The growth of data science and its importance to society creates an opportunity to establish it as a profession with public confidence and trust.
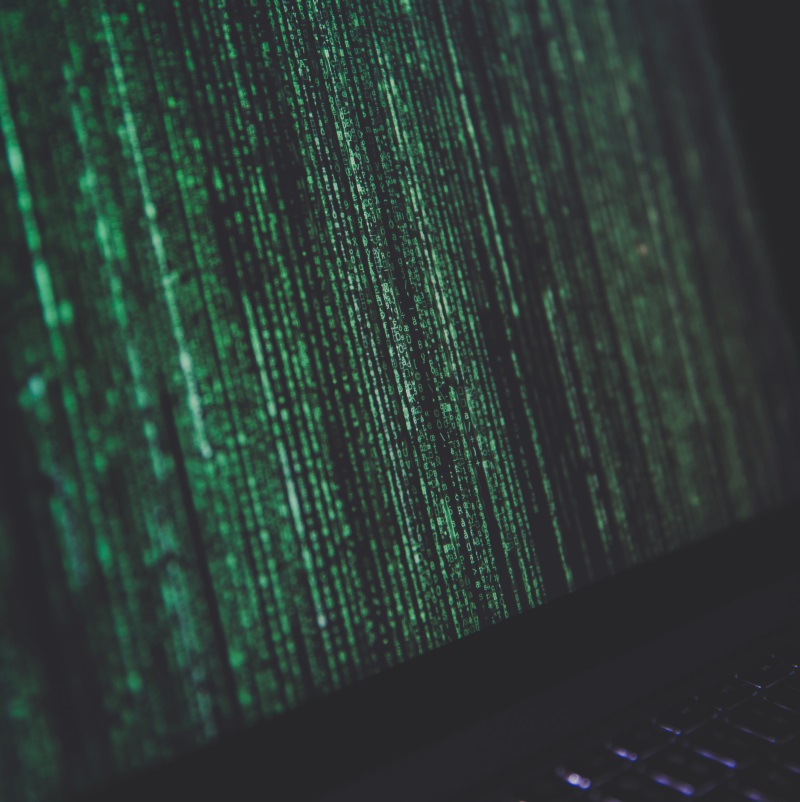
The COVID-19 pandemic has highlighted how data can be critical to life and to livelihoods. As the pandemic has unfolded, data of many kinds has proven crucial, be that testing data, healthcare data or travel data, often processed by epidemiological models to inform government decisions on interventions to manage the pandemic. Given the potential of data to impact on individuals, communities and society, it is important to know: how reliable is the data and its collection and processing? And how rigorous or fair are the processes for capturing, analysing and interpreting this data? Questions about data use and analysis aren’t new, but what makes them particularly timely now is the growing importance of the field of “data science” – a powerful interdisciplinary practice of mathematics, statistics, and computer science that draws on unprecedentedly large or complex datasets. The rapid growth of data science and its importance to society creates an opportunity to establish it now as a profession with public confidence and trust.
Data collection: planning for unforeseeable situations
Consider an apparently straightforward example of data collection: the recording of deaths. In Britain, there is a legal requirement to register a death. Medical registrars, in turn, are obliged to report (PDF) the results promptly so that the Office for National Statistics can publish weekly totals for deaths with some high-level analysis of the figures. But when the COVID-19 pandemic broke out, weekly reporting wasn’t sufficient given the speed of infections, and it became vital to get accurate data in real time in order to understand how the pandemic was developing. This posed a challenge, as while hospitals could report on COVID-19 related deaths in hospital, there was no obvious way to tally this record with registered deaths, nor to allow for NHS Health Trusts each compiling their respective figures at different speeds.
Data science is central to creating systems for approaching challenges like these. If creating a data recording system for deaths, a professional data science approach tries far as possible to foresee the range of demands that might be made of the data, so that the form of its capture would make it fit for all these different purposes in the future. For example, it might ensure that the data is traceable, so that errors can be tracked to source and corrected. At the same time, it might ensure that the collection and storage of the data is done ethically and does not compromise the right to privacy of the deceased and their families and friends. And it would do all this while keeping the effort proportionate to the benefit that might be expected to accrue from it, to minimise administrative demands on busy health and care professionals. Introducing professional development frameworks for data scientists doesn’t guarantee perfect solutions every time, but it might ensure that a data scientist or team of data scientists tasked with a technical challenge had tools for being aware of a wide range of considerations.
Data interpretation: handling uncertainty
Data always comes with uncertainty regarding its accuracy and its completeness. Misunderstanding or underestimating the limitations of data can invalidate the conclusions subsequently drawn from it, potentially giving rise to unintended consequences and in the worst cases life-changing implications. An example of this importance of data assurance is the recent Surgisphere controversy over hydroxychloroquine studies, whereby the World Health Organisation and some national governments based COVID-19 policy and treatment decisions on a data source that was subsequently challenged. It is essential that any conclusions made from data analysis can be interpreted and contextualised by a range of data practitioners with a view to possible risks around the data uncertainties. A professional data science approach could facilitate this by providing a competence framework that included essential technical skills such as data curation – identifying the strengths and limitations of the datasets in use – and applied or “soft” skills such as communication of data uncertainty (PDF) to laypeople who will use the outcomes of the data analysis.
Data analysis and the human in the loop
At the other end of the spectrum to data collection, very advanced modelling or predictive analytics are being employed to support complex decision-making on the data that has been captured, often drawing on machine learning or techniques such as Artificial Intelligence (AI). But the contribution of human interpretation and judgement alongside sophisticated computational analysis is essential: for example, do the models treat different population groups equally, are the decisions fair, are the decisions consistent, does a decision remain valid if the circumstances change? An openSAFELY study published in Nature found that 26% of the patient records in their analysis didn’t contain ethnicity data; but, since ethnicity is now a known risk factor for COVID-19, this might present challenges for the reliability of a risk scoring algorithm trained on the data. Additionally, recent research by the Health Foundation has highlighted the importance of an intersectional approach to health inequalities in COVID-19 that considers combinations that might accentuate risk for individuals or groups, instead of a universal public health approach. By standardising professional approaches for data science, these can help ensure ethical practice and codes of conduct are in place to help guide data science practitioners regardless of their technical or personal strengths, backgrounds, or experiences so far.
A roadmap for the future
In June 2019, the Royal Society published its Dynamics of Data Science Skills report, assessing the UK’s data science landscape. One of its recommendations was that data science be developed as a profession – that is to say, bringing together accredited training, standards, frameworks and codes of conduct to ensure that data scientists have the skills, knowledge and behaviours to navigate the challenges of the data science environment. Since then, a group of professional bodies led by the British Computer Society, the Operational Research Society and the Royal Statistical Society, in partnership with the Royal Society and the Royal Academy of Engineering, has been exploring what a coordinated approach to the professionalisation of data science might look like.
This is important because the UK is positioned to be a world leader in data science, and data science has a crucial role to play in the digital economy – across all aspects of business and policy, and across society. This is a trend that is set to grow, as we see advances in the volume and variety of data collected and analysed, advances in algorithms used for data analysis, and developments in new technologies derived from these advanced uses of data. Alongside this, the need to build and maintain trust and confidence in data science will increase too. Professionalisation of data science will play a vital part in this and is a key building block for the UK to grow the most trusted, ethical and sought-after data science teams in the world.
You can learn more about the roadmap for professionalising data science in the UK and contact the project team through this webform.
You can also download the Royal Society’s report Dynamics of Data Science Skills (PDF).