Forecasting infectious disease incidence for public health
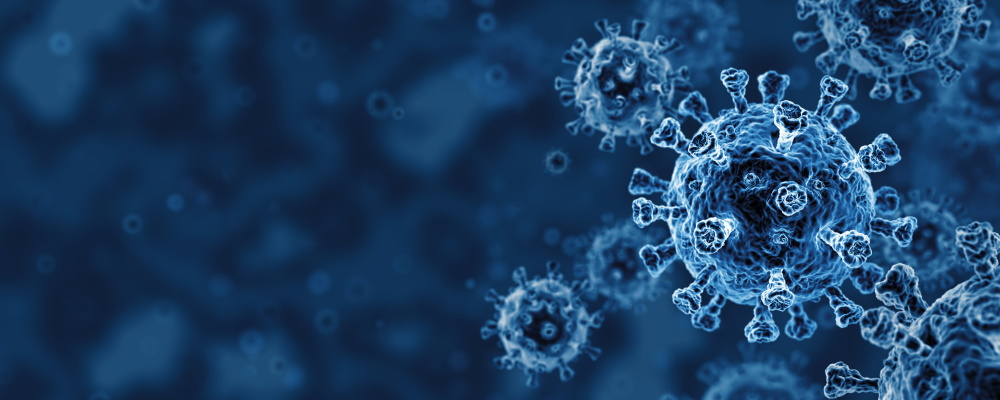
Satellite meeting organised by Professor Sebastian Funk, Professor Nicholas Reich, Professor Steven Riley and Professor Rachel Lowe.
Forecasts have rarely been the focus when analysing and modelling infectious disease dynamics. Recently they have become a topic of increasing activity. The meeting discussed the latest developments in this area and identify cross-cutting areas and synergies with other fields.
The schedule of talks, speaker biographies and abstracts are available below.
Attending this event
This event has now taken place.
Enquiries: contact the Scientific Programmes team
Organisers
Schedule
Chair
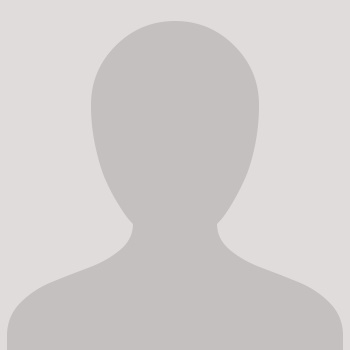
Dr Nicholas Reich, Department of Biostatistics and Epidemiology University of Massachusetts - Amherst, USA
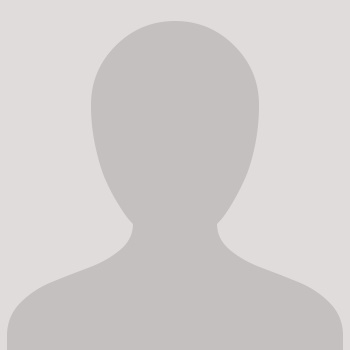
Dr Nicholas Reich, Department of Biostatistics and Epidemiology University of Massachusetts - Amherst, USA
09:00-09:10 |
Introduction
|
---|---|
09:10-09:25 |
Discussion: Overview of the main meeting
|
09:25-09:45 |
Parsimonious models for βt and the consequences for forecasting
Throughout the course of the SARS-CoV-2 pandemic Dr Birrell and his team fulfilled a requirement both from within the UK Health Security Agency (including Public Health England) and externally through SPI-M, to produce pandemic nowcasts and medium-term ‘projections’ in real-time. This was achieved through the PHE-Cambridge real-time model (RTM), a deterministic compartmental model designed to assimilate an array of pandemic data streams to produce epidemic nowcasts and forecasts, whilst estimating key epidemic quantities. Such models need to accurately capture fluctuations in transmission arising from external determinants (eg changing government advice, the emergence of new variants). Ignoring this “environmental stochasticity" can lead to mis-calibrated models that underestimate uncertainty and produce biased predictions. This stochasticity was injected in the current RTM by modelling the infection hazard, βt, as a stochastic process, a discrete-time random-walk with coarse time-steps (Birrell et al, 2021). This, however, was a pragmatic choice, designed, above all, to alleviate computational burden. Here Dr Birrell will present alternative representations for βt, and frame the choice between them on the basis of predictive ability, investigating the performance in predicting deaths among individuals testing positive for SARS-CoV-2 over a three-week time horizon. In doing so he will introduce a novel approach using approximating pathwise-expansions of diffusion processes. ![]() Dr Paul Birrell, UK Health Security Agency and MRC Biostatistics Unit University of Cambridge, UK
![]() Dr Paul Birrell, UK Health Security Agency and MRC Biostatistics Unit University of Cambridge, UKDr Paul Birrell is a Senior Statistician at the UK Health Security Agency, leading on real-time pandemic modelling and a Senior Research Associate at the MRC Biostatistics Unit at the University of Cambridge. He sits on the Scientific Pandemic Influenza Group on Modelling (SPI-M) a permanent sub-group of the Scientific Advisory Group for Emergencies (SAGE) which provides decision-making support to government in the event of a national emergency. Paul began working on transmission modelling in response to the 2009 A/H1N1pdm outbreak and, whilst working at the University of Cambridge, developed a real-time modelling framework that was adopted by Public Health England as their official real-time model. |
09:45-10:05 |
Challenges for mechanistic infectious disease forecast
Dynamic models of infectious disease systems are often used to study the epidemiological characteristics of disease outbreaks, the ecological mechanisms affecting transmission, and the suitability of various mitigation and intervention strategies. In recent years these same models have been employed to generate probabilistic forecasts of infectious disease incidence at population scale. Here, Professor Shaman will discuss some of the issues impeding mechanistic infectious disease forecast accuracy and possible pathways for overcoming these challenges. ![]() Professor Jeff Shaman, Carnegie Mellon University, USA
![]() Professor Jeff Shaman, Carnegie Mellon University, USAJeffrey Shaman is a Professor of Environmental Health Sciences in the Columbia University Mailman School of Public Health and of Climate in the Columbia University Climate School. He also serves as Director of the Climate and Health Program at the Mailman School, Faculty Chair of the Earth Institute, and Vice Dean for Faculty Affairs at the Climate School. Professor Shaman holds a BA in Biology from the University of Pennsylvania and an MA and PhD in Climate Science from Columbia University. Current research foci include study of the survival, transmission and epidemiology of infectious agents, development and improvement of infectious disease forecasting systems, and investigation of contagion and transmission processes generally. |
10:05-10:25 |
Strategic vs tactical modelling approaches to predicting vector-borne diseases
There are a variety of approaches to modelling and making predictions about the dynamics of infectious diseases. For instance, one can take a strategic/mechanistic approach that primarily concerns itself with determining what types of processes can cause certain patterns. Strategic models often require large amounts of data to parameterise them and make them useful for prediction. On the other extreme are tactical/phenomenological models, like regressions, that usually focus on fitting a pattern without elucidating why those patterns exist. Tactical models, while often conceptually simpler, can be poor for extrapolating beyond the range of the data. Thus, each approach has its strengths and weaknesses in terms of data needed to parameterise and validate the model and the types of predictions that we can make using them. Professor Johnson will talk about the open challenge of how to use data at different resolutions with models that incorporate both mechanistic and strategic elements to improve prediction. In particular, she will focus on building models to prediction the dynamics of vector-borne diseases at different spatial and temporal. ![]() Professor Leah Johnson, Virginia Tech, USA
![]() Professor Leah Johnson, Virginia Tech, USAProfessor Leah Johnson is an Associate Professor of Statistics at Virginia Tech. She received her BS in Physics from the College of William and Mary (Virginia, US), and then her MS (Physics) and PhD (Physics/Applied Mathematics and Statistics) at the University of California Santa Cruz. Before joining Virginia Tech, she held research posts at the University of Cambridge, University of California Santa Barbara, and University of Chicago, and was an Assistant Professor of Integrative Biology at the University of South Florida. Her research interests are in statistical and mathematical biology, ecology, and epidemiology. In particular, she is interested in how individual behaviour and local interactions between individuals in a population, influence population level patterns as well as statistical methods for inference in mechanistic models of biological systems. Recently, her work has focused on understanding how environmental factors (particularly temperature) influence transmission of vector-borne pathogens. |
10:25-10:30 |
Discussion
|
10:30-11:00 |
Break
|
11:00-11:20 |
The reproduction number: an essential ingredient for epidemic forecasts?
The epidemic reproduction number has gained global attention during the COVID-19 pandemic. A wide range of approaches exist to estimate it, with varying levels of data requirements, model complexity, and computational cost. Dr Cori will present one of the simplest approaches, EpiEstim, and review some of the recent developments to improve it, and implications for epidemic forecasting. She will showcase selected examples from her team’s recent work including on influenza and COVID-19. Finally, Dr Cori will discuss what she considers to be priorities for future epidemic forecasting based on reproduction number estimation. ![]() Dr Anne Cori, Imperial College London, UK
![]() Dr Anne Cori, Imperial College London, UKDr Cori develops statistical methods and tools for the analysis of epidemic data. She is particularly interested in emerging infections, and contexts where data are scarce and statistical methods can be developed to answer important public health questions despite these limitations in data. She is the author of the R package EpiEstim. Her research mainly focuses on viruses including SARS-CoV-2, Ebola, MERS, influenza and HIV. |
11:20-11:40 |
How can sequence data inform and improve epidemiological forecasting models
Throughout the COVID-19 pandemic, the SARS-CoV-2 virus has continued to evolve, with new variants outcompeting existing variants and often leading to different dynamics of disease spread. In this talk, Dr Del Valle will discuss how her team characterised variant transition differences with respect to speed, calendar timing, and magnitude of 13 SARS-CoV-2 variant waves/transitions for 215 countries and sub-country regions, between October 2020 and October 2022. Furthermore, she will discuss some of the associations contributing to the dynamics observed and how this work can inform epidemic forecasting models to ultimately increase accuracy. ![]() Dr Sara Del Valle, Los Alamos National Laboratory, USA
![]() Dr Sara Del Valle, Los Alamos National Laboratory, USADr Sara Del Valle is a Senior Scientist at Los Alamos National Laboratory where she works on the development of mathematical, statistical, and computational models for the spread of infectious diseases and other applications in support of decision makers. Her team combines large-scale heterogeneous data streams with epidemiological models to understand, model, and forecast disease spread in real-time. Her work has been covered in several top media outlets such as National Geographic, NPR Science Friday, Popular Mechanics, and the New York Times. She received a PhD in Applied Mathematics from the University of Iowa. |
11:40-12:00 |
Human judgement forecasting - limitations and opportunities
Predictions from human forecasters can play a useful role, complementing model-based predictions when aiming to provide timely and accurate forecasts to decision-makers and the public. Human judgement can incorporate information not easily available to computer models; it can be elicited rapidly, and it can help answer questions that are hard to capture in a model. On the other hand, human judgement forecasting requires experience, subject matter knowledge, as well as time and, therefore, is not easily scalable. This talk will discuss strengths and weaknesses of human judgment forecasting compared to model-based predictions and explore ways to overcome these by combining the two approaches. Dr Bosse will present findings from a study eliciting crowd forecasts of COVID-19 in Germany and Poland using a newly created app, 'crowdforecastr', and a follow-up study with a larger pool of forecasters conducted in the UK. ![]() Dr Nikos Bosse, London School of Hygiene and Tropical Medicine, UK
![]() Dr Nikos Bosse, London School of Hygiene and Tropical Medicine, UKNikos Bosse MD is a PhD student at the London School of Hygiene & Tropical Medicine working on infectious disease forecasting and forecast evaluation. For his PhD, he elicited forecasts from epidemiologists and lay people about the future trajectory of COVID-19 and compared these against model-based predictions. In addition to that, his work focuses on refining methods and building tools that help evaluate forecasts, such as the ‘scoringutils’ package in R. |
12:00-12:20 |
Forecasting the past, present and future of epidemics
An epidemic incidence curve is inherently latent, with substantial uncertainty even in the far past. To forecast the future, we must first reduce and manage the uncertainty in the immediate past and present. One framework for doing that is ‘pancasting’ – the joint stochastic modelling of past, present and future. The temporal dynamic model is the same throughout, while the distribution of past curves is stochastically constrained by measurements to date. Professor Rosenfeld will discuss this framework and its use of the data revision triangle. A realistic model of the temporal dynamics of epidemics must account for the fact that viral transmissibility is not constant, but rather a complex function of population immunity, environmental factors, government policy, human behaviour, and viral evolution. These drivers of viral transmissibility are well known yet their effect is hard to quantify. The first year of the pandemic gave us a rare opportunity to make progress on this front, due to (1) no pre-existing immunity; (2) slow viral antigenic evolution; and (3) unprecedentedly detailed measurements. Professor Rosenfeld will discuss his team’s ongoing attempt to use this period to glean some insight into the true drivers of SARS-Cov-2 transmissibility. Time permitting, he will also discuss Delphi’s work on acquiring epidemiologically relevant data sources and extracting and publishing myriad epidemic signals in real-time and at fine geographic granularity. ![]() Professor Roni Rosenfeld, Carnegie Mellon University, USA
![]() Professor Roni Rosenfeld, Carnegie Mellon University, USARoni Rosenfeld is head of the machine learning department and Professor of machine learning, language technologies, computer science, and computational biology in the School of Computer Science at Carnegie Mellon University, Pittsburgh, Pennsylvania. His current research interests are in tracking and forecasting epidemics. The Delphi Research group, which he co-founded and co-leads since 2012, has been playing a leading role in the development of epidemic forecasting technology in the US, and has been named a National Center for Epidemic Forecasting by the US CDC. Roni has previously worked on statistical language modelling, speech recognition, human machine speech interfaces, and the use of speech and language technologies to aid international developments. He received the Spira Teaching Excellence Award in 2017 and the Allen Newell Medal for Research Excellence in 1992 and again in 2022. |
12:20-12:30 |
Discussion
|
13:30-13:50 |
Forecasting COVID-19 healthcare demand: the case of France
Short-term forecasting of the COVID-19 pandemic is required to facilitate the planning of COVID-19 healthcare demand in hospitals. Over the course of the pandemic, Dr Paireau and her team developed different approaches to anticipate COVID-19 healthcare needs in France. In this talk, she will revisit the different steps that they followed to improve forecasts, moving from a single complex mechanistic model to an ensemble model relying on early predictors (such as the number of cases, mobility, or meteorological predictors) and a variety of statistical and machine learning methods. Dr Paireau will present the latest developments relative to this ensemble model, including the re-evaluation of the impact of mobility predictors in a period when non-pharmaceutical interventions restricting population movements were no longer the main driver of the epidemic dynamics. Now that we are moving towards endemicity, she will also present a new approach to forecast future COVID-19 waves based on the dynamics of past waves. Finally, Dr Paireau will discuss methodological challenges they faced in this forecasting exercise. ![]() Dr Juliette Paireau, Institut Pasteur, Santé publique France, France
![]() Dr Juliette Paireau, Institut Pasteur, Santé publique France, FranceDr Juliette Paireau is a research engineer in the Mathematical Modelling of Infectious Diseases Unit at Institut Pasteur, Paris and in the Infectious Diseases Department at Santé publique France, Paris. Her work is at the interface between modelling and public health. She conducts various research projects investigating the spatial and temporal dynamics of infectious diseases to improve prevention and control strategies. In particular, she develops ensemble models to forecast the dynamics of COVID-19 and influenza in France at the regional level, to support public health decision-making. |
---|---|
13:50-14:10 |
Trade-offs between individual and ensemble forecasts of an emerging infectious disease
Probabilistic forecasts play an indispensable role in answering questions about the spread of newly emerged pathogens. However, uncertainties about the epidemiology of emerging pathogens can make it difficult to choose among alternative model structures and assumptions. To assess the potential for uncertainties about emerging pathogens to affect forecasts of their spread, we evaluated the performance of 16 forecasting models in the context of the 2015-2016 Zika epidemic in Colombia. Each model featured a different combination of assumptions about human mobility, spatiotemporal variation in transmission potential, and the number of virus introductions. Dr Oidtman and her team found that which model assumptions had the most ensemble weight changed through time. They additionally identified a trade-off whereby some individual models outperformed ensemble models early in the epidemic, but on average the ensembles outperformed all individual models. Their results suggest that multiple models spanning uncertainty across alternative assumptions are necessary to obtain robust forecasts for emerging infectious diseases. At the end of the presentation, Dr Oidtman will additionally consider the public health implications of this research and the methodological challenges and potential advancements moving forward. This research was completed as part of a large cross-functional collaboration among UNICEF, public health entities in Colombia (Instituto Nacional de Salud and Ministerio de Salud y Protección Social), multiple universities, and a telecommunication company (Telefónica). ![]() Dr Rachel Oidtman, Colorado Access, USA
![]() Dr Rachel Oidtman, Colorado Access, USADr Rachel Oidtman is an infectious disease modeler and data scientist at Colorado Access, a non-profit healthcare provider in Colorado, USA. Her research focuses on the development of mathematical and statistical models to understand and forecast infectious disease dynamics, with a specific interest in reducing the burden of diseases. She has worked on a range of applied research questions and a variety of pathogens, including arboviruses (including Zika and dengue) and respiratory viruses (including influenza and SARS-CoV-2). Dr Oidtman earned her PhD in Biological Sciences from University of Notre Dame in 2020 and her BSc in Applied Natural Resources and Statistics from Cornell University in 2015. |
14:10-14:30 |
Lessons learned from real-time forecasting for the COVID-19 pandemic and seasonal influenza in the US
The COVID-19 and FluSight Forecast Hubs have collected, disseminated, synthesised, and evaluated millions of real-time forecasts for reported COVID-19 cases, hospitalisations, and deaths and influenza hospitalisations at the national, state, and county (cases only) level from more than 100 different academic, industry, and independent research groups. This presentation will review several key findings from Forecast Hub evaluations and experience from the operation of these systems, including:
![]() Dr Matthew Biggerstaff, US Centers for Disease Control and Prevention, USA
![]() Dr Matthew Biggerstaff, US Centers for Disease Control and Prevention, USAMatthew Biggerstaff, ScD, MPH, serves as Team Lead of the Applied Research and Modeling Team in CDC’s Influenza Division’s Epidemiology and Prevention Branch (EPB). In this role, he oversees team activities related to the following: characterizing flu season severity, disease burden, and influenza virus transmission in the population; integrating advanced analytics and mathematical modelling into flu-related public health response efforts; and improving the use and accuracy of influenza forecasting to better predict and mitigate the impact of flu. Dr Biggerstaff joined CDC in 2006 and has been with the Influenza Division since 2009. |
14:30-14:40 |
Discussion
|
14:40-15:15 |
Flash talks: software and tools
|
15:15-15:45 |
Break
|
15:45-16:05 |
Evaluation of infectious disease forecasts
Forecast evaluation is a crucial step in the development cycle of epidemiological prediction models, and a prerequisite for data-driven combination of different forecasts into an ensemble. Moreover, transparent evaluation is required to create trust in predictions and determine to which degree they can inform public health decision making. In this talk Dr Bracher will provide an overview of statistical evaluation methods, with a particular focus on probabilistic forecasts. He will introduce some central theoretical concepts including PIT histograms as well as consistency and propriety of scoring rules. The qualitative behaviour and practical applicability of different scoring rules will be contrasted, and score decompositions which enhance the interpretability of results will be discussed. He will then turn to specific challenges encountered in large-scale epidemiological forecasting projects. These include accounting for the discreteness of forecast targets, the comparison of models applied to incongruent sets of forecasting tasks, the role of naïve benchmark models and the appropriate choice of scale on which forecasts are evaluated. To conclude, Dr Bracher will touch upon the relationship between statistical evaluations and the perceived public health utility of different predictions. ![]() Dr Johannes Bracher, Karlsruhe Institute of Technology, Germany
![]() Dr Johannes Bracher, Karlsruhe Institute of Technology, GermanyJohannes Bracher is a postdoctoral researcher at Karlsruhe Institute of Technology (KIT) and Heidelberg Institute of Theoretical Studies (HITS), Germany. He holds an MSc in Statistics from the University of Munich (2016) and a PhD in Biostatistics from the University of Zurich (2020). His research interests include probabilistic forecasting, in particular forecast evaluation and combination, as well as real-time modelling of infectious diseases and count time series methodology. In 2023 he will establish an Emmy Noether Independent Junior Research Group at KIT, funded by the German Research Foundation (DFG). |
16:05-16:25 |
Evaluating forecasts in the context of public health decision making
Infectious disease forecasts may be useful for public health workers. For example, they can serve as a general tool for situational awareness to help inform communications with the public. They could also support more specific decision-making tasks such as site selection for clinical trials, implementation of non-pharmaceutical interventions, or planning the allocation of a limited supply of medical care resources such as ventilators. In settings where forecasts are used as an input to specific decisions, it may be helpful to evaluate the value of forecasts in the context of that decision-making exercise. For example, some decisions may require accurate information about the timing of the onset of a new wave of infections, while other decisions may require accurate forecasts of the timing of a peak in hospitalisations, and still other decisions may require accurate information about the relative size of peaks in hospitalisations across different jurisdictions. Different forecasters may achieve varying levels of accuracy for these different tasks. Professor Ray will illustrate these ideas using the example of allocating a limited supply of medical care resources to multiple jurisdictions. He has developed a proper scoring rule that formalises a measure of the value of a forecast in the context of this decision-making problem, as determined by the costs that would be incurred through misallocation if the forecast were used to set allocation levels. In his presentation, Professor Ray will explore how this scoring rule is related to the Weighted Interval Score (WIS) and will illustrate how it could be used to evaluate forecasts from the US COVID-19 Forecast Hub. ![]() Professor Evan Ray, University of Massachusetts, USA
![]() Professor Evan Ray, University of Massachusetts, USAProfessor Ray is a Research Assistant Professor of Biostatistics at the University of Massachusetts, Amherst, and co-director of the US COVID-19 Forecast Hub. His research focuses on methods for infectious disease forecasting. |
16:25-16:45 |
What does it mean to evaluate a scenario projection? Lessons from the COVID-19 Scenario Modelling Hub
Over 24 months, the COVID-19 Scenario Modelling Hub (SMH) has provided 16 rounds of scenario projections to inform the US pandemic response. In each round, the SMH solicits and ensembles 3- to 12-month projections of cases, hospitalisations, and deaths at the state and national level from 6-10 independent modelling teams. Here we evaluate the SMH effort over these 16 rounds. However, the nature of scenario projections makes evaluation challenging. Scenario projections are conditional on a set of assumptions about the future, which will never unfold exactly as assumed. To address these challenges, we present a conceptual framework for understanding and interpreting the evaluation of a scenario projection. This framework rests on an evaluation of both the scenarios themselves (eg, scenario-specified vaccine uptake vs realised uptake) and the projections that were based on these scenarios (eg, projected incident hospitalisations vs observed hospitalisations). Key findings for SMH include: (1) in most cases, scenarios bracketed reality, but very few were close to the realised future; and (2) for scenarios that were “close” to reality, the ensemble was consistently more reliable than individual models. Building on the SMH experience, Dr Howerton will discuss further foundational work on scenario evaluation to advance the growing field of multi-model infectious disease scenario projections. ![]() Dr Emily Howerton, Penn State University, USA
![]() Dr Emily Howerton, Penn State University, USAEmily Howerton is a PhD candidate in the Department of Biology at Penn State University. She uses mathematical models to design strategies for controlling infectious diseases in the face of uncertainty, most recently focusing on the COVID-19 pandemic. For example, Emily has modelled the interaction between diagnostic testing and non-pharmaceutical interventions to identify optimal combination management strategies. Emily is also a member of COVID-19 Scenario Modelling Hub coordination team, where she develops methods to capture uncertainty from multiple models in 6-month projections of COVID-19 outcomes. In particular, she has adapted aggregation and evaluation methods from multiple fields to accommodate the unique needs of infectious disease scenario projections. |
16:45-17:05 |
Sequential evaluation of decisions throughout emergency response to outbreaks
The primary task of a decision-maker during a disease outbreak is to decide on the best course of action. When making decisions in the face of uncertainty, such as in responding to emergency disease outbreaks, there exists a trade-off between information accrual and capitalising upon information by taking action. That is, as information accrues, such as via improved parameter estimates or model structure clarification, we become better informed as to the best course of action. However, the longer we wait to take action, the larger the potential opportunity cost from inaction. This work investigated this trade-off by updating model estimates and subsequent control action recommendations throughout the foot-and-mouth disease outbreaks of 2001 in the UK and 2010 in Miyazaki, Japan. Every four weeks throughout each outbreak, parameters were re-estimated based upon all available data and control actions re-evaluated. These recommendations were compared to those derived from parameters estimates using data from the whole outbreak (ie assuming one has all available information from the start of the outbreak). Overall, Dr Probert and his team found that while forecasts of outbreak severity in the early stages of an outbreak may be poor, the relative performance of different interventions were resolved early. He will reflect on comparable studies in other infectious disease systems. ![]() Dr Will Probert, Consultant, UK
![]() Dr Will Probert, Consultant, UKWill Probert currently works as a consultant within WHO's Global HIV, Hepatitis, and STI programmes. Will previously held postdoctoral research positions deploying individual-based models to inform public health, or veterinary, decision-making. While at Oxford University with Professor Christophe Fraser, projects included being co-lead developer of the COVID-19 individual-based model 'OpenABM-Covid19' and as a modeller on the HPTN 071 (PopART) trial of HIV prevention. At both the University of Warwick, with Professor Mike Tildesley, and the Pennsylvania State University, with Professor Katriona Shea and Professor Matt Ferrari, projects investigated the use of reinforcement learning algorithms to generate novel control strategies in individual-based models of foot-and-mouth disease. |
17:05-17:15 |
Discussion
|
Chair
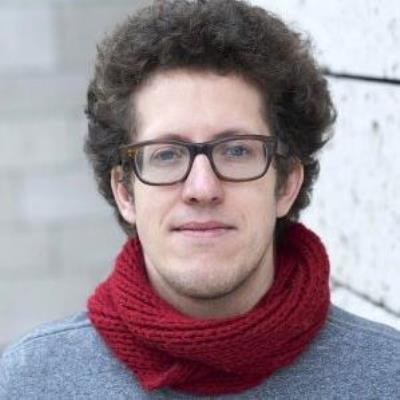
Professor Sebastian Funk, London School of Hygiene and Tropical Medicine, UK
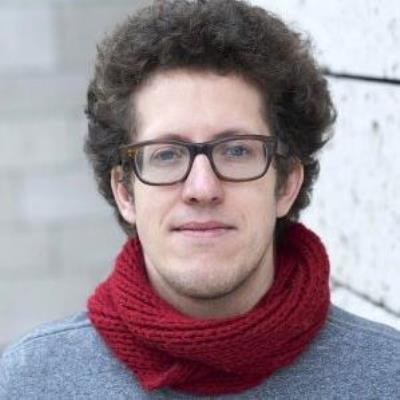
Professor Sebastian Funk, London School of Hygiene and Tropical Medicine, UK
Sebastian Funk is a Professor of Infectious Disease Dynamics at the London School of Hygiene & Tropical Medicine and Wellcome Trust Senior Research Fellow. His work focuses on statistical and mathematical models of infectious disease dynamics, and particularly the application to ongoing outbreaks. He has worked with numerous national and international agencies such as the World Health Organization (WHO), the European Centre for Disease Control (ECDC) and Public Health England (PHE). He leads the EpiForecast group at which produces real-time modelling of infectious disease outbreaks in collaboration with public health decision makers.
09:00-09:20 |
Evidence for action
Public health decisions can take many forms, from individual decisions about mitigating personal risk to global vaccine development policy. Forecasting is intrinsic to these decisions and is increasingly being addressed with quantitative models. Yet quantitative forecasting is relatively new to public health and there is often limited, or sometimes blind, trust in models. Specific forecasts may be heralded for convenience, institutional reverence, inclusion of certain variables, or one-off successes. However, as forecasting becomes more common, we will all benefit from better evidence of the effective development, communication, and use of forecasts. One example is ensemble forecasts. Multiple forecasting challenges have shown that ensembles provide more robust forecasts than individual forecast models. Those assessments also indicate that we need forecasts for longer horizons that are more precise, more reliable, and cover more geographical scales. In addition to better forecasts, we also need to improve the evidence base by developing interpretable skill metrics and performance benchmarks, including assessments for forecasted outcomes which may change as a result of the forecast. Over the past decade infectious disease forecasting has gone from obscurity to the nightly news. Evidence of the strengths and weaknesses of forecasts can both drive their improvement and cement their place in the public health toolbox. ![]() Dr Michael Johansson, US Centers for Disease Control and Prevention, USA
![]() Dr Michael Johansson, US Centers for Disease Control and Prevention, USAMichael Johansson is a Biologist and Epidemic Analytics Team Lead in the Centers for Disease Control and Prevention Division of Vector-Borne Diseases and an Adjunct Lecturer at the Harvard TH Chan School of Public Health Center for Communicable Disease Dynamics. He uses statistical and mathematical modelling to improve surveillance, prevention, and control of arboviral diseases. He also works to improve the use of quantitative models to support decision making related to infectious disease outbreaks more broadly, including co-leading the CDC COVID-19 Modelling Team, the interagency COVID-19 Data and Analytics Task Force, White House working groups, and the CDC Epidemic Prediction Initiative, which he also co-founded. |
---|---|
09:20-09:40 |
Annual dengue outlook of Singapore, derived from machine learning and risk signals
Dengue is endemic in tropical Singapore and outbreaks are not uncommon due to presence of Aedes aegypti which is a very efficient vector, low population immunity as a result of decades of effective dengue control, a diversity of dengue viruses from continuous introduction and in-country transmission, and a very conducive warm and humid urban environment. Dengue epidemiology is driven by complex interactions of a myriad of factors, which include a) four serotypes of dengue viruses, with varying and unknown degree of epidemic potential and disease severity; b) vector capacity (vector density and competency) of mosquitoes; c) immunity of human population, made complex by varying and unknown level and duration of cross protection from different serotypes; d) human behaviour and interventions; and e) environmental conditions such as state of infrastructure, temperature, rainfall and humidity. The difficult-to-predict epidemiology of dengue had made preparation for outbreaks and early intervention challenging. We have thus developed a predictive LASSO and a random-forest model based on our understanding of the drivers of dengue. They are used in conjunction with other data such as serotype, seroprevalence and vector population to assess national and localised dengue risk, spatially and temporally. An annual dengue outlook is projected early in the year to inform preparation and activities such as allocation of resources, stepping up inspection of premises, convening the Inter-Agency Dengue Task Force and social mobilisation. ![]() Dr Ng Lee-Ching, National Environment Agency, Singapore
![]() Dr Ng Lee-Ching, National Environment Agency, SingaporeAssociate Professor Ng Lee-Ching is the Group Director of Environmental Health Institute (EHI) at the National Environment Agency in Singapore. She has spent more than 20 years building scientific capabilities for Singapore’s environmental public health, conducting research to understand disease risk and transmission, and developing tools and strategies for mitigation of risks. She has co-authored more than 210 scientific papers and book chapters, in the area of vector-borne diseases, COVID-19, food borne diseases, anti-microbial resistance, indoor air quality etc. She serves as an Adjunct Associate Professor at the Nanyang Technological University of Singapore and as the Director of the WHO Collaborating Centre for Reference and Research of Arbovirus and their Associated Vectors. |
09:40-10:00 |
Analytics for decision making during Public Health Emergencies
Through examples of recent epidemics this talk will focus on the use of analytics and forecasting for decision making during acute responses and discuss some ongoing challenges and limitations. The presentation will specifically focus on a Global Situational Alert System developed by WHO during the COVID-19 pandemic to help resource allocation and critical assistance, using a mixed method combining short-term forecasting with contextual, social, and epidemiological analysis. It will outline both the technical features and implementation aspects and discuss the practical use of the approach and its potential application to other emergencies. ![]() Dr Olivier le Polain OBE, World Health Organisation, Switzerland
![]() Dr Olivier le Polain OBE, World Health Organisation, SwitzerlandOlivier le Polain currently heads the Acute Events Analytics unit within the Acute Response Coordination Department of WHO’s Health Emergencies Programme in Geneva, supporting responses to epidemics and acute humanitarian crises. Between 2017 and 2020, prior to joining WHO, he was Deputy Director of Operations on the UK-Public Health Rapid Support Team at Public Health England (now UK Health Security Agency). Prior to that, he held various other roles as epidemiologist and public health specialist in the UK and in several countries in Sub-Saharan Africa and South Asia, particularly in response to epidemics and public health crises. Olivier trained in medicine in Belgium, holds an MSc in Public Health from the London School of Hygiene and Tropical Medicine (LSHTM), is an alumnus of the European Programme for Intervention Epidemiology (EPIET), holds a PhD in infectious disease epidemiology from LSHTM, and is a Fellow of the Faculty of Public Health in the UK. |
10:00-10:10 |
Discussion
|
10:10-10:30 |
Discussion: decision making
|
10:30-11:00 |
Break
|
11:00-11:20 |
Forecasting to inform the Australian COVID-19 response
Since April 2020, Australia's national and state public health responses to COVID-19 have been informed by weekly situational reports that include an ensemble COVID-19 case forecast for each jurisdiction. Forecasts of ward and ICU bed occupancy in each jurisdiction, based on these case forecasts, have also been provided. Forecasting models have been contributed by several groups around Australia, and these models have been repeatedly revised over the past three years to address priority public health decisions. Model revisions have included multiple characterisations of vaccine-acquired immunity, accounting for the emergence of new variants of concern and co-circulation of multiple variants, the impact of new variants on hospital patient length of stay and incorporating time-varying case ascertainment driven by changes in healthcare-seeking behaviours and clinical decision-making. In this talk Dr Moss will give an overview of how these forecasts have been developed and evaluated, highlight some of the data sources and analyses that have fed into the forecasting models, explain how we have selected which models to include in the ensemble at different periods of the pandemic, and reflect on his group's experiences in visualising and communicating these forecasts to government committees and other public health stakeholders. ![]() Dr Rob Moss, University of Melbourne, Australia
![]() Dr Rob Moss, University of Melbourne, AustraliaRob Moss is a Senior Research Fellow in the Infectious Disease Dynamics Unit at the Melbourne School of Population and Global Health, University of Melbourne. His research focuses on predicting and mitigating the burden of infectious disease epidemics, through the use of mathematical/computational modelling and Bayesian inference. In collaboration with state and national Departments of Health, his work has informed national pandemic preparedness and response plans, and public health responses to pandemic influenza and COVID-19. He develops research software and publishes open-source software packages. He also leads professional development activities to support RHD students and EMCRs to make effective use of computational resources and ensure that their research is reproducible. |
11:20-11:40 |
Navigating the maze of model outputs - communicating the complex for better understanding
The communication of epidemic modelling scenarios and forecasts presents numerous challenges that can impact their effectiveness in informing public health decision-making. These challenges include the limitations of forecasting models, the complexity of the data and information being communicated, and the need to convey uncertainty and risk. The definition of best practices in communication strategies and visualisation techniques that bridge the gap between the modelling practitioners and decision makers (and the public) are crucial in ensuring that modelling results are actionable. Professor Vespignani will briefly discuss examples of strategies, and their pitfalls, in communicating the landscape of complex modelling results. ![]() Professor Alessandro Vespignani, Northeastern University, USA
![]() Professor Alessandro Vespignani, Northeastern University, USAAlessandro Vespignani is Sternberg Family distinguished Professor of Computer Science and Health Sciences and the Director of the Network Science Institute at Northeastern University, Boston. With a focus on interdisciplinary research, he applies statistical and numerical simulation methods to study the spread of epidemics and the analysis of biological, social, and technological networks. Vespignani is known for his work on modelling the spatial spread of diseases, particularly in the area of emerging infectious diseases. Vespignani’s work on modelling the spatial spread of epidemics includes the realistic and data-driven modelling of emerging infectious diseases, and contributions to computational epidemiology by developing specific tools for the analysis and forecast of the global spread of epidemics. Vespignani is a Fellow of the American Physical Society, the NetworkScience Society, member of the Academy of Europe, and Fellow of the Institute for Quantitative Social Sciences at Harvard University. |
11:40-12:00 |
Infectious disease modelling to support rapid outbreak response: insights from academia and government
Over the past decade, the use of infectious disease modelling in public health practice has grown significantly. Modelling has been instrumental in guiding decisions during the COVID-19 response, including during waves driven by new variants, and has also played a role in responding to the monkeypox outbreak. In previous public health emergencies, this support was primarily provided through academic-government hybrid arrangements. More recently, new modelling groups have been established by the US Centres for Disease Control and Prevention and the UK Health Security Agency, and long-standing academic consortia and hybrid arrangements continue to provide immense value. However, more work is needed to ensure that the field of applied epidemiological modelling continues to grow and evolve, including establishing a pipeline for training. This talk will provide an overview of the latest developments in the structure and function of applied epidemiological modelling in public health practice, including identifying important gaps and lessons learned. ![]() Dr Caitlin Rivers, Johns Hopkins University, USA
![]() Dr Caitlin Rivers, Johns Hopkins University, USADr Rivers is a Senior Scholar at the Johns Hopkins Center for Health Security and an Assistant Professor in the Department of Environmental Health and Engineering at the Johns Hopkins Bloomberg School of Public Health. She is an epidemiologist specialising in preparedness and response for epidemics, pandemics and deliberately occurring events. Dr Rivers recently returned from an appointment as founding associate director of the Center for Forecasting and Outbreak Analytics at the Centers for Disease Control and Prevention (CDC). Dr Rivers has testified in front of the United States Congress on several occasions and is a frequent advisor to senior leaders at the state and federal levels. She served on the Biden-Harris Presidential Transition Team working on COVID-19 policies. Her writing has been published in the Washington Post, Wall Street Journal, New York Times, and USA Today. |
12:00-12:20 |
Are planning scenarios forecasts? Predictive epidemic modelling to inform policy over the longer term
The SARS-CoV-2 experience has shown that forecasting the trajectory of epidemics of acute infectious diseases more than a few weeks into the future is a difficult, if not impossible, task. However, we often need to make plans months or years into the future. Defining planning scenarios that make explicit assumptions about future epidemic drivers is one way to inform such decisions and can also provide insight into the likely impact of decisions we make about disease control or possible future events (eg, emergence of a new variant). Communication, interpretation, and evaluation of model projections based on these scenarios is complicated by the fact that the assumptions themselves are unlikely to exactly match reality, hence predictions conditional on these assumptions are unlikely to match observations even if the models themselves are perfectly calibrated. Still, if planning scenarios are to be taken seriously, they must have some level of "face validity" in light of recent observations in the period immediately after their release, and match observed data to some extent when scenario assumptions roughly hold. In this talk Justin Lessler will explore the sometimes-fuzzy line between planning scenarios and forecast through the lens of the work of the US COVID-19 Scenario Modelling Hub and early scenario modelling efforts. He will discuss the communication and evaluation of such predictions, with a particular focus on how our tendency to treat any model projection as a forecast. ![]() Justin Lessler, University of North Carolina, Gillings School of Global Public Health, USA
![]() Justin Lessler, University of North Carolina, Gillings School of Global Public Health, USAJustin Lessler is a Professor in the Department of Epidemiology researching the dynamics and control of infectious disease, with particular interest in SARS-CoV-2, influenza, cholera and dengue. Justin works on the development and application of statistics, dynamic models, and novel study designs to better understand and control infectious disease. In particular, he is interested in creating synergies between infection control practice, data collection and infectious disease dynamics. |
12:20-12:30 |
Discussion
|
Chair
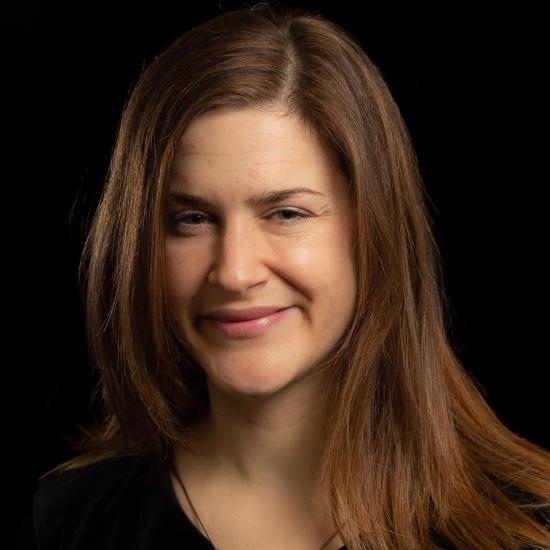
Professor Rachel Lowe, ICREA/Barcelona Supercomputing Center, Spain
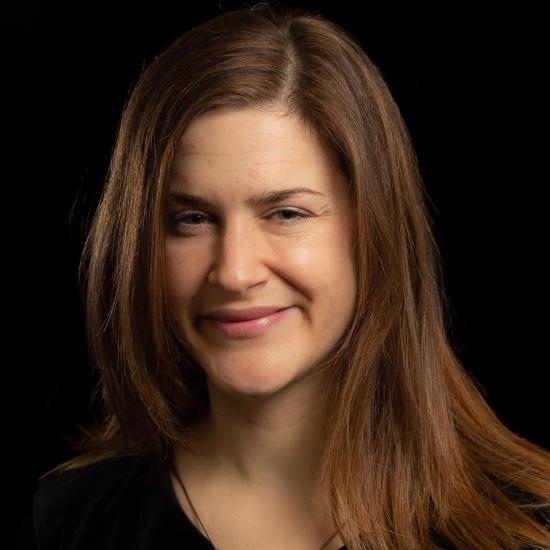
Professor Rachel Lowe, ICREA/Barcelona Supercomputing Center, Spain
Rachel Lowe is an ICREA Research Professor and Global Health Resilience Team Leader at the Barcelona Supercomputing Center. She also holds a Royal Society Dorothy Hodgkin Fellowship at the London School of Hygiene & Tropical Medicine. Rachel’s research focuses on co-developing policy-relevant methodological solutions, to enhance surveillance, preparedness and response to climate-sensitive disease outbreaks and emergence. She has published high impact research on integrating seasonal climate forecasts in early warning systems for infectious diseases in Latin America, the Caribbean and Southeast Asia. Rachel is the Director of the Lancet Countdown on health and climate change in Europe. She is a member of the World Meteorological Organization COVID-19 research task team and was a contributing author of the IPCC Sixth Assessment Report (WGII) chapter on risks across sectors and regions.
13:30-14:10 |
Breakout session: synthesis of the two meetings
|
---|---|
14:10-14:45 |
Discussion
|
14:45-15:00 |
Final points
|