der Schaar is the John Humphrey Plummer Professor of Machine Learning, Artificial Intelligence and Medicine at the University of Cambridge and a Fellow at The Alan Turing Institute in London. In addition to leading the van der Schaar lab, Mihaela is founder and director of the Cambridge Centre for AI in Medicine (CCAIM).
Mihaela was elected IEEE Fellow in 2009. She has received numerous awards, including the Oon Prize on Preventative Medicine from the University of Cambridge (2018), a National Science Foundation CAREER Award (2004), 3 IBM Faculty Awards, the IBM Exploratory Stream Analytics Innovation Award, the Philips Make a Difference Award and several best paper awards, including the IEEE Darlington Award.
Mihaela is personally credited as inventor on 35 USA patents, many of which are still frequently cited and adopted in standards. She has made over 45 contributions to international standards for which she received 3 ISO Awards. In 2019, a Nesta report determined that Mihaela was the most-cited female AI researcher in the UK.
13:40-14:00 |
Transforming the practice of medicine - a human-centred perspective
‘Healthcare is perhaps AI’s most urgent application' – Satya Nadella, CEO of Microsoft. This urgency has been brought to life for all by the COVID-19 pandemic; now, healthcare providers are expected to perform and transform at scale, in real time. The recent disruptions in AI have the potential to revolutionise medical care, but to fully leverage its potential, a human-centred approach is essential. This talk will share various opportunities and challenges for AI to deliver real-world impact, while prioritising the human aspect of high-quality care. Illustrative examples will highlight how a human-centred approach is crucial in transforming the practice of medicine for the future. ![]() Aditya NoriMicrosoft Health Futures ![]() Aditya NoriMicrosoft Health Futures Aditya Nori leads healthcare research and delivery within Microsoft Health Futures, which aims to empower every person on the planet to live a healthier future. He leads the following research and incubation programmes: Biomedical Imaging, Biomedical NLP (natural language processing), Biomedical Signal Processing and Health Access/Equity. Previously, Aditya founded the Health Intelligence theme at Microsoft Research, Cambridge, UK, with a mission to transform the practice of medicine via trustworthy and human-centred AI. As a researcher at MSR, he has developed AI-based productivity tools for cancer treatment (The InnerEye Project) and explored various synergies between programming languages and machine learning. This has inspired new perspectives in formal verification, probabilistic programming, and reliable machine learning. He has developed several programmer productivity tools, including the 2nd generation of the Static Driver Verifier toolkit for Microsoft Windows. |
---|---|
14:00-14:20 |
Closing the loop with AI: integrating large scale population health databases, observational cohorts and clinical trials for drug discovery
A new era of data in biology and medicine is upon us - caused by the impact of cheap, high-quality measurement technology at scale (genome sequencing, single cell methods) and the increasing use of electronic health records. The discovery of new medicines needs to integrate data from large population level datasets with finely-detailed, smaller-scale and disease-specific observational cohorts. The entire pharmaceutical development process can be considered a learning loop, with feedback into early discovery. An example of this is the use of new complex cellular models (often referred to as biological digital twins) that use machine learning models to bridge to the responses of individual patients. This talk will illustrate how GSK is using machine learning models increasingly to integrate complex multimodal multi-scale data in both clinical development and early discovery. ![]() Dr Kim BransonGSK ![]() Dr Kim BransonGSK Dr Kim Branson is Senior Vice President and Global Head of AI/ML at GSK, based in San Francisco. He leads the GSK.ai team, a global organization of 100 machine learning researchers and engineers who are pioneering the application of AI to drug discovery and development. Kim brings a deep expertise in modelling and machine learning to drug and vaccine discovery, combining perspectives spanning academia to technology start-up. Under his leadership, GSK has built one of the industry’s few completely in-house AI efforts to leverage the potential of complex genetic data and maximize GSK’s industry-leading collaborations. Kim joined GSK from Genentech where he was Head of AI, Early Clinical Development. He has been involved in large-scale machine learning and medical informatics initiatives for more than 20 years, over a range of ventures from computational drug design to disease risk prediction. Previously, Kim helped found several Silicon Valley start-ups, including Discovery Engine (acquired by Twitter), Gliimpse as Chief Scientist (acquired by Apple) and Lumiata, a predictive health analytics company. Kim received degrees from the University of Adelaide and a PhD from the University of Melbourne. He was a Peter Doherty fellow and did his postdoctoral training at Stanford University. |
14:20-14:40 |
Machine learning for translatable biomarkers and targets
Despite significant progress in modern medicine, the design and development of new medicines remains very challenging and with a low probability of success. Improving our understanding of the underlying biology of disease can help identify new therapies and predict which interventions will positively (or negatively) affect clinical outcomes in diverse groups of patients. This talk will describe how insitro is using cutting-edge machine learning methods to develop a new approach to drug development that uses biological and clinical data to design novel, safe, and effective therapies that help more people, faster and at a lower cost. The process begins with the generation and aggregation of large amounts of high-content biomarker data from both human samples and human-derived cellular systems. These data are used to create a representation of biological states, enabling the construction of machine learning models that reveal novel therapeutic targets, identify coherent patient segments and predict the effect of different therapies on different patients. It will also explore insitro’s vision for the value of AI-driven biomarker identification in precision medicine. ![]() Professor Daphne Kollerinsitro, US ![]() Professor Daphne Kollerinsitro, US Daphne Koller is CEO and Founder of insitro, a machine learning-driven drug discovery company. She was the co-founder and co-CEO of Coursera, an online education platform for massive open online courses (MOOCs), which has reached over 100 million learners worldwide. Previously Daphne was the Rajeev Motwani Professor of Computer Science at Stanford University, where she served on the faculty for 18 years, and remains an Adjunct Faculty member. She is the author of over 300 refereed publications appearing in venues such as Science, Cell, Nature Genetics, NeurIPS, and ICML, with an h-index of over 145. In 2012 she was recognized as one of TIME Magazine’s 100 most influential people and Newsweek’s 10 most important people in 2010. She received the Sloan Foundation Faculty Fellowship in 1996, the ONR Young Investigator Award in 1998, the Presidential Early Career Award for Scientists and Engineers (PECASE) in 1999, the IJCAI Computers and Thought Award in 2001, the MacArthur Foundation Fellowship in 2004, the ACM Prize in Computing in 2008, the ACM AAAI Allen Newell Award in 2019, the IEEE CS Women of ENIAC Computer Pioneer award and the AnitaB.org Technical Leadership Abie Award Winner in 2022. Daphne was inducted into the National Academy of Engineering in 2011 and elected a fellow of the American Association for Artificial Intelligence in 2004, the American Academy of Arts and Sciences in 2014 and the International Society of Computational Biology in 2017. Her teaching has been recognized via the Stanford Medal for Excellence in Fostering Undergraduate Research and she is also a Bass University Fellow in Undergraduate Education. |
14:40-15:05 |
Q&A and discussion
![]() Professor Daphne Kollerinsitro, US ![]() Professor Daphne Kollerinsitro, US Daphne Koller is CEO and Founder of insitro, a machine learning-driven drug discovery company. She was the co-founder and co-CEO of Coursera, an online education platform for massive open online courses (MOOCs), which has reached over 100 million learners worldwide. Previously Daphne was the Rajeev Motwani Professor of Computer Science at Stanford University, where she served on the faculty for 18 years, and remains an Adjunct Faculty member. She is the author of over 300 refereed publications appearing in venues such as Science, Cell, Nature Genetics, NeurIPS, and ICML, with an h-index of over 145. In 2012 she was recognized as one of TIME Magazine’s 100 most influential people and Newsweek’s 10 most important people in 2010. She received the Sloan Foundation Faculty Fellowship in 1996, the ONR Young Investigator Award in 1998, the Presidential Early Career Award for Scientists and Engineers (PECASE) in 1999, the IJCAI Computers and Thought Award in 2001, the MacArthur Foundation Fellowship in 2004, the ACM Prize in Computing in 2008, the ACM AAAI Allen Newell Award in 2019, the IEEE CS Women of ENIAC Computer Pioneer award and the AnitaB.org Technical Leadership Abie Award Winner in 2022. Daphne was inducted into the National Academy of Engineering in 2011 and elected a fellow of the American Association for Artificial Intelligence in 2004, the American Academy of Arts and Sciences in 2014 and the International Society of Computational Biology in 2017. Her teaching has been recognized via the Stanford Medal for Excellence in Fostering Undergraduate Research and she is also a Bass University Fellow in Undergraduate Education. ![]() Aditya NoriMicrosoft Health Futures ![]() Aditya NoriMicrosoft Health Futures Aditya Nori leads healthcare research and delivery within Microsoft Health Futures, which aims to empower every person on the planet to live a healthier future. He leads the following research and incubation programmes: Biomedical Imaging, Biomedical NLP (natural language processing), Biomedical Signal Processing and Health Access/Equity. Previously, Aditya founded the Health Intelligence theme at Microsoft Research, Cambridge, UK, with a mission to transform the practice of medicine via trustworthy and human-centred AI. As a researcher at MSR, he has developed AI-based productivity tools for cancer treatment (The InnerEye Project) and explored various synergies between programming languages and machine learning. This has inspired new perspectives in formal verification, probabilistic programming, and reliable machine learning. He has developed several programmer productivity tools, including the 2nd generation of the Static Driver Verifier toolkit for Microsoft Windows. ![]() Dr Kim BransonGSK ![]() Dr Kim BransonGSK Dr Kim Branson is Senior Vice President and Global Head of AI/ML at GSK, based in San Francisco. He leads the GSK.ai team, a global organization of 100 machine learning researchers and engineers who are pioneering the application of AI to drug discovery and development. Kim brings a deep expertise in modelling and machine learning to drug and vaccine discovery, combining perspectives spanning academia to technology start-up. Under his leadership, GSK has built one of the industry’s few completely in-house AI efforts to leverage the potential of complex genetic data and maximize GSK’s industry-leading collaborations. Kim joined GSK from Genentech where he was Head of AI, Early Clinical Development. He has been involved in large-scale machine learning and medical informatics initiatives for more than 20 years, over a range of ventures from computational drug design to disease risk prediction. Previously, Kim helped found several Silicon Valley start-ups, including Discovery Engine (acquired by Twitter), Gliimpse as Chief Scientist (acquired by Apple) and Lumiata, a predictive health analytics company. Kim received degrees from the University of Adelaide and a PhD from the University of Melbourne. He was a Peter Doherty fellow and did his postdoctoral training at Stanford University. |
Chair
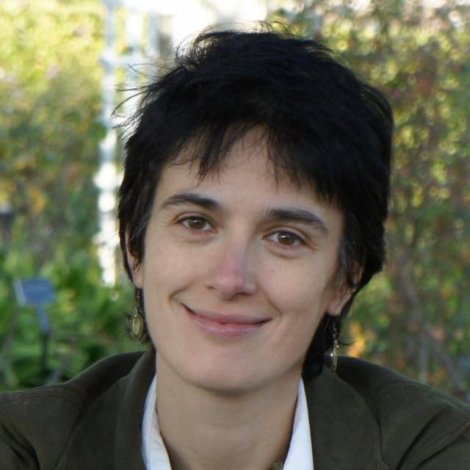
Professor Mihaela van der Schaar
University of Cambridge
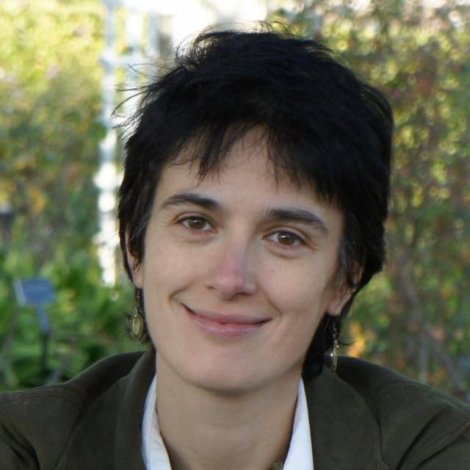
Professor Mihaela van der Schaar
University of Cambridge
Mihaela van der Schaar is the John Humphrey Plummer Professor of Machine Learning, Artificial Intelligence and Medicine at the University of Cambridge and a Fellow at The Alan Turing Institute in London. In addition to leading the van der Schaar lab, Mihaela is founder and director of the Cambridge Centre for AI in Medicine (CCAIM).
Mihaela was elected IEEE Fellow in 2009. She has received numerous awards, including the Oon Prize on Preventative Medicine from the University of Cambridge (2018), a National Science Foundation CAREER Award (2004), 3 IBM Faculty Awards, the IBM Exploratory Stream Analytics Innovation Award, the Philips Make a Difference Award and several best paper awards, including the IEEE Darlington Award.
Mihaela is personally credited as inventor on 35 USA patents, many of which are still frequently cited and adopted in standards. She has made over 45 contributions to international standards for which she received 3 ISO Awards. In 2019, a Nesta report determined that Mihaela was the most-cited female AI researcher in the UK.
15:35-16:45 |
Panel discussion
![]() Jia-Yi HarCathay Capital ![]() Jia-Yi HarCathay Capital Jia-Yi Har is a Partner at Cathay Health, a venture fund investing in early-stage convergence medicine companies developing technologies to address unmet medical needs along the healthcare value chain, from upstream drug development, clinical trial innovations, life science tools, diagnostics, advanced therapeutics platforms to downstream digital health solutions and connected devices. Jia-Yi brings nearly 20 years of professional experience in a career spanning healthcare investment, venture building, business management and scientific research. Prior to joining Cathay, she was part of the healthcare private equity team at Partners Group, a global private equity firm with $120 billion asset under management. Thereafter, she led Philips Healthcare’s Asia Pacific Imaging Software business and embarked on an entrepreneurial journey where she built and scaled early-stage health ventures in digital on-demand homecare and genetics. Prior to her business career, Jia Yi was an academic researcher in microbial genetics and biophysics at Massachusetts Institute of Technology and National University of Singapore. Jia-Yi holds a MSc in Civil & Environmental Engineering from MIT, where she received the Schoettler and Linden Earth System Fellowships. ![]() Prof Eoin McKinneyUniversity of Cambridge ![]() Prof Eoin McKinneyUniversity of Cambridge Professor Eoin McKinney is the Versus Arthritis Chair of Rheumatology in the Department of Medicine at the University of Cambridge, an honorary consultant in nephrology and transplantation at Cambridge University Hospitals NHS Foundation Trust and a faculty member of the Cambridge Centre for Artificial Intelligence in Medicine. His laboratory research explores ‘systems immunology’, studying the interface between immune responses to infection and those driving inflammatory pathology by applying machine learning methods to the integration of multi-omics data. He also explores the building of interpretable predictive models for rapid translation into clinical practice while informing underlying disease biology and identifying novel therapeutic strategies for inflammatory diseases. ![]() Dr Thomas CallenderUniversity College London ![]() Dr Thomas CallenderUniversity College London Tom Callender trained in medicine in Manchester, Oxford and London before specialising in public health and becoming a clinical academic at UCL, currently supported by a fellowship from the Wellcome Trust. As a researcher, his focus has been on the early detection of cancer, developing the first model of a polygenic risk-stratified screening programme for prostate cancer and now AI tools for risk-stratifying lung cancer screening. For his clinical and academic work, he has received the McEwan Award from the UK Faculty of Public Health and an American Association for Cancer Research (AACR) Scholar-in-Training Award. ![]() Dr Danielle BelgraveDeepMind ![]() Dr Danielle BelgraveDeepMind Dr Danielle Belgrave is a Senior Staff Research Scientist at DeepMind, where she leads a team focusing on machine learning for scientific discovery in health. Her research focuses on integrating medical domain knowledge, machine learning and causal modelling frameworks to understand health. Prior to joining DeepMind she led a team working on AI for mental health in the Healthcare Intelligence group at Microsoft Research and was a tenured research fellow at Imperial College London. She obtained a BSc in Mathematics and Statistics from London School of Economics, an MSc in Statistics from University College London and a PhD in the area of machine learning in health applications from the University of Manchester. Dr Belgrave serves on a number of boards including the UCL AI Centre for Doctoral Training, The Association for Health, Learning and Inference and Women in Machine Learning. She has been Program Chair (2022) and Tutorial Chair (2019, 2020) for NeurIPS, the largest international Machine Learning Conference. |
---|
16:45-17:25 |
Using AI to accelerate scientific discovery
The past decade has seen incredible advances in artificial intelligence. DeepMind has been in the vanguard of many of these big breakthroughs, pioneering the development of self-learning systems like AlphaGo, the first program to beat the world champion at the complex game of Go. Games have proven to be a great training ground for developing and testing AI algorithms, but the aim at DeepMind has always been to build general learning systems ultimately capable of solving important problems in the real world. We are on the cusp of an exciting new era in science, with AI poised to be a powerful tool for accelerating scientific discovery itself. DeepMind recently demonstrated this potential with AlphaFold, a solution to the 50-year grand challenge of protein structure prediction, culminating in the release of the most accurate and complete picture of the human proteome and release of the predicted structures of over 200 million proteins - nearly all catalogued proteins known to science. Dr Demis Hassabis, Google Deepmind
Dr Demis Hassabis, Google DeepmindDemis Hassabis is a neuroscientist and leading expert on the neural basis of memory and imagination. He was the co-founder and CEO of DeepMind, a neuroscience-inspired AI company, bought by Google in Jan 2014. He is now Vice President of Engineering at Google DeepMind and leads Google’s general AI efforts. Demis is a former child chess prodigy, who finished his A-levels two years early before coding the multi-million selling simulation game Theme Park aged 17. Following graduation from Cambridge University with a Double First in Computer Science he founded the pioneering videogames company Elixir Studios. After a decade of experience leading successful technology startups, Demis returned to academia to complete a PhD in cognitive neuroscience at UCL. His research connecting memory with imagination was listed in the top ten scientific breakthroughs of 2007 by the journal Science. Demis is a 5-times World Games Champion, a Fellow of the Royal Society of Arts, and the recipient of the Royal Society’s Mullard Award. |
---|
Chair
Sir Adrian Smith
President, the Royal Society
Sir Adrian Smith
President, the Royal Society
Adrian Smith became President of the Royal Society on 30 November 2020. He is also Institute Director and Chief Executive of The Alan Turing Institute.
He is a mathematician with expertise in Bayesian statistics. This branch of mathematics represents uncertainties in the form of probabilities, which are then modified through the mechanism of Bayes theorem as new information becomes available. Adrian's comprehensive publications on diverse areas of Bayesian statistics have had a major impact on statistical practice in a wide range of disciplines and application areas.
Between 2008-2012, he was Director General, Knowledge and Innovation in BIS (now BEIS) and has previously worked with the UK Higher Education Funding and Research Councils. Adrian is Chair of the Board of the Diamond Light Source and is also a board member of the UK Atomic Energy Authority. In 2017, he carried out a review of the maths curriculum for 16-18 year olds for the Treasury and Department for Education. In the 2011 New Year Honours list, he was awarded the title of Knight Bachelor.