Forecasting natural and social systems
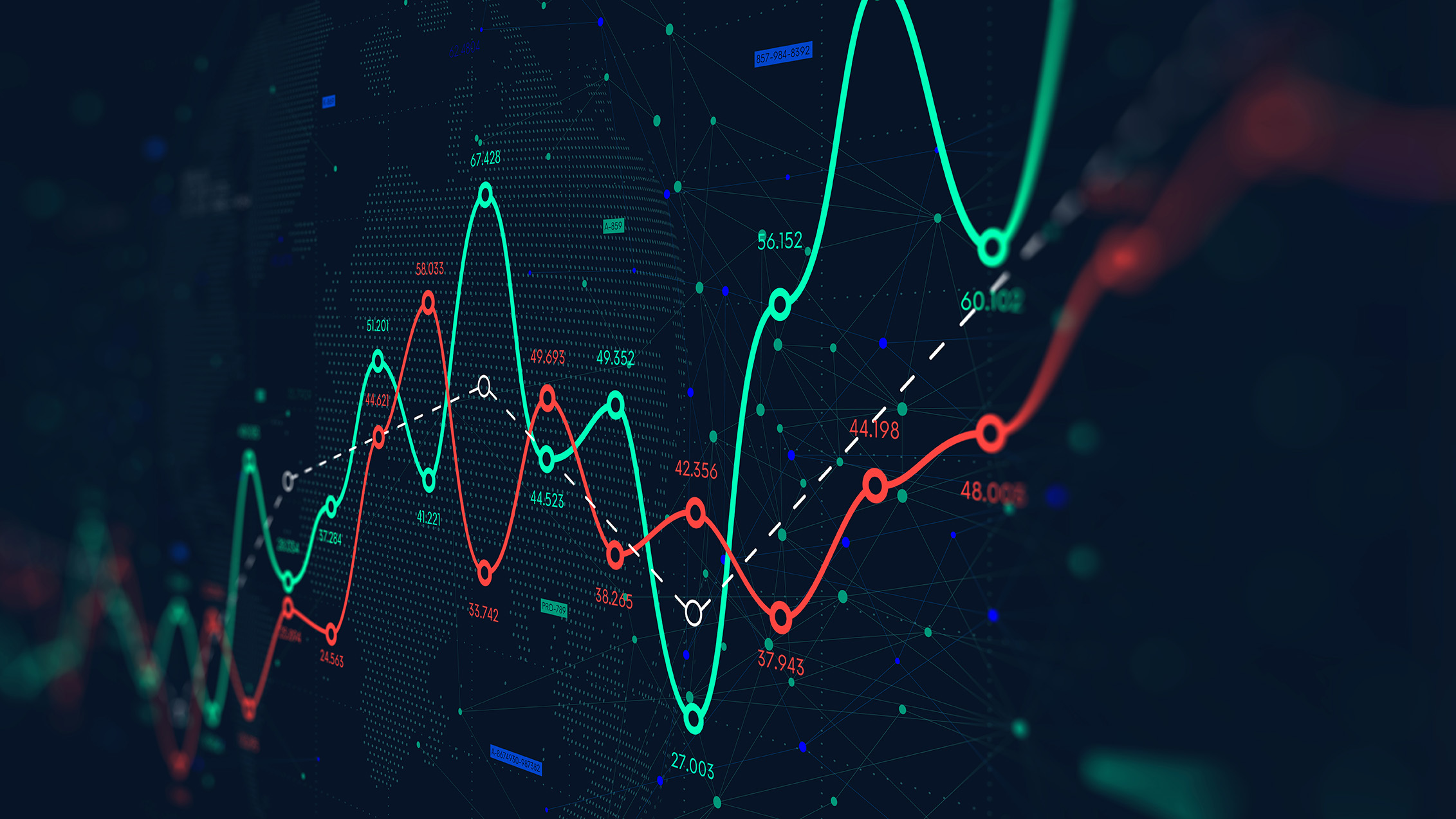
Scientific discussion meeting organised by Professor Sebastian Funk, Professor Nicholas Reich, Professor Steven Riley and Professor Rachel Lowe.
Science is used to underpin forecasts of many types, but methodologies are often developed in isolation within respective disciplines. This meeting aimed to convene leading experts in forecasting across disciplines to discuss the predictability of different systems, to exchange expertise, and to identify common challenges and possible solutions.
The schedule of talks, speaker biographies and abstracts are available below.
Attending this event
This event has now taken place.
Enquiries: contact the Scientific Programmes team
Organisers
Schedule
Chair
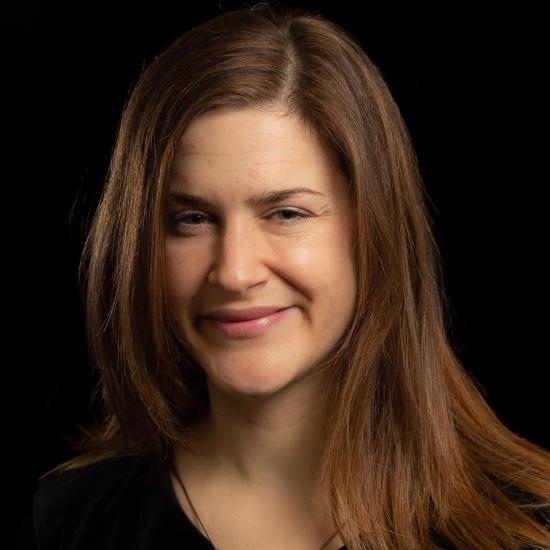
Professor Rachel Lowe, ICREA/Barcelona Supercomputing Center, Spain
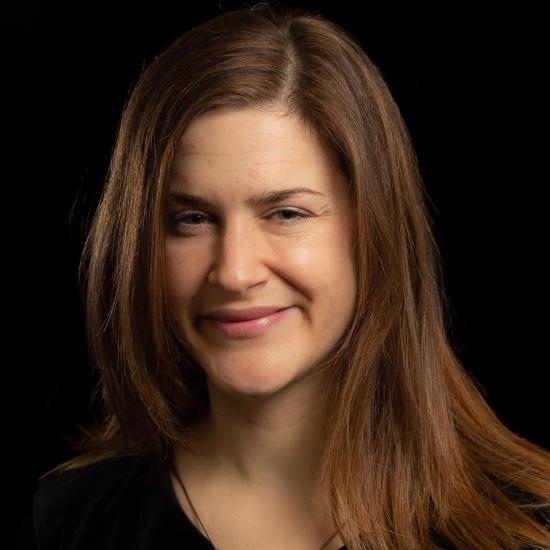
Professor Rachel Lowe, ICREA/Barcelona Supercomputing Center, Spain
Rachel Lowe is an ICREA Research Professor and Global Health Resilience Team Leader at the Barcelona Supercomputing Center. She also holds a Royal Society Dorothy Hodgkin Fellowship at the London School of Hygiene & Tropical Medicine. Rachel’s research focuses on co-developing policy-relevant methodological solutions, to enhance surveillance, preparedness and response to climate-sensitive disease outbreaks and emergence. She has published high impact research on integrating seasonal climate forecasts in early warning systems for infectious diseases in Latin America, the Caribbean and Southeast Asia. Rachel is the Director of the Lancet Countdown on health and climate change in Europe. She is a member of the World Meteorological Organization COVID-19 research task team and was a contributing author of the IPCC Sixth Assessment Report (WGII) chapter on risks across sectors and regions.
09:00-09:05 |
Introduction by the Royal Society and lead organiser
|
---|---|
09:05-09:30 |
An interpretable machine learning workflow with an application to economic forecasting
Andreas Joseph will discuss a generic workflow for the use of machine learning models to inform decision making and to communicate modelling results with stakeholders. It involves three steps: (1) a comparative model evaluation, (2) a feature importance analysis and (3) statistical inference based on Shapley value decompositions. Joseph will cover the different steps of the workflow in detail and demonstrate each by forecasting changes in US unemployment one year ahead using the well-established FRED-MD dataset. He will also illustrate that universal function approximators from the machine learning literature, including gradient boosting and artificial neural networks, outperform more conventional linear models. This better performance is associated with greater flexibility, allowing the machine learning models to account for time-varying and nonlinear relationships in the data generating process. The Shapley value decomposition identifies economically meaningful nonlinearities learned by the models. Shapley regressions for statistical inference on machine learning models enable us to assess and communicate variable importance akin to conventional econometric approaches. While Joseph will also explore high-dimensional models, he will suggest that the best trade-off between interpretability and performance of the models is achieved when a small set of variables is selected by domain experts. ![]() Dr Andreas Joseph, Bank of England, UK
![]() Dr Andreas Joseph, Bank of England, UKAndreas received his PhD from City University of Hong Kong in 2014 working on international trade and financial networks. He then briefly moved to the economics department of the European Central Bank before arriving at the Bank of England’s Advanced Analytics division in 2015. Since then, Andreas mostly worked on the development and use of machine and deep learning techniques in an economic policy context, such as model inference and interpretability, and more recently reinforcement learning in macroeconomics. |
09:30-09:40 |
Discussion
|
09:40-10:05 |
Persons, situations, and time: personalised behavioural forecasting
A longstanding goal of psychology is to predict or forecast the things people do and feel. Despite this, tools to accurately predict future behaviours and experiences have remained elusive. Dr Beck’s work indicates that one reason behavioural forecasting models may fail is the assumption that people should share psychological and situational antecedents of the same behaviours and experiences. In this talk, Dr Beck will present research from two different studies of younger and older adults to highlight the utility of person-specific machine learning methods. Using intensive longitudinal data and three machine learning forecasting approaches, she investigated the degree to which several behaviours and experiences (eg, loneliness, procrastination, physical pain) could be predicted from past psychological (ie personality and affective states), situational (ie objective situations and psychological situation cues), and time (ie trends, diurnal cycles, time of day, and day of the week) phenomena from a person-specific perspective. Rather than pitting persons against situations, such an approach allows psychological phenomena, situations, and time to jointly predict future behaviour and experiences. Dr Beck found (1) a striking degree of prediction accuracy across participants, (2) that most participants’ future behaviours are predicted by both person and situation features, and (3) that the most important features vary greatly across people. She concluded with future directions and challenges in psychological and behavioural forecasting. ![]() Dr Emorie D Beck, University of California, Davis, USA
![]() Dr Emorie D Beck, University of California, Davis, USADr Emorie Beck is an Assistant Professor of Personality and Individual Differences at the University of California, Davis. She received her PhD (2020) and MA (2019) from Washington University in St. Louis and her BA (with honours; 2016) from Brown University. Dr Beck’s research focuses on what personality is, which has consequences for how we measure personality, what those measures predict both short- and long-term, how personality is thought to change. She studies how to understand the personality of an individual relative to only themself, relative to some others, and relative to all others. To do so, she uses a mix of methods, including experience sampling methods, passive sensing, survey data, panel data, cognitive tests, and more measured across time intervals from moments to years along with an array of statistical approaches, including time series analysis and forecasting, hierarchical modeling, machine learning, network psychometrics, structural equation modeling, and more. |
10:05-10:15 |
Discussion
|
10:15-10:45 |
Break
|
10:45-11:10 |
Application and validation of forecasting principles for election forecasting
PollyVote.com was launched in 2004 as a long-term project to demonstrate the value of forecasting principles, derived from the general forecasting literature, by applying them to the high-profile task of forecasting major elections in the United States, Germany, and France. As part of this project, Professor Graefe provided empirical evidence on the relative predictive accuracy of different forecasting methods (ie, intention-based polling, expectations-based judgment, and model-based forecasts). The evidence shows that a method’s past accuracy is a poor predictor of future accuracy, and that it is difficult to assess the direction of forecasting error a priori, let alone identify the most accurate forecast prior to the event. The data further shows that combining forecasts across different methods that incorporate different information yields substantial gains in accuracy and avoids large errors. Many of the lessons learned from nearly twenty years of election forecasting have implications for improving forecasting in other domains. ![]() Professor Andreas Graefe, Macromedia University, Munich, Germany
![]() Professor Andreas Graefe, Macromedia University, Munich, GermanyDr Andreas Graefe is professor in the Faculty of Business at Macromedia University of Applied Sciences, Munich, Germany. Prior to that, he worked at Karlsuhe Intitute of Technology and LMU Munich, and was a visiting scholar at the University of Pennsylvania and University of Columbia. His research interests are forecasting, with a particular focus on political forecasting, decision-making, and algorithms. He is responsible for the PollyVote.com project for forecasting elections in different countries and currently serves as chair of APSA’s Political Forecasting Group. |
11:10-11:20 |
Discussion
|
11:20-11:45 |
Translating climate forecasts into models for humanitarian anticipatory action
In the past few years, humanitarian organisations have been exploring the potentials of anticipatory action, where scientific forecasts are used to trigger pre-agreed financing and action ahead of devastating climate shocks from floods to drought. These frameworks build upon existing climate models, such as seasonal tercile forecasts or global flood models, to predict when the worst shocks are expected to occur and act accordingly. However, applying models of climate phenomena with varying forecast outputs, lead times, uncertainty, or forecasting periods to forecast humanitarian impact presents numerous hurdles. The UN OCHA Centre for Humanitarian has been working to understand and overcome these challenges in our anticipatory action pilots. Through the collection of impact data or proxies, the validation of climate models’ performance in predicting impacts, and the development of simple frameworks around these models for decision makers, the Centre is developing or has implemented pilots in 14 countries for multiple different shocks. This talk will give an overview of the Centre’s approach in these pilots to translate climate science into actionable analysis for humanitarian decision makers. It will particularly cover the technical issues faced when validating model performance and how this performance and the uncertainty around it is communicated to decision makers. It will finish with a discussion on the open questions the Centre is currently working on as the humanitarian anticipatory action agenda continues to grow. ![]() Mr Seth Caldwell, United Nations, USA
![]() Mr Seth Caldwell, United Nations, USAWith over 7 years of experience in data and analytics in humanitarian response and a masters in analytics, Seth Caldwell is a data scientist at the UN Centre for Humanitarian Data. He and the rest of the predictive analytics team develop triggers and manage the technical work for OCHA’s anticipatory pilots on drought, floods, and other shocks. In the past year, Seth has developed drought prediction frameworks in the Sahel, applied flood forecast models in South Sudan, and led the Centre’s work in exploring the potential of conflict prediction models for anticipatory humanitarian action. |
11:45-11:55 |
Discussion
|
11:55-12:20 |
How to improve the accuracy of human forecasts for geopolitical events
People often make mistakes, sometimes quite serious, when they try to predict the future. Examples include international politics, financial investments, public policies, and medical diagnoses. Past psychological research shows that human predictions are often worse than very simple mathematical rules or algorithms, although predictions based on more sophisticated techniques are now gaining some traction. The Intelligence Community needed predictions of global events, so IARPA (the research wing of the US Intelligence Community) conducted a series of geopolitical forecasting tournaments in which the team at the University of Pennsylvania competed with other university teams. Questions covered a wide range of topics, including events the Syrian civil war, the Russian invasion in Crimea and whether French or Swiss inquiries would find elevated polonium in Yasser Arafat's body. IARPA defined predictive success as the Brier scoring rule. All the teams, using the same scoring rule, were given the same questions during the same period, so science could proceed at a rapid pace. The team at the University of Pennsylvania won the tournaments by a wide margin each year for four years. Five drivers accounted for our success. They included: identifying forecasting talent; training forecasters in probabilistic reasoning; placing forecasters in teams rather than having them work alone; tracking them by putting the best forecasters on the same teams; and aggregating forecasts using simple new algorithms. Human forecasts could be predicted with a surprising degree of accuracy when predictions were viewed from multiple perspectives, including psychological, economic, political, and statistical standpoints. ![]() Professor Barbara Mellers, University of Pennsylvania, USA
![]() Professor Barbara Mellers, University of Pennsylvania, USABarbara Mellers is a cognitive psychologist at the University of Pennsylvania who studies how people make judgments and decisions. She has developed descriptive models of factors that influence human decisions but are not incorporated into classical economic models. These ‘nuisance’ variables include the context for a judgment (or the distribution of surrounding stimuli), the response mode (or how the question is asked), anticipated emotions about the outcomes of decisions, and human tendencies to cooperate despite opportunities to engage in self-interest. In the last few decades, Barbara has focused primarily on how to improve human judgments and decisions. For example, she has worked with friends and colleagues on ways to increase the accuracy of human forecasts of geopolitical events using large-scale field experiments with randomised control trials. She, and others, have tested the effectiveness of elicitation methods (i.e. prediction polls vs prediction markets), probability training techniques, team interactions and aggregation algorithms. Improving the accuracy of human forecasts is a critical step toward improving the overall quality of judgments and decisions. |
12:20-12:30 |
Discussion
|
Chair
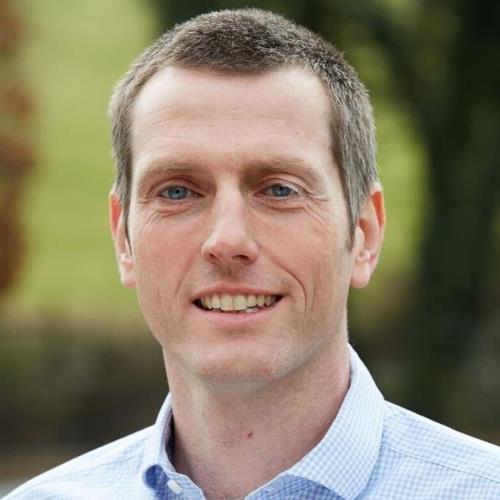
Professor Steven Riley, UK Health Security Agency, United Kingdom
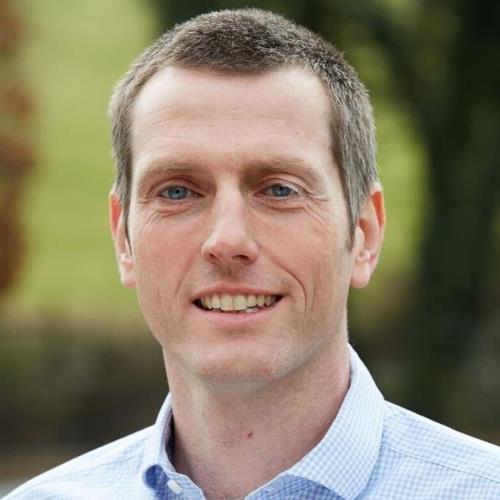
Professor Steven Riley, UK Health Security Agency, United Kingdom
Steven is an infectious disease scientist currently working in the UK Health Security Agency (UKHSA). His group leads on improving how data, analytics and surveillance are used in the agency. Outside of UKHSA his personal research interests are in the use of models, epi and field work to better understand respiratory viruses. During the COVID-19 pandemic he was part of the Imperial College COVID-19 Response Team, the Scientific Pandemic Influenza - Modelling committee (SPI-M) and the REal-time Assessment of Community Transmission (REACT) team.
13:30-13:55 |
Forecasting in demography
Forecasting exercises in demography attempt to answer questions related to human populations. Example questions range from big general ones such as “How many people will there be globally in 2100?” to questions that are focused on smaller populations and specific indicators like “What will be the change in adolescent birth rates in a specific subnational area between now and 2030?”. Often, due to data limitations, nowcasting of demographic indicators is necessary for producing forecasts, and estimates of current levels and past trends are of interest in their own right. Examples include estimation of abortion rates currently in countries where abortions are illegal, or the assessment of past trends in maternal mortality in low-income countries without well-functioning registration systems. Typically, uncertainty associated with past trends and future predictions is substantial and needs to be taken into account when interpreting results. In this talk, Professor Alkema will introduce the use of Bayesian statistical modelling approaches to nowcasting and forecasting in demography. Illustrated by examples in the area of family planning, reproductive health, and fertility, she will present general modelling challenges for producing nowcasts and forecasts, and common features of Bayesian statistical models that overcome these challenges. Professor Alkema will end with some comments related to ongoing and future research in statistical demography to improve the quality and utility of model-based nowcasts and forecasts. ![]() Professor Leontine Alkema, University of Massachusetts, USA
![]() Professor Leontine Alkema, University of Massachusetts, USALeontine Alkema, PhD, is Professor of Biostatistics at the University of Massachusetts Amherst, United States of America. Dr. Alkema’s research focuses on the development of statistical models to assess trends and differentials in reproductive, maternal, and child health outcomes worldwide. She has developed estimation and projection methods for key global indicators, such as the under-five mortality rate, maternal mortality, usage of and unmet need for contraceptive methods, abortion incidence, and total fertility. She collaborates with various United Nations agencies, the World Health Organization, the FP2030 community, and Guttmacher Institute to help make available improved estimation methods, software and resulting model-based estimates to diverse international audiences. |
---|---|
13:55-14:05 |
Discussion
|
14:05-14:30 |
Flood forecasting and its impact on making decisions that reduce risks
Floods are major natural disasters that can be responsible for life losses and severe economic damages. Flood forecasting and early warning systems are needed to anticipate the arrival of these events and mitigate their impacts. Flood impacts are in great part related to changes in societal vulnerability, such as increased exposure to extreme events and increased density of risk-prone urbanised areas. Climate change is also likely playing a role in increasing flood risk, by exacerbating the frequency and intensity of extreme rainfall events. When forecasting floods, these two factors must be considered: the weather, and the characteristics of the river basin. Understanding and tackling the many uncertainties that affect our ability to foresee when and where a flood event will occur, and how strong it will be, from the atmospheric to the land and river conditions, is important to inform decision-makers, civil protection authorities and the population at risk. We often assume that supplied with information, people will take better risk-based decisions. However, the efficient communication of uncertain flood forecasts also requires understanding how forecast information is perceived and used in real time. New technologies and data have been increasingly used to achieve more accurate, timely and reliable flood forecasts and better predict their impacts. Lessons learned from past flood events have shown that we can still improve our ability to communicate and act based on uncertain flood forecasts, by increasing anticipation and confidence in the forecasts. ![]() Dr Maria-Helena Ramos, INRAE, France
![]() Dr Maria-Helena Ramos, INRAE, FranceMaria-Helena Ramos is a research scientist in hydrology and hydrometeorology. She was awarded a BSc in Civil engineering in 1993 and an MSc in Environment and water resources in 1998 in Brazil. In France, she was awarded a PhD in Atmospheric and Earth Sciences in 2002. She carried out research on hydrometeorological forecasting at the Joint Research Center of the European Commission in Italy, working with the European Flood Awareness System, before joining INRAE in France in 2007. Her research focuses on methods to improve flood and drought forecasting, to communicate forecasts and to inform decision making in water resources management under present and future climate conditions. She is currently the president of the Hydrological Sciences Division of the European Geosciences Union (EGU), member of the Scientific Steering Committee of the World Weather Research Programme (WMO), and a Fellow of the European Centre for Medium-range Weather Forecasts (ECMWF). |
14:30-14:40 |
Discussion
|
14:40-15:10 |
Break
|
15:10-15:35 |
The science of earthquake forecasting
Earthquakes are emergent phenomena, arising in complex fault systems where tectonic stresses cause spontaneous ruptures across a vast range of spatiotemporal scales. The complexity of such systems requires that forecasting models treat seismic activity as a stochastic process. An earthquake forecast estimates the probability that a future earthquake greater than a certain magnitude will occur in specified space-time window. The most common type of forecast, widely used in seismic hazard analysis, is the time-independent Poisson model, which assumes earthquakes occur randomly in time with spatially variable magnitude-frequency distributions but stationary rates. Forecasts can be improved by introducing time-dependent probabilities that account past seismic activity. On long time scales of decades to centuries, earthquake activity is modulated by the accumulation and release of stress on major faults, which can be modelled as a Reid renewal process. On short time scales of days to months, earthquake sequences show clustering in space and time, as indicated by aftershock activity, which can be described by a Hawkes self-exciting process. Long-term forecasts, such as the US National Seismic Hazard Model, are currently the most important forecasting tools for civil protection against earthquake damage, because they guide the seismic safety provisions of building codes and performance-based design. Properly applied, short-term forecasts have operational utility in anticipating the increased seismicity that follows large earthquakes; however, while the short-term probabilities of large, potentially damaging earthquakes can vary over several orders of magnitude, they typically remain low in an absolute sense (< 1% per day). Translating such low-probability forecasts into effective decision-making is a difficult challenge. ![]() Professor Thomas Jordan, University of Southern California, USA
![]() Professor Thomas Jordan, University of Southern California, USAThomas H. Jordan is a University Professor at the University of Southern California and holds the W. M. Keck Foundation Chair of Earth Sciences. His current research is focused on system-level models of earthquake processes, earthquake forecasting, and structural seismology. He received his PhD from the Caltech in 1972, was head of MIT’s Department of Earth, Atmospheric and Planetary Sciences from 1988 to 1998, and moved from MIT to USC in 2000. From 2002 to 2017, he was the director of the Southern California Earthquake Center, where he coordinated an international research program in earthquake system science. He is a member of the US National Academy of Sciences. |
15:35-15:45 |
Discussion
|
15:45-16:10 |
Wildland fire prediction
Wildland fires are dynamic, complex events that generate transient phenomena including fire whirls, blow-ups, bursts of flame ahead of the fire line, deep thunderstorms, and wind speeds among atmospheric extremes. Public fire spread predictions are not yet a standard forecast product and, despite emergency alert systems and improving weather forecasts, communities may be tragically unprepared for complex and explosive fire behaviour. Fire behaviour models have been developed and applied since the 1970s. Early models – steady-state kinematic formulae or empirical relationships based on theory, laboratory fire beds, or small-scale prescribed fire experiments – were primarily used to estimate a fire’s rate of expansion. Ad hoc calibration prolonged their use though recent years. As complexities of wildfire behaviour became recognised, models evolved from simple algorithms to computational fluid dynamics modelling systems, where fluid or weather models are coupled to algorithms parameterising fire behaviour or combustion processes. Compared to legacy tools, computational modelling systems increase cost and complexity but have produced breakthrough understanding of the mechanisms underlying outlier wildfire events such as fine-scale extreme winds that can draw ignitions from the electric grid and the production of fire-induced winds. When combined with remotely sensed active fire detection data, coupled weather-fire models have been configured to not only forecast a fire’s growth but expand our ability to anticipate when fires may bifurcate, merge, or change directions, and currently broach prediction of phenomena like large firenadoes. Coen will discuss case studies of recent extreme events and the challenges and limitations in our remote sensing systems, fire prediction tools, and meteorological models that add to wildfires’ mystery and apparent unpredictability. ![]() Dr Janice Coen, National Center for Atmospheric Research, USA
![]() Dr Janice Coen, National Center for Atmospheric Research, USADr Janice Coen holds positions of Project Scientist in the Mesoscale and Microscale Meteorology Laboratory at the National Center for Atmospheric Research in Boulder, Colorado, and Senior Research Scientist at the University of San Francisco in San Francisco, California. She received a BSc in Engineering Physics from Grove City College and an MSc and PhD from the Department of Geophysical Sciences at the University of Chicago. She studies fire behaviour and its interaction with weather using coupled weather-fire CFD models and flow analysis of high-speed IR fire imagery. Her recent work investigated the mechanisms leading to extreme wildfire events, fine-scale wind extrema that lead to ignitions by the electric grid, and integration of coupled models with satellite active fire detection data to forecast the growth of landscape-scale wildfires. |
16:10-16:20 |
Discussion
|
16:20-16:45 |
Using physical modelling to assess natural hazard risks
Most extant assessments of long-term risks of weather hazards rely mostly or entirely on historical records. In many cases, such records are too short to capture the all-important tail risks and are often seriously flawed owing to inadequate measurements. Moreover, even if there were excellent and long records of weather hazards, the application of such records to assessment of current risks would be seriously compromised by climate change that has already occurred. For this reason, Emanuel advocates for the application of computational weather models to long term risks and, in his talk, will discuss such an application to the specific risks posed by tropical cyclones. The main challenge posed by the application of computational models to long-term risk is the need of enough computer power to simultaneously resolve the phenomena in question and run the model for approximately 1,000 years of simulated time to define the all-important 100-year events. Emanuel will discuss how this challenge can be addressed through a deep understanding of the physics of the weather hazards. ![]() Emeritus Professor Kerry Emanuel ForMemRS, Massachusetts Institute of Technology, USA
![]() Emeritus Professor Kerry Emanuel ForMemRS, Massachusetts Institute of Technology, USADr Kerry Emanuel is the Cecil and Ida Green emeritus professor of atmospheric science at the Massachusetts Institute of Technology, where he was on the faculty from 1981 to 2022. Before that he was on the faculty of UCLA from 1978 to 1981. Emanuel’s initial focus was on the dynamics of rain and snow banding in winter storms, but his interests gradually migrated to the meteorology of the tropics and to climate change. His specialty is hurricane physics and he was the first to investigate how long-term climate change might affect hurricane activity, an issue that continues to occupy him today. His interests also include cumulus convection, and advanced methods of sampling the atmosphere in aid of numerical weather prediction. Emanuel is the author or co-author of over 200 peer-reviewed scientific papers, and three books, including Divine Wind: The History and Science of Hurricanes, published by Oxford University Press and aimed at a general audience, and What We Know about Climate Change, published by the MIT Press and now entering its third edition. He was a co-founder and co-director of MIT’s Lorenz Center, a climate think tank devoted to basic, curiosity-driven climate research. |
16:45-17:00 |
Discussion
|
Chair
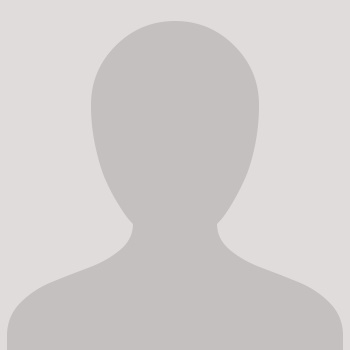
Dr Nicholas Reich, Department of Biostatistics and Epidemiology University of Massachusetts - Amherst, USA
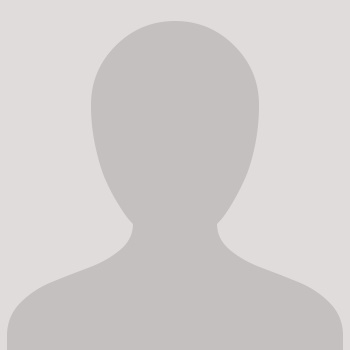
Dr Nicholas Reich, Department of Biostatistics and Epidemiology University of Massachusetts - Amherst, USA
09:00-09:25 |
The power of collaborative hubs for infectious disease
Drawing from her experience with the Ebola Forecasting Challenge and the COVID19 Scenario Modeling Hub, Dr Viboud will illustrate how collaborative and coordinated modeling hubs can help guide the response to infectious disease crises. She will also reflect on differences in the application and evaluation of short-term forecasts and long-term scenario projections. The Ebola Forecasting Challenge was inspired by the West African Ebola crisis in 2014-2015 and was the first synthetic challenge applied to infectious disease. The challenge ran during 2016-2017 and involved 16 international academic teams and US government agencies; the goals were to compare the predictive performance of 8 independent modeling approaches under different levels of interventions and "fog of war" in outbreak data. The COVID-19 Scenario Modeling Hub was created in December 2020 to provide multiple rounds of real-time, long-term scenario projections in the US for health authorities and the public. Notably, scenario hub projections were used to guide the expansion of the primary COVID-19 vaccine schedule to school-age children in 2021, and booster recommendations in autumn 2022. In August 2022, she helped to launch the companion Flu Scenario Modeling Hub. ![]() Dr Cecile Viboud, National Institutes of Health, USA
![]() Dr Cecile Viboud, National Institutes of Health, USACecile Viboud is a senior research scientist in the Division of International Epidemiology and Population Studies of the Fogarty International Center, National Institutes of Health, USA. Her research focuses on the epidemiology and transmission dynamics of acute viral infections, at the interface of public health and computational modeling. Her work has concentrated on modeling the epidemiology, excess mortality, and transmission dynamics of respiratory viruses, including influenza and COVID-19. Her most recent work has been focused on scenario planning for influenza and COVID-19, and evaluation of vaccination programs. |
---|---|
09:25-09:35 |
Discussion
|
09:35-10:00 |
Forecasting ecology in a changing world
What is ecological forecasting and why do it? This talk will introduce the idea of near-term iterative ecological forecasting, which is the process of making probabilistic, out-of-sample predictions of ecological processes and then testing and updating those predictions as new information becomes available. Professor Dietze will then explain how this approach is a potential win-win for accelerating science, addressing discipline-spanning questions about ecological predictability, and making ecology both more robust and more directly relevant to societal needs. He will discuss the challenges and opportunities in iterative ecological forecasting and highlight ongoing efforts to build an ecological forecasting community of practice. He will draw on examples from a wide range of ecological processes, including carbon monitoring, land-surface fluxes, the soil microbiome, vegetation phenology, freshwater resources, and tick-borne disease. Finally, Professor Dietze will touch on the efforts of the Ecological Forecasting Initiative (EFI) to build a community of practice and EFI’s ongoing NEON forecasting challenge. ![]() Professor Michael Dietze, Boston University, USA
![]() Professor Michael Dietze, Boston University, USAMichael Dietze is professor of Earth & Environment at Boston University. He is author of the book, “Ecological Forecasting” and founding director of the Ecological Forecasting Initiative (EFI, https://ecoforecast.org), an international grassroots research consortium aimed at fostering a community of practice around near-term ecological forecasting. His lab uses a combination of field research, remote sensing, novel statistical methods, numerical models, and ecoinformatics tools to gain a quantitative understanding of ecological dynamics across scales from the individual to the globe. |
10:00-10:10 |
Discussion
|
10:10-10:40 |
Break
|
10:40-11:05 |
Evaluation and communication of climate-sensitive disease risk forecasts
Extreme climatic events, such as tropical storms, floods, and droughts, can impact the timing and intensity of climate-sensitive disease outbreaks, including dengue, malaria, and leptospirosis. For example, Aedes mosquitoes vector thrives in warm and humid conditions with rainfall increasing the number of outdoor breeding sites. However, drought conditions can also promote breeding, due to an increase in water storage containers in and around the home. In this session, Professor Lowe will present a Bayesian mixed-model framework, which quantifies the extent to which climatic, environmental, and socio-economic indicators explain spatial and interannual variations in disease risk. The framework is designed to provide probabilistic predictions of monthly disease incidence and the probability of exceeding outbreak thresholds, which are determined in consultation with public health stakeholders. This disease model framework, combined with seasonal climate forecasts, has been successfully applied to produce real-time probabilistic dengue early warnings in Brazil, southern coastal Ecuador, and Vietnam. The model framework was recently extended to account for delayed and nonlinear impacts of drought and extreme rainfall on dengue risk in Barbados and across Brazil and Vietnam, showing that the impact of drought is exacerbated in urban areas due to interrupted water supply. Incorporating seasonal climate forecasts in disease early warning systems can trigger action plans to support public health decision-makers in targeting timely disease control and prevention strategies months in advance, to mitigate the risk of imminent disease epidemics and emerging disease threats. ![]() Professor Rachel Lowe, ICREA/Barcelona Supercomputing Center, Spain
![]() Professor Rachel Lowe, ICREA/Barcelona Supercomputing Center, SpainRachel Lowe is an ICREA Research Professor and Global Health Resilience Team Leader at the Barcelona Supercomputing Center. She also holds a Royal Society Dorothy Hodgkin Fellowship at the London School of Hygiene & Tropical Medicine. Rachel’s research focuses on co-developing policy-relevant methodological solutions, to enhance surveillance, preparedness and response to climate-sensitive disease outbreaks and emergence. She has published high impact research on integrating seasonal climate forecasts in early warning systems for infectious diseases in Latin America, the Caribbean and Southeast Asia. Rachel is the Director of the Lancet Countdown on health and climate change in Europe. She is a member of the World Meteorological Organization COVID-19 research task team and was a contributing author of the IPCC Sixth Assessment Report (WGII) chapter on risks across sectors and regions. |
11:05-11:15 |
Discussion
|
11:15-11:40 |
Predictability of influenza virus evolution
Seasonal influenza viruses repeatedly infect humans in part because they rapidly change their antigenic properties and evade host immune responses, necessitating frequent updates of the vaccine composition. Accurate predictions of strains circulating in the future could therefore improve the vaccine match and predicting influenza virus evolution has been an active area of research over the past decades. However, accurate predictions with time horizons beyond 6 months have remained elusive. I will discuss these challenges and the underlying features of influenza immunology and evolution that limit predictability. Similar challenges will likely emerge when predicting the success of SARS-CoV-2 variants. ![]() Professor Richard Neher, University of Basel, Switzerland
![]() Professor Richard Neher, University of Basel, SwitzerlandRichard Neher studied physics in Munich, Germany, and spent 3 years as a post-doctoral fellow at KITP. In 2010, he became Research Group Leader at the Max Planck Institute in Tübingen, Germany, and in 2017 Associate Professor at the University of Basel, Switzerland. Richard Neher studies the evolution of RNA viruses, including HIV, influenza virus, and SARS-CoV-2. He is co-founder of Nextstrain, a pioneering platform to track the evolution of viruses in real time. |
11:40-11:50 |
Discussion
|
11:50-12:15 |
Forecasting bird migration for science and conservation
Billions of birds cross the globe each year during seasonal migrations. Migratory birds face an increasing array of challenges in our modern world, and their populations have declined precipitously in the last half-century. In the United States, forecasts of bird migration are becoming important tools for ameliorating direct sources of mortality (eg, bird-building collisions), directing science, engaging stakeholders, and raising public awareness. Migration forecasts are based on machine learning models that use atmospheric conditions to predict the intensity of nightly migratory flights. These models are trained on over two decades of observational data from a large network of meteorological radars, which can detect large-scale bird migration. Forecast performance degrades with increasing time horizon, but migration forecasts are effective several days in advance. Through the BirdCast website, we provide daily continental forecasts and targeted local alerts. An important application of migration forecasts is the selective reduction of light pollution during nights with intense bird migration, as light pollution attracts migrating birds and greatly increases collision risk. A variety of ‘lights-out’ coalitions across the US utilise migration forecasts to make decisions about when to turn off lights to protect migrating birds. Future work on will focus on improving the temporal and spatial resolution of predictions, incorporating birds’ stopover behaviour, and tuning predictions for particular use cases (eg, directly forecasting collision risk). ![]() Dr Benjamin Van Doren, Cornell University, USA
![]() Dr Benjamin Van Doren, Cornell University, USABenjamin Van Doren studies the eco-evolutionary responses of migratory birds to environmental change. His research spans spatial and population scales and unites ecology, evolution, behaviour, and conservation. Benjamin earned a DPhil in Zoology from the University of Oxford, and he has received achievement awards from the Linnean Society of London, Zoological Society of London, and the American Ornithological Society. As a Presidential Postdoctoral Fellow at the Cornell Lab of Ornithology, he explores how climate change, light pollution, and human-dominated landscapes influence migratory bird ecology and behaviour. |
12:15-12:30 |
Discussion
|
Chair
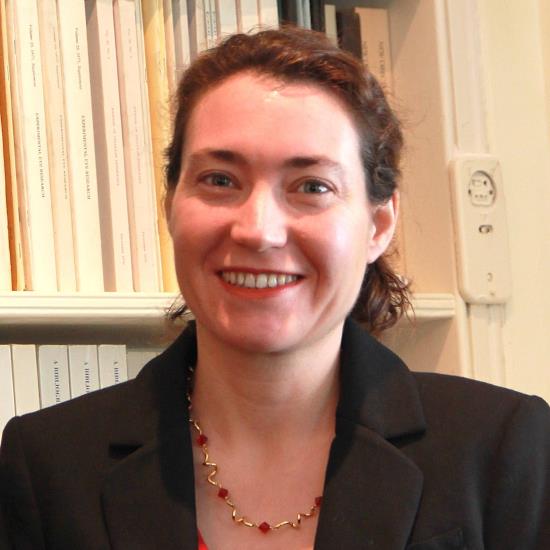
Dr Cecile Viboud, National Institutes of Health, USA
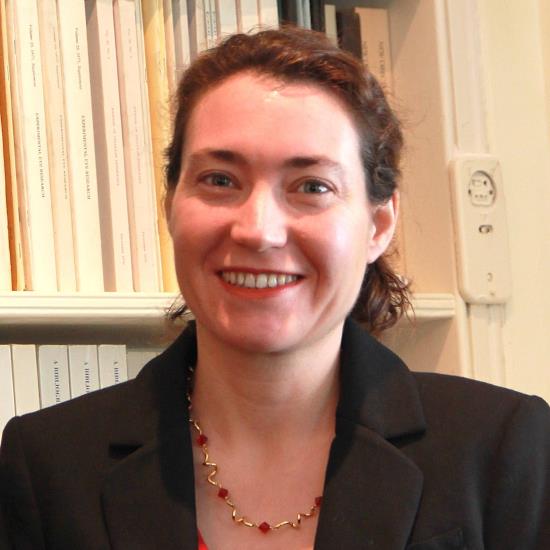
Dr Cecile Viboud, National Institutes of Health, USA
Cecile Viboud is a senior research scientist in the Division of International Epidemiology and Population Studies of the Fogarty International Center, National Institutes of Health, USA. Her research focuses on the epidemiology and transmission dynamics of acute viral infections, at the interface of public health and computational modeling. Her work has concentrated on modeling the epidemiology, excess mortality, and transmission dynamics of respiratory viruses, including influenza and COVID-19. Her most recent work has been focused on scenario planning for influenza and COVID-19, and evaluation of vaccination programs.
13:30-13:55 |
From weather to climate predictions
The changing nature of weather and climate has increased the need for predictive thinking in the assessment and handling of climate risk. In this talk, Dr Thorarinsdottir will discuss some contributions that statistics and machine learning provide to the assessment of near-future climate risk with applications in a range of sectors. She will, in particular, focus on the predictability of weather and climate, efforts to push the limits of climate prediction, the importance of uncertainty quantification and model evaluation. ![]() Dr Thordis Thorarinsdottir, Norwegian Computing Center, Norway
![]() Dr Thordis Thorarinsdottir, Norwegian Computing Center, NorwayThordis Thorarinsdottir is a statistician and chief research scientist at the Norwegian Computing Center in Oslo, Norway. She is also a co-leader of Climate Futures, a center for research-based innovation focusing on developing climate predictions to handle climate risk that launched in October 2020. In her research, she develops stochastic models and methods for the environmental sciences, with a special emphasis on uncertainty quantification. |
---|---|
13:55-14:05 |
Discussion
|
14:05-14:30 |
From weather to climate forecasting
Weather forecasts are issued routinely by national meteorological agencies around the world and checking the latest forecast on our favourite apps is for many of us a part of daily life. Dr Weisheimer has the privilege to jointly work in a world-leading operation weather prediction centre and carry out research on the predictability of our weather and climate from an academic perspective. In this talk, she will give a brief conceptual overview of how the forecasts that we see in public are produced, how quantitative statements including probabilities are derived, and how weather forecasts are evaluated under critical scrutiny on a constant basis using a wide range of quantifiable performance measures for skill and reliability. From there, Dr Weisheimer will introduce the concept of seamless weather and climate prediction that leads us to seasonal forecasts, i.e., predictions of the climate system for the next winter and summer. The scientific foundations for predictions over such long-time horizons will be explained, typical forecast products presented, and recent examples given. Historical retrospective forecasts where the observed outcome is known are used to calibrate real-time forecasts and provide crucial data sets for skill evaluation. In the last part of the talk, challenges for improving confidence in climate forecasts will be addressed. These originate from deficiencies in the realism of our physical forecast models to represent the full complexity of the system, but are also related to non-stationarity and signal-to-noise characteristics of the climatic states. ![]() Dr Antje Weisheimer, University of Oxford & European Centre for Medium-Range Weather Forecasts (ECMWF), UK
![]() Dr Antje Weisheimer, University of Oxford & European Centre for Medium-Range Weather Forecasts (ECMWF), UKAntje Weisheimer is a senior research fellow at the Physics Department at the University of Oxford where she leads the Predictability of Weather and Climate group. She holds a joined appointment as a senior scientist in the Research Department of the European Centre for Medium-Range Weather Forecasts (ECMWF) where her work is focused on seasonal predictions. Antje graduated from Humboldt University in Berlin, received her PhD in Atmospheric Physics from the University of Potsdam, and took a fellowship at the London School of Economics and Political Science. She’s an Associate Editor for the Quarterly Journal of the Royal Meteorological Society and serves as a member in the World Climate Research Programme Lighthouse Activity on Explaining and Predicting Earth System Change. In recognition of her research on climate dynamics and seasonal predictability, she was awarded with the Buchan Prize of the Royal Meteorological Society in 2021. |
14:30-14:40 |
Discussion
|
14:40-15:10 |
Break
|
15:10-15:35 |
Building and improving VIEWS, a political violence early-warning system
The Violence Early-Warning System (VIEWS) seeks to forecast the number of fatalities armed conflicts in Africa and the Middle East, at the country level as well as for a 0.5 x 0.5-degree geographical grid. Professor Hegre will present how VIEWS is constructed, sketching the main methodological choices, summarise its performance, and survey the main challenges ahead. VIEWS performs well for the established conflict situations that account for the vast majority of deaths in armed conflict. In order to improve further, he will argue that armed conflict forecasts must strengthen its handling of uncertainty. In addition to discussing the uncertainty at the core of violent decision-making itself, Professor Hegre will discuss a number of more technical sources, such as imprecision in what we know about armed conflicts in the past and other core predictors, statistical uncertainty, uncertainty about what are the best model specifications, and uncertainty in the metrics we use to evaluate, select, and weight models. ![]() Professor Håvard Hegre, Uppsala University, Sweden
![]() Professor Håvard Hegre, Uppsala University, SwedenHåvard Hegre is Research Professor at Peace Research Institute Oslo (PRIO) and at the Department of Peace and Conflict Research at Uppsala University. His research focuses on forecasting of political events and their impacts, as well as the relationships between democracy, development and armed conflict. The research has been funded by the European Research Council (ERC), the Research Council of Norway, Riksbankens Jubileumsfond, the Swedish Research Council, the World Bank, the UN, and other funders. From 2017 to 2021, he developed an early-warning system for political violence. Hegre is currently extending this system with funding from ERC and Riksbankens Jubileumsfond. He is on the editorial board or associate editor of Journal of Peace Research, International Studies Review, and Research and Politics. |
15:35-15:45 |
Discussion
|
15:45-16:10 |
Physcially-based probability forecasting of weather and applications in social science
Weather forecasting is based on physical science, using a numerical model of the atmosphere, initiated using the latest observations, to simulate the future evolution of the atmosphere and produce a forecast. Uncertainty is inherent to the process, not just because of the complexity of the system, but because the atmosphere is a chaotic system. Chaos places fundamental limits on predictability. Ensemble forecasting, using multiple forecasts from perturbed initial states, has been developed to extract the maximum predictability amidst the chaos, to understand the uncertainty in each forecast and generate probabilistic forecast information. Ultimately, however, weather forecasts are of no use to society unless they are used to drive decision-making. This requires social science techniques to communicate the forecast to the public and decision-makers in ways which make the physical science relevant, supporting a risk-based decision approach. This has led to the development of a Value Chain approach to the development of forecast services which is now widely used around the world, in which the physical forecasting of the weather and its uncertainty is only the first step. A good example is the UK National Severe Weather Warning Service which is based on a risk matrix depending on both the potential level of societal impact expected from an event and the likelihood of those impacts occurring. Timeliness and effective communication of the warning message are also vital if the warning is to be acted upon and society and assets protected from the impacts of the weather. ![]() Mr Ken Mylne, Met Office
![]() Mr Ken Mylne, Met OfficeKen Mylne joined the Met Office from Oxford University in 1984 and initially conducted experimental research on pollution dispersion in the turbulent boundary layer. He then spent 6 years as a weather forecaster issuing aviation and shipping forecasts and warnings, before returning to research in ensemble forecasting, a technique used to understand the uncertainty in the weather forecast. He led the development of the Met Office's own ensemble prediction system, MOGREPS, which is now one of the world's leading operational ensemble forecasting systems. More recently he has focused more on the application of ensembles and integrating their use fully into the Met Office's operational production procedures, and in propagating the uncertainty in the weather forecast into an understanding of the impact on end-users to enable those users to make effective decisions in the face of uncertainty. Ken has been chair of the World Meteorological Organisation's Expert Team on Ensemble Prediction for the past 8 years. |
16:10-16:20 |
Discussion
|
16:20-17:00 |
Panel discussion
|