In a Journal of the Royal Society Interface paper, artificial intelligence methods are used to analyse fossil tracks. The work sheds light on why this approach outperforms conventional methods.
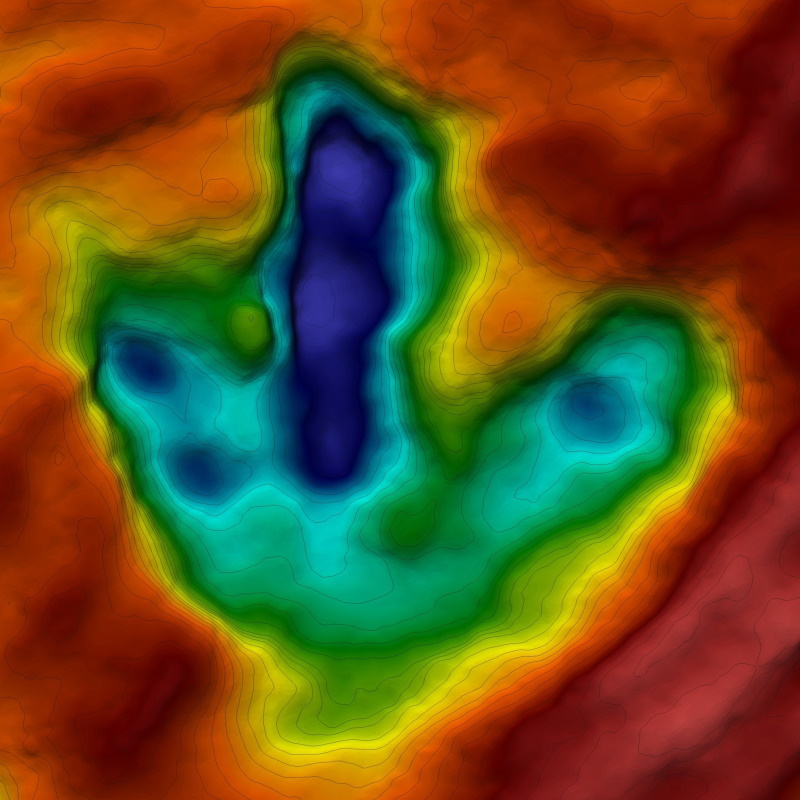
A recent Journal of the Royal Society Interface paper examines how deep convolutional neural networks, a form of artificial intelligence, can help researchers analyse dinosaur tracks. We spoke to one of the authors, Dr Jens Lallensack, to find out more.
Please can you introduce your research and tell us what it’s about?
We tested if artificial intelligence, more specifically deep convolutional neural networks, can solve longstanding problems in the analysis of fossil tracks. We focused on a particularly difficult problem: the discrimination between three-toed tracks from the plant-eating ornithischians from those of the meat-eating theropods. On an independent test set, our neural network outperformed human experts by a wide margin. We then used our neural network to classify some controversial cases, including the trackway of a large dinosaur in Queensland, Australia, that is thought to have triggered a stampede of smaller dinosaurs. It is the first application of artificial intelligence methods to fossil tracks.
How does deep convolutional neural network analysis work?
Such artificial neural networks are inspired by the structure of the human brain. They learn from a larger number of examples – in our case, 1372 track outlines of known category – either "theropod" or "ornithischian". Based on these training examples, the neural network will attempt to determine features, or combinations of features, that are helpful for distinguishing the categories. It therefore figures out everything by itself, and could possibly find ways to distinguish these categories that humans haven't thought about yet.
Why does this approach outperform more traditional methods of fossil track analysis?
It is a much more flexible approach. Traditional methods are based on linear measurements (e.g., track length), angular measurements (e.g., angles between toes), or landmarks (e.g., coordinates of the toe tips). However, these measurements can only capture parts of the complex shape information. More importantly, measurements need to be consistent in all tracks that are to be analysed. However, the shape of a track may change drastically from one step to the next, and different species may show different features in their tracks. A neural network is able to model all of this complexity. Given that traditional methods had such limitations, the study of tracks remained a largely qualitative discipline, relying in large parts on human intuition. We now show that neural networks may outperform such human intuition.
What do you think the future potential of this approach is for palaeontology?
We believe that deep convolutional neural networks are the most promising technique that may significantly advance our field in the near future. A track is the result of multiple factors, including the anatomy of the foot, the consistency of the substrate, the movements of the animal that made the track, and alteration of the track that occurred after it was formed. This makes interpreting fossil tracks so difficult. To go further, we need powerful quantitative methods rather than human intuition, and neural networks may be a real game changer.
For more research that applies innovative techniques to complex questions, check out Journal of the Royal Society Interface.
Image caption: Theropod (meat-eating) dinosaur track from Münchehagen, Germany. In this false-colour height map, red indicates high regions and blue low regions. Image credit: Jens N. Lallensack