Following the publication of the latest Philosophical Transactions A theme issue, ‘Reliability and reproducibility in computational science: implementing verification, validation and uncertainty quantification in silico’, Dr Roger Highfield, Science Director of the Science Museum Group and contributing author to the issue, explores the question, ‘should we trust computers?’.
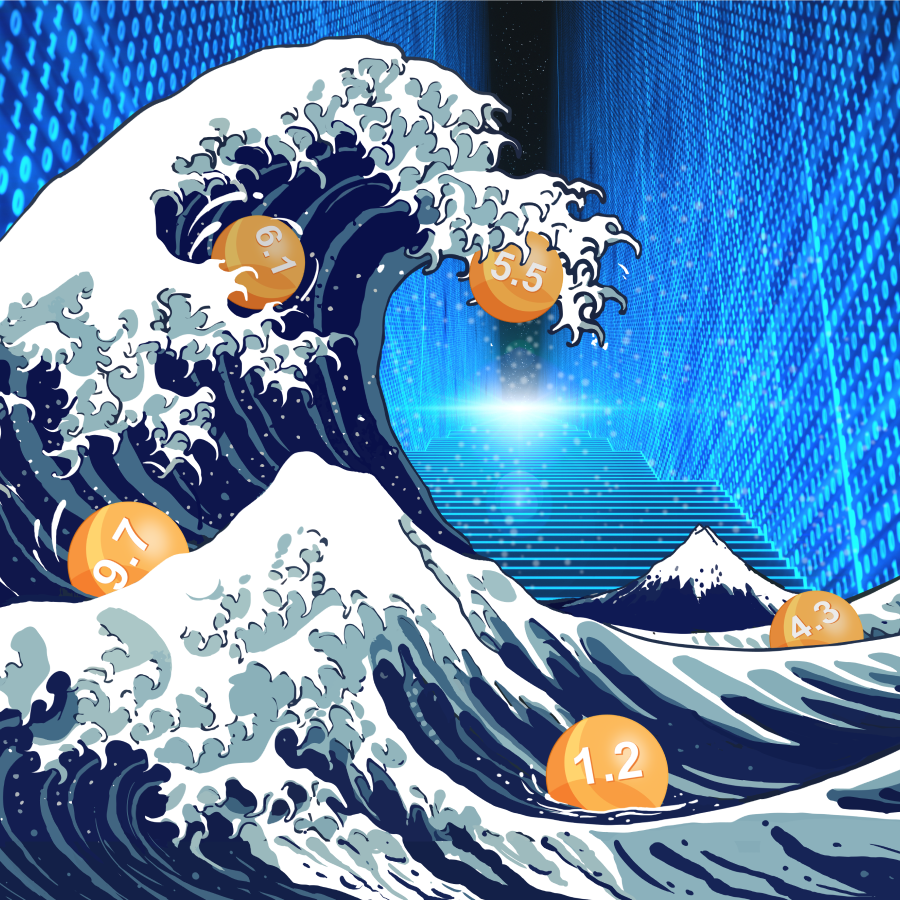
Science harbours an embarrassing secret: it is done by people, with all their frailties, foibles, and shortcomings. Yes, they do have many noble qualities, but they can sometimes make mistakes. They also have a capacity for self-deception, sometimes even fraud. As computers become ever more powerful, can these machines make science less reliant on people? Can we harness them to make science more objective? Can we use AI to erase subjectivity from research? The answer is a heavily-qualified yes, according to this Philosophical Transactions A special issue.
Computers have shortcomings too, not least that they are only as smart as the people who use them, the people who write their algorithms, the people who supply their data and the people who curate those data and algorithms based on the current state of understanding, in the form of theory. The implication of the papers in this special issue is that we need to broaden the Royal Society’s motto, nullius in verba. As well as taking ‘nobody’s word for it’, we need to extend that healthy skepticism to in silico science: take no machine’s word for it either. Peter Coveney, who edited the issue with Derek Groen and Alfons Hoekstra, argues that computational science now needs the rigorous application of verification and validation, along with uncertainty quantification, collectively known by the acronym, VVUQ. The advantage is that, when computer-based predictions pass muster, in terms of VVUQ, they become “actionable” – you can use them to make decisions. If we can reliably predict events before they occur using a computer, we can use them to great effect, from weather forecasting to clinical decision-making. With the relentless rise of computer applications in research, reproducibility in computational science is becoming increasingly important; from the use of ‘digital twins’ to carry out virtual tests across engineering, to computer models to simulate shape public health policy, for drug design, climate modelling, diagnosis of disease, the virtual human project and more.
While computers are surging in power, concerns about reproducibility abound, notably in the biosciences. In 2005, John P. A. Ioannidis wrote an influential article about biomedical research with the title “Why Most Published Research Findings are False”. There are many documented examples of reproducibility issues in medical science, along with psychological science and cognitive neuroscience as well. Though the worst fears around the ‘reproducibility crisis’ are likely exaggerated, the consequences can be profound. As one example, the infamous MMR studies by Andrew Wakefield have paved the way for a surge in antivaccination views, which are predicted to rise, according to one recent analysis of the views of nearly 100 million people on Facebook.
Can computers help tackle the reproducibility crisis? They can, so long as it is appreciated that they are constrained; biomedical and life sciences do not submit easily to mathematical treatment because they deal with such complex systems. Compared with the physical sciences, they are relatively lacking in theory. One way around this is to use big data and machine learning, but there can be too much data to produce correlations to any degree of confidence, while the ratio of false to true correlations soars with the size of the dataset. Assumptions are often made about complex systems, such as the smoothness of the curves that join data points (not always true) or that they fall on a bell curve or, perhaps best known and most infamous of all, that correlation implies causality. Without deeper theoretical understanding, AI methods can be “black boxes” that reveal little about their inherent limitations.
To deal with the challenges of reproducibility, countermeasures have been suggested: documenting detailed methodological and statistical plans of an experiment ahead of data collection; demanding that studies are thoroughly replicated before they are published; insisting on collaborations to double check findings; explicit consideration of alternative hypotheses; and the sharing of methods. That should extend to the sharing of data, computer code and results in central repositories too. Along with transparency and openness, computational science needs rigorous VVUQ.
The need for VVUQ and reproducibility is critical for a future where computer models will be used to make predictions about the state of the planet, or the rise of a deadly disease, or indeed any forecast which could lead to a drastic change in the way we live. We need to be more confident than ever that we can trust computers because, as climate change and the COVID-19 pandemic have shown, that future is already upon us. However, there is a deeper issue when it comes to our reliance on digital computers. Ever since the 1960s, when Edward Lorenz discovered that tiny rounding errors can lead to chaotic fluctuations (the ‘butterfly effect’), we have known that care must be taken with systems that have strong sensitivity to rounding or inaccuracies. Working with colleagues in the United States, Peter Coveney has shown that this sensitivity can be a problem for digital computers.
The impact on simulation science is still under investigation. However, as I point out in the special issue with Peter Coveney, and in a blog for the Science Museum, the corollary of this work is that renewed emphasis on analogue computers will be necessary in the long term, not just because of the rounding issue but because of the soaring power demands of next generation exascale computers, where many tens of megawatts are needed to power the hot heart of a single high-performance machine. We may need a revival of the oldest form of computation to cool the excessive faith currently placed in digital computation.
Pace TR-48 Analogue Computer, by Electronic Associates Inc (EAI), United States, 1960-1965. Designed to work on a 'desk top', this model was used by Brunel University, London, until 1989. (Image credit: Dr MBE Abdelrazik)
For more information about Philosophical Transactions A, and for details about how to become a Guest Editor, please visit our website.