Bits, neurons, and qubits for sustainable AI
Discussion meeting organised by Professor Osvaldo Simeone, Professor Bipin Rajendran, Professor Yulia Sandamirskaya, and Dr Olga Kazakova.
The meeting will focus on the design and analysis of computing technologies for sustainable AI that leverage informational and physical properties of computing platforms beyond standard deterministic digital processing.
Organisers
Schedule
Chair
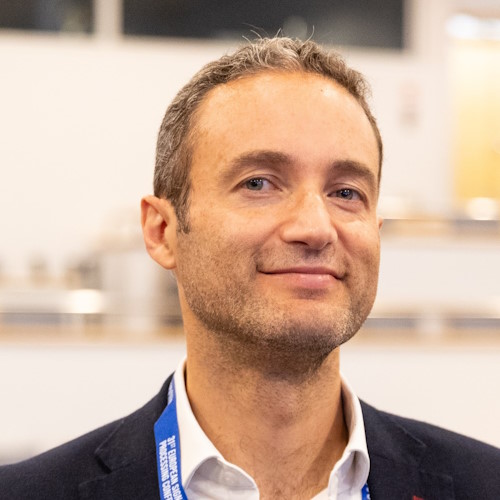
Professor Osvaldo Simeone
King's College London, UK
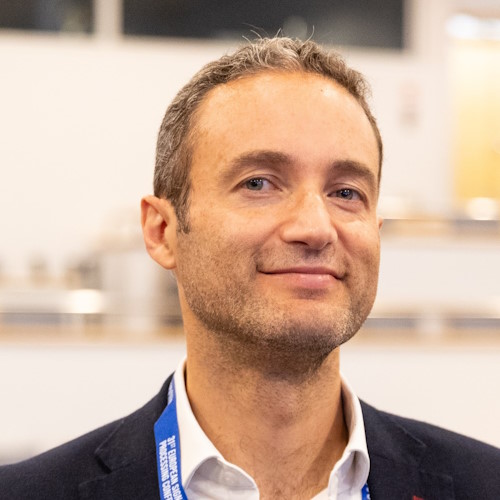
Professor Osvaldo Simeone
King's College London, UK
Osvaldo Simeone is a Professor of Information Engineering. He co-directs the Centre for Intelligent Information Processing Systems within the Department of Engineering of King's College London, where he also runs the King's Communications, Learning and Information Processing lab. He is also a visiting Professor with the Connectivity Section within the Department of Electronic Systems at Aalborg University. He received an MSc degree (with honours) and a PhD degree in information engineering from Politecnico di Milano, Milan, Italy, in 2001 and 2005, respectively. From 2006 to 2017, he was a faculty member of the Electrical and Computer Engineering (ECE) Department at New Jersey Institute of Technology (NJIT), where he was affiliated with the Center for Wireless Information Processing (CWiP). His research interests include information theory, machine learning, wireless communications, neuromorphic computing, and quantum machine learning. Dr Simeone is a co-recipient of the 2022 IEEE Communications Society Outstanding Paper Award, the 2021 IEEE Vehicular Technology Society Jack Neubauer Memorial Award, the 2019 IEEE Communication Society Best Tutorial Paper Award, the 2018 IEEE Signal Processing Best Paper Award, the 2017 JCN Best Paper Award, the 2015 IEEE Communication Society Best Tutorial Paper Award and of the Best Paper Awards of IEEE SPAWC 2007 and IEEE WRECOM 2007. He was awarded an Open Fellowship by the EPSRC in 2022 and a Consolidator grant by the European Research Council (ERC) in 2016. His research has been also supported by the US National Science Foundation (NSF), the European Commission, the European Research Council (ERC), the Engineering & Physical Sciences Research Council (EPSRC), the Vienna Science and Technology Fund, the European Space Agency, as well as by a number of industrial collaborations including with Intel Labs and InterDigital. He was the Chair of the Signal Processing for Communications and Networking Technical Committee of the IEEE Signal Processing Society in 2022, as well as of the UK & Ireland Chapter of the IEEE Information Theory Society from 2017 to 2022. He was a Distinguished Lecturer of the IEEE Communications Society in 2021 and 2022, and he was a Distinguished Lecturer of the IEEE Information Theory Society in 2017 and 2018. Professor Simeone is the author of the textbook "Machine Learning for Engineers" published by Cambridge University Press, four monographs, two edited books, and more than 200 research journal and magazine papers. He is a Fellow of the IET, EPSRC, and IEEE.
09:00-09:10 |
Welcome by the Royal Society and lead organiser
|
---|---|
09:15-10:00 |
Resource-constrained learning over wireless networks
It is anticipated that the next generation of wireless networks will incorporate AI to a significant degree at all network layers. A major part of this trend is the movement of AI and machine learning functions to the network edge. This is due to several reasons: (i) a growing number of AI applications demand implementations involving end-user devices, (ii) much data of interest is born at the network edge on smart phones and IoT devices, and (iii) fog/edge computing has emerged to take advantage of the increasing sophistication of end-user devices. A notable framework for engaging the wireless network edge in machine learning is wireless federated learning, in which multiple end-user devices collaborate with the help of an aggregator to build a common model, each using its local data. In this framework, exchanges between end-user devices and the aggregator necessarily take place over wireless links. Since wireless networks are notoriously resource-limited, this creates a situation in which the interactions between the wireless medium and machine learning algorithms must be considered as a factor in the design and implementation of AI applications. This talk will explore aspects of this problem, including trade-offs among energy consumption and other criteria such as spectral efficiency, learning rate, model complexity and data privacy. ![]() Professor H Vincent Poor FREng ForMemRSPrinceton University, USA ![]() Professor H Vincent Poor FREng ForMemRSPrinceton University, USA H Vincent Poor is the Michael Henry Strater University Professor at Princeton University, where his interests include information theory, machine learning and network science, and their applications in wireless networks, energy systems and related fields. Since 2004, he has also been a Visiting Professor at Imperial College, and he has held visiting positions at a number of other universities as well, including most recently at Berkeley and Caltech. Among his publications is the book Machine Learning and Wireless Communications (Cambridge University Press, 2022). Dr Poor is a member of the US National Academy of Engineering and the US National Academy of Sciences, an International Fellow of the Royal Academy of Engineering, and a Foreign Member of the Royal Society, among memberships in other national and international academies. Recognition of his work includes the IEEE Alexander Graham Bell Medal and honorary doctorates from a number of universities in Asia, Europe, and North America. |
10:00-10:15 |
Break
|
10:15-11:00 |
Information vs computation in non-linear transform based compression
Professor Weissman will focus the talk on a few non-linear transforms and their use for compression. The first is a "textual transform" sharing key properties with traditional transforms underlying much of our current multimedia compression technologies. It can form the basis for compression at bit rates until recently considered uselessly low, and for boosting human satisfaction from reconstructions at more traditional bit rates. The second is a "neural transform" - such as in the "implicit neural representation" framework - allowing to view the tension between compression ratio and neural network performance through the lens of rate-distortion theory and to develop information theoretically guided network pruning strategies. The third is a "Lempel-Ziv (LZ) transform", which can take any of a large class of compressors into new ones that share the main universality properties of the Lempel-Ziv compressor. Collectively, these transforms give insight into achievable trade-offs between compression and computation. These insights carry over to other information processing tasks such as classification, denoising and "GenAI". ![]() Professor Tsachy WeissmanStanford University, USA ![]() Professor Tsachy WeissmanStanford University, USA Tsachy Weissman is the Robert and Barbara Kleist Professor in the School of Engineering, and Professor of Electrical Engineering at Stanford University, where he has been on the faculty since 2003. His researching and teaching focus on the science of information, with applications spanning genomics, neuroscience, and technology. He has been serving on editorial boards for scientific journals, technical advisory boards in industry, and as the Founding Director of the Stanford Compression Forum. His recent projects include the SHTEM summer internship program for high schoolers, the Starling initiative for data integrity, and Stagecast, a low-latency video platform allowing actors and singers to perform together in real-time while geographically distributed. He has received multiple awards for his research and teaching, including best paper awards from the IEEE Information Theory and Communications Societies, while his students received best student authored paper awards at the top conferences of their areas of scholarship. He has prototyped Guardant Health’s algorithms for early detection of cancer from blood tests, co-founded and sold Compressable to Amazon, and worked at AWS on reducing humanity’s cloud storage footprint via compression. His favourite gig to date was advising the HBO show Silicon Valley. He hates writing about himself in the third person. |
11:15-12:00 |
On theories for interference-compute scaling and information batteries
There is growing interest in improving the performance of large language models for reasoning tasks by drawing on techniques such as chain-of-thought reasoning. Moreover, recent empirical findings demonstrate a scaling law relationship between inference-time compute and performance. Unfortunately, such techniques increase the computational and energetic resources used during the inference phase of AI systems, scaling with the amount of inference-time compute. Unlike large model training, such inference energy is not a one-time cost, but is incurred each time the model is used. Further, such computational effort must be done in essentially real time, rather than over periods of weeks or months that allow some time shifting. Recent research has proposed a concept of information batteries where the intermittency of renewable power is smoothed by storing energy in the form of information, specifically by storing results of predicted computational tasks, which may be relevant for energy-intensive AI applications. Here we ask three questions: (1) whether there are mathematical theories akin to information-theoretic analyses that provide insight into inference-compute scaling laws; (2) whether information batteries might fruitfully be used for AI inference workloads; and (3) whether there are information-theoretic limits to information batteries. ![]() Professor Lav R VarshneyUniversity of Illinois Urbana-Champaign, USA ![]() Professor Lav R VarshneyUniversity of Illinois Urbana-Champaign, USA Lav Varshney is an associate professor of electrical and computer engineering at the University of Illinois Urbana-Champaign, co-founder and CEO of Kocree, Inc, a start-up company using novel human-integrated AI in social music co-creativity platforms to enhance human wellbeing across society, and chief scientist of Ensaras, Inc, a start-up company focused on AI and wastewater treatment. He also holds appointments at RAND Corporation and at Brookhaven National Laboratory. He is a former White House staffer, having served on the National Security Council staff as a White House Fellow, where he contributed to national AI and wireless communications policy. His research interests include information theory and artificial intelligence. He received his BS degree from Cornell University and his SM and PhD degrees from the Massachusetts Institute of Technology. |
Chair
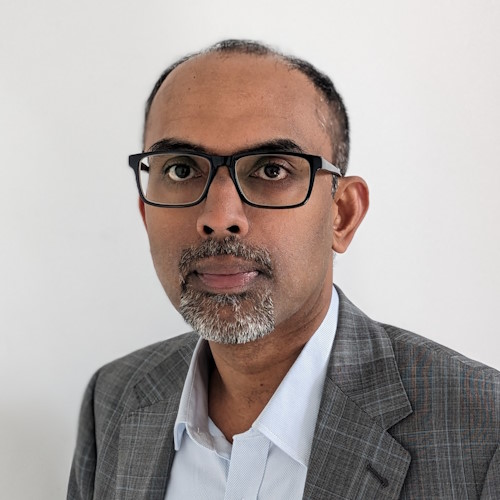
Professor Bipin Rajendran
King's College London, UK
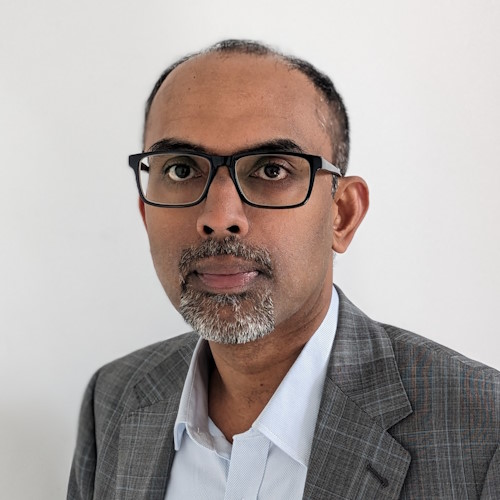
Professor Bipin Rajendran
King's College London, UK
Bipin Rajendran is a Professor of Intelligent Computing Systems and EPSRC Fellow at King’s College London (KCL). He received a B Tech degree from IIT Kharagpur in 2000, and MS and PhD degrees in Electrical Engineering from Stanford University in 2003 and 2006, respectively. He was a Master Inventor and Research Staff Member at IBM TJ Watson Research Centre in New York from 2006-’12 and has held faculty positions in India and the US. His research focuses on building algorithms, devices, and systems for brain-inspired computing. He has co-authored over 100 papers in peer-reviewed journals and conferences, one monograph, one edited book, and 59 issued US patents. He is a recipient of the IBM Faculty Award (2019), IBM Research Division Award (2012), and IBM Technical Accomplishment (2010). He was elected a senior member of the US National Academy of Inventors in 2019.
13:30-14:15 |
In-memory computing: revolutionising AI acceleration at Axelera AI
In-Memory Computing (IMC) is an innovative paradigm that integrates computation and memory, enabling ultra-dense, energy-efficient, and high-throughput hardware acceleration for deep neural networks. This talk will begin with an overview of the latest advancements in IMC, comparing various analogue and digital approaches and assessing their suitability for deep learning workloads. In the second part, Dr Eleftheriou will explore Axelera AI's cutting-edge implementation of Digital In-Memory Computing (D-IMC) combined with RISC-V technology. This unique integration delivers a scalable, high-performance platform for AI inference tasks, ranging from edge-based computer vision to emerging generative AI workloads. By leveraging the strengths of D-IMC, Axelera AI is redefining the landscape of AI acceleration, achieving exceptional energy efficiency, performance, and cost-effectiveness while driving the next generation of AI deployment. ![]() Dr Evangelos EleftheriouAxelera AI, The Netherlands ![]() Dr Evangelos EleftheriouAxelera AI, The Netherlands Evangelos Eleftheriou is the CTO and co-founder of Axelera AI. Previously, he held various research and management positions at IBM Research - Zurich. He has a PhD and a Master of Eng in Electrical Engineering from Carleton University, Canada, and a BSc in Electrical & Computer Engineering from the University of Patras, Greece. His interests include AI, machine learning, and emerging computing paradigms such as neuromorphic and in-memory computing. He has authored over 250 publications and holds over 160 patents. Evangelos is an IEEE Fellow and co-recipient of the 2003 IEEE ComS Leonard G Abraham Prize Paper Award, the 2005 Technology Award of the Eduard Rhein Foundation, and the 2009 IEEE Control Systems Technology Award and IEEE Transactions on Control Systems Technology Outstanding Paper Award. He was also appointed an IBM Fellow in 2005 and inducted into the IBM Academy of Technology. In 2016, he received an honoris causa professorship from the University of Patras, Greece, and in 2018, he was inducted into the US National Academy of Engineering as Foreign Member. |
---|---|
14:15-14:30 |
Break
|
14:30-15:15 |
Self-timed systems for energy-efficient computing
Self-timed circuits can significantly improve the energy-efficiency of large-scale digital computer systems. The benefits of these circuits include switching activity only when there is useful computation being performed, and the ability to optimise for expected delays rather than the system-wide worst-case delay. This benefit is partially offset by the overheads introduced to implement synchronisation and communication protocols. We present both analytical results as well as chip examples that demonstrate the efficiency of self-timed systems. The benefits of self-timed logic are immediately evident when designing large-scale neuromorphic systems. Many past and recent large-scale neuromorphic systems use self-timed digital electronics to achieve energy-efficient operation. We will explain why this is the case, and highlight the uses of self-timed logic in the design of the TrueNorth and Braindrop neuromorphic systems. We will also describe ongoing work on creating a quantitative, full-stack approach to evaluating the trade-offs in neuromorphic system design, enabled by our recent work on open-source tools for the design and implementation of self-timed logic. ![]() Professor Rajit ManoharYale University, USA ![]() Professor Rajit ManoharYale University, USA Rajit Manohar is the John C Malone Professor of Electrical and Computer Engineering and Professor of Computer Science at Yale. He received his BS (1994), MS (1995), and PhD (1998) from Caltech. His group conducts research on the design, analysis, and implementation of self-timed systems. He is the recipient of twelve best paper awards, nine teaching awards, and was named to MIT technology review's top 35 young innovators under 35 for contributions to low power microprocessor design. His work includes the design and implementation of a number of self-timed VLSI chips including the first high-performance asynchronous microprocessor, the first microprocessor for sensor networks, the first asynchronous dataflow FPGA, and the first deterministic large-scale neuromorphic architecture. His group developed the first true ASIC flow for asynchronous circuits. Prior to Yale, He founded Achronix Semiconductor to commercialise high-performance asynchronous FPGAs. |
15:30-16:15 |
AI acceleration roadmap: co-designing algorithms, hardware, and software
Deep Neural Networks (DNNs) have become state-of-the-art in a variety of machine learning tasks spanning domains across vision, speech, and machine translation. Deep Learning (DL) achieves high accuracy in these tasks at the expense of 100s of ExaOps of computation. Hardware specialization and acceleration is a key enabler to improve operational efficiency of DNNs, in turn requiring synergistic cross-layer design across algorithms, hardware, and software. In this talk I will present this holistic approach adopted in the design of a multi-TOPs AI hardware accelerator. Key advances in the AI algorithm/application-level exploiting approximate computing techniques enable deriving low-precision DNNs models that maintain the same level of accuracy. Hardware performance-aware design space exploration is critical during compilation to map DNNs with diverse computational characteristics systematically and optimally while preserving familiar programming and user interfaces. The opportunities to co-optimize the algorithms, hardware, and the software provides the roadmap to continue to deliver superior performance over the next decade. ![]() Dr Viji SrinivasanIBM, USA ![]() Dr Viji SrinivasanIBM, USA Viji Srinivasan is a Distinguished Research Scientist and a manager of the accelerator architectures and compilers group at the IBM TJ Watson Research Center in Yorktown Heights. At IBM, she has worked on various aspects of data management including energy-efficient processor designs, microarchitecture of the memory hierarchies of large-scale servers, cache coherence management of symmetric multiprocessors, accelerators for data analytics applications and more recently end-to-end accelerator solutions for AI. She leads the architecture and compiler team of IBM’s AI Accelerator. Many of her research contributions have been incorporated into IBM’s Power & System-z Enterprise-class servers. |
Chair
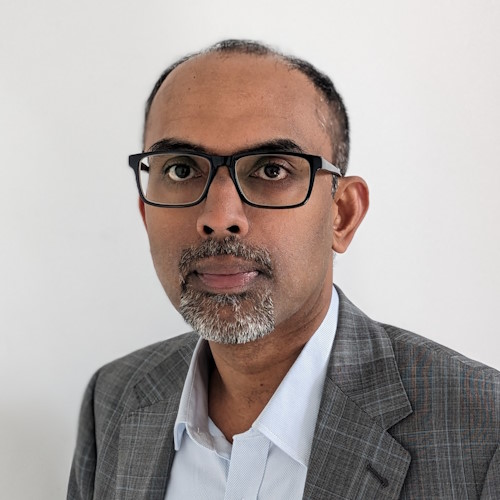
Professor Bipin Rajendran
King's College London, UK
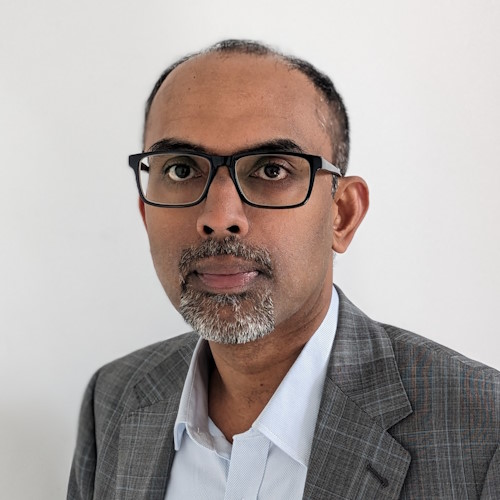
Professor Bipin Rajendran
King's College London, UK
Bipin Rajendran is a Professor of Intelligent Computing Systems and EPSRC Fellow at King’s College London (KCL). He received a B Tech degree from IIT Kharagpur in 2000, and MS and PhD degrees in Electrical Engineering from Stanford University in 2003 and 2006, respectively. He was a Master Inventor and Research Staff Member at IBM TJ Watson Research Centre in New York from 2006-’12 and has held faculty positions in India and the US. His research focuses on building algorithms, devices, and systems for brain-inspired computing. He has co-authored over 100 papers in peer-reviewed journals and conferences, one monograph, one edited book, and 59 issued US patents. He is a recipient of the IBM Faculty Award (2019), IBM Research Division Award (2012), and IBM Technical Accomplishment (2010). He was elected a senior member of the US National Academy of Inventors in 2019.
09:15-10:00 |
Neuromorphic intelligence: from AI-enabled to neuroscience-inspired solutions
The development of efficient bio-inspired algorithms and hardware is currently missing a clear framework. Should we start from the brain computational primitives and figure out how to apply them to real-world problems (bottom-up approach), or should we build on working AI solutions and fine-tune them to increase their biological plausibility (top-down approach)? We will see why biological plausibility and hardware efficiency are often two sides of the same coin, and how neuroscience- and AI-driven insights can cross-feed each other toward neuromorphic edge intelligence. By applying these findings to real-world problems such as on-device learning and safety-critical scenarios, we will show how smart devices can be made to adapt to their environment and users within a few microwatts, or how they can be made to react to stimulus within just a few microseconds. ![]() Dr Charlotte FrenkelDelft University of Technology, The Netherlands ![]() Dr Charlotte FrenkelDelft University of Technology, The Netherlands Charlotte Frenkel is an Assistant Professor at Delft University of Technology, The Netherlands. She received her PhD from Université catholique de Louvain in 2020 and was a post-doctoral researcher at the Institute of Neuroinformatics, UZH, and ETH Zürich, Switzerland. Her research aims at bridging the bottom-up (bio-inspired) and top-down (engineering-driven) design approaches toward neuromorphic intelligence, with a focus on digital neuromorphic processor design, embedded machine learning, and brain-inspired on-device learning. Dr Frenkel received a best paper award at IEEE ISCAS 2020, and her PhD thesis was awarded the FNRS / Nokia Bell Scientific Award 2021 and the FNRS / IBM Innovation Award 2021. She serves or has served as a program co-chair of the NICE neuromorphic conference and of the tinyML Research Symposium, as a co-lead of NeuroBench, as a TPC member of IEEE ESSERC, and as an associate editor for IEEE TBioCAS. |
---|---|
10:00-10:15 |
Break
|
10:15-11:00 |
Automated design space exploration and generation of AI accelerators
Designing high performance and energy efficient AI accelerators requires significant engineering effort, and as the rapidly evolving field of machine learning develops new models, the current approach of designing ad hoc accelerators does not scale. In this talk, Professor Raina will present our ongoing research on a high-level synthesis (HLS)-based framework for design space exploration and generation of hardware accelerators for AI. Given architectural parameters, such as datatype, scaling granularity, compute parallelism and memory sizes, the framework generates a performant fabrication-ready accelerator. Accelerators generated through this framework have been taped out in several chips, targeting various workloads including convolutional neural networks and transformer networks. In this talk, Professor Raina will present both the generator framework as well as some of the chips she and her team have designed using it. ![]() Professor Priyanka RainaStanford University, USA ![]() Professor Priyanka RainaStanford University, USA Priyanka Raina received the BTech degree in Electrical Engineering from IIT Delhi in 2011, and the MS and PhD degrees in Electrical Engineering and Computer Science from MIT in 2013 and 2018, respectively. She was a Visiting Research Scientist with NVIDIA Corporation in 2018. Since 2018 she is an Assistant Professor of Electrical Engineering at Stanford University, where she works on domain-specific hardware architectures and agile hardware–software codesign methodology. Dr Raina is a 2018 Terman Faculty Fellow. She has won the DARPA Young Faculty Award in 2024, Sloan Research Fellowship in 2024, the National Science Foundation (NSF) CAREER Award in 2023, the Intel Rising Star Faculty Award in 2021, and the Hellman Faculty Scholar Award in 2019. She was the Program Chair of the IEEE Hot Chips in 2020. She serves as an Associate Editor for the IEEE Journal of Solid-State Circuits and IEEE Solid-State Circuits Letters. |
11:15-12:00 |
Photonics for next-generation compute - opportunities and challenges
Optical compute has a long history, with very little commercial success. "This time is different" does not seem to cut it with most sceptics. In this talk, Professor Farmakidis will try to convince you that it is not optics that is the secret ingredient, but the unique confluence of opportunities afforded by integration that photonics now provides (and did not during the earlier wave of optical computing). Crucially, such systems allow us to integrate electronics and optics together on a platform that has provided the impetus aided by the surge in demand for GPU/accelerator-type compute. Professor Farmakidis shall provide an overview of the research of his former and current colleagues in this area to create a scaffold for discussion. ![]() Professor Nikolaos FarmakidisUniversity of Oxford, UK ![]() Professor Nikolaos FarmakidisUniversity of Oxford, UK Nikolaos Farmakidis is a departmental lecturer in advanced nanoscale engineering at the University of Oxford. He received his DPhil degree in materials from the University of Oxford and has been working primarily on integrated photonic technologies for computing and sensing since then. His research interests lie at the intersection of photonics, electronics, and materials, with a focus on application-driven innovation. Nick is recognised for several impactful patents and publications in the fields of neuromorphic computing, plasmonics, and sustainable photonic computing. Before joining Oxford, he held research and teaching positions at Columbia University working on lab-on-a-chip technologies, and Boston University working on additive nanomanuacturing and nanometrology. |
Chair
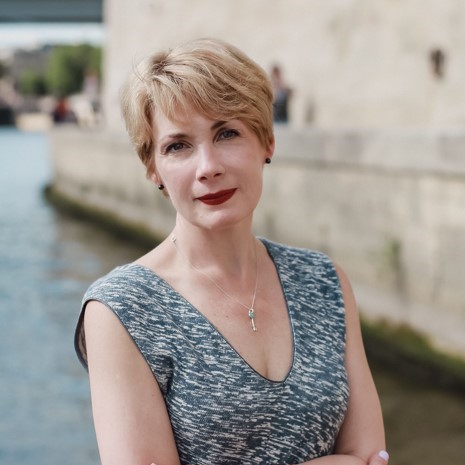
Dr Olga Kazakova
National Physical Laboratory, UK
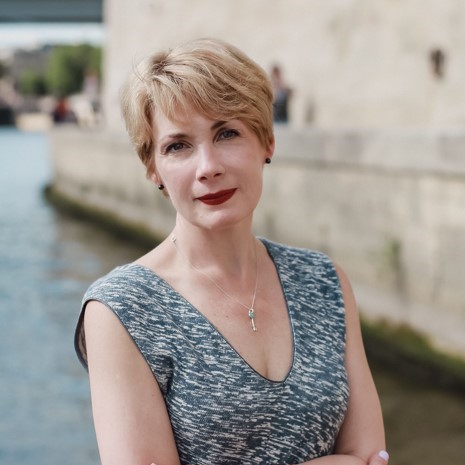
Dr Olga Kazakova
National Physical Laboratory, UK
Olga Kazakova is an NPL Fellow in Quantum Materials and Sensors. She joined NPL in 2002 after working as an Assistant Professor at the Chalmers University of Technology (Gothenburg, Sweden). Olga is a Fellow of the Institute of Physics and a visiting professor at University of Manchester. She is an author of above 190 peer-refereed publications and has delivered over 70 invited talks and seminars. Olga is actively involved in the Materials for Quantum Network (M4QN), being a Chair of the Spin & Topology Material Interest Group and an Executive Committee representative. She is also a member of several industrial advisory boards in the UK. Olga has been actively involved if the IEEE Magnetic Society, in past serving as an Associate Chair of the Conference Executive Committee and being a member of the Administrative Board. She has been a recipient of the numerous national and international awards, including Intel European Research and Innovation Award (2008), NPL Rayleigh Award and Serco Global Pulse Award (2011). She has a vast editorial and conference organisation experience and has a broad collaborative network. Olga has profound research experience in quantum materials, nanotechnology, low loss electronics, and the development of novel sensors. She has a strong experience in project and team management, supervision and coaching.
13:30-14:15 |
Quantum enhanced generative AI with ORCA’s PT series
At ORCA Computing we believe that quantum mechanics provides a revolutionary new paradigm for the most demanding computing applications. But unlike other companies in the quantum computing space, we have shown that our current and near-term quantum systems can be leveraged to enhance the performance of machine learning and large scale AI models. In this talk, Josh Nunn will describe the promise of photonic quantum processors, and he will explain the principles and technologies underlying their approach. ![]() Dr Josh NunnORCA Computing Ltd, UK ![]() Dr Josh NunnORCA Computing Ltd, UK Josh is co-founder and Chief Scientist of ORCA Computing, a full stack quantum computing company focussing on photonic quantum systems for machine learning and generative AI. Josh also co-founded the quantum networking start-up Veriqloud in Paris. Previously, he was a Reader in Photonics at the Department of Physics at the University of Bath, where his research focused on quantum light-matter interactions. Prior to this, he was a Royal Society University Research Fellow at the University of Oxford, running the Photonics programme for the UK National Quantum Technology Hub in Networked Quantum Information Technologies (NQIT) where his team invented the ORCA memory. He has published papers on Raman storage, quantum state and process tomography, quantum thermodynamics and quantum key distribution, and has patents licensed on microwave-to-optical conversion and random number generation. |
---|---|
14:15-14:30 |
Break
|
14:30-15:15 |
Neutral atoms: a flexible platform for quantum AI
Arrays of optically trapped neutral atoms have long been neglected as a viable platform for Quantum Computers, only in recent years subverting this belief by demonstrating the possibility to run both analogue protocols native to this architecture, as well as more mainstream digital quantum algorithms, recently culminating in convincing demonstrations of error correction protocols. In this talk Dr Gentile will present the fundamental features and advantages of neutral atom QPUs, the various modes of operation, discuss their state-of-art limitations and (Pasqal’s) roadmap for their near future, and highlight their potential by showcasing some key perspective use cases in various types of physics and engineering problems, towards industrial-scale relevant applications. In particular, he will focus on how Pasqal investigated porting into quantum protocols: (i) graph-based approaches to Machine Learning, that attained increasing attention in the ML community, and can best leverage the analogue mode of neutral atom machines; (ii) Scientific Machine Learning (SciML) approaches, targeting the solution of (stochastic) Differential equations, seamlessly embed actual data where available, and exploiting generalisation properties typical of ML models; (iii) extremisation & combinatorial optimisation, a traditional target of quantum methodologies that we address via appropriate embeddings to reduce such optimisations to either Quantum-SciML or graph theoretic strategies. ![]() Dr Antonio "Andrea" GentilePasqal SaS, The Netherlands ![]() Dr Antonio "Andrea" GentilePasqal SaS, The Netherlands Antonio "Andrea" Gentile leads a software unit in the quantum scale-up Pasqal, working at the intersection between quantum computing and machine learning and actively involved in various R&D collaborations with leading academic institutions around the world. Involved early-on in quantum technologies, his current interests revolve around investigating the potential applications of quantum computers and simulators. Now and then he dives out of the above and into the Ocean(s). His academic background is mostly in Physics, and spans a diverse range of interests, moving from (experimental) Nanotechnology to Condensed Matter Physics, with a PhD from the Quantum Technology Labs (UniBristol, UK), focusing on integrated photonic implementations of quantum simulators. As side interests, Andrea previously co-founded a high-tech venture (“Material Recovery Systems”) active in industrial waste recovery from thin-films manufacturing, gained project management and business strategy skills as a Fulbright BEST scholar in the USA. |
15:30-16:15 |
The Quantum Internet: wiring the weirdness
The interconnection of quantum devices via the Quantum Internet - ie through a network enabling quantum communications among remote quantum nodes – is a disruptive technology. Indeed, the Quantum Internet can provide functionalities with no counterpart in the classical world, such as advanced quantum security services, distributed quantum computing services characterised by exponential increase of the computing power, and new forms of communications. These functionalities will fundamentally change our lives in ways we cannot fully image yet. The aim of the talk is to provide the participants with a wide view about quantum communications by highlighting the challenges and the opportunities connected to the design of the Quantum Internet, which requires a major network-paradigm shift and a multidisciplinary effort to harness the counter-intuitive marvels of quantum mechanics. ![]() Professor Angela Sara CacciapuotiUniversity of Naples Federico II, Italy ![]() Professor Angela Sara CacciapuotiUniversity of Naples Federico II, Italy Angela Sara Cacciapuoti is a Professor of Quantum Communications and Networks at the University of Naples Federico II (Italy). She is an 2024 ERC grantee for the Grant “QNattyNet”. Her work has appeared in first tier IEEE journals and she received different awards, including the “2024 IEEE ComSoc Award for Advances in Communication”, the “2022 IEEE ComSoc Best Tutorial Paper Award”, the “2022 WICE Outstanding Achievement Award” for her contributions in the quantum communication and network fields, and “2021 N2Women: Stars in Networking and Communications”. She also received the IEEE ComSoc Distinguished Service Award for EMEA 2023, assigned for the outstanding service to IEEE ComSoc in the EMEA Region. Currently, she is an IEEE ComSoc Distinguished Lecturer with lecture topics on the Quantum Internet design. And she serves also as Member of the TC on SPCOM within the IEEE Signal Processing Society. Moreover, she serves as Area Editor for IEEE Trans on Communications and as Editor/Associate Editor for the journals: npj Quantum Information, IEEE Trans on Quantum Engineering and IEEE Communications Surveys & Tutorials. She is also Associate EiC for IEEE ComSoc Best Readings. Her research interests are in Quantum Information Processing, Quantum Communications and Quantum Networks. |
16:15-17:00 |
Panel on "Bits, neurons, and qubits for sustainable AI: the way forward"
![]() Sir Bashir M Al-Hashimi CBE FREng FRSKing's College London, UK ![]() Sir Bashir M Al-Hashimi CBE FREng FRSKing's College London, UK Bashir M Al-Hashimi is recognised for sustained, pioneering contributions to advanced semiconductor chips test, energy-efficient embedded systems and energy harvesting computing. As Vice President (Research & Innovation) and Arm Professor of Computer Engineering at King's College London, his research has led to substantive innovations and impact worldwide through related enabling hardware and software technologies applied in mobile electronic systems and devices. With over 350 technical papers and 8 best paper awards at international conferences, he has also authored / co-authored and edited 5 books. Influential and visible in Higher Education and experienced in UK research assessments and government consultations, he has received £25m in external research funding and several international awards including the IET Faraday Medal (2020). His contributions to engineering and industry were recognised by a CBE in the Queen’s Honours 2018 and election as Fellow of the Royal Academy of Engineering in 2013. Elected Fellow of the Royal Society in 2023, he is a member of Sectional Committee 4 (Engineering). |